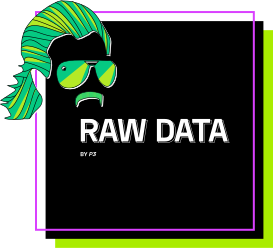
Raw Data By P3 Adaptive
“Hallucination” is Just a Fancy Word for Being Wrong
Listen Now:Dive into the depths of AI with Rob and Justin in our latest episode where we peel back the layers of AI’s current facade. In this compelling discussion, we take a critical look at the hyped-up narratives surrounding artificial intelligence, focusing on a crucial issue at its core: the “hallucination problem.” Rob and Justin navigate through the intriguing landscape of AI advancements, debunking myths and uncovering the reality behind AI’s ability to produce outputs that, while often impressive, can lead to misleading and incorrect conclusions. This episode promises to enlighten you with a fresh perspective on AI, challenging the status quo and urging listeners to question what lies beyond the surface of this technology’s glittering promise.
But it’s not all critique and caution; our hosts also delve into the implications of AI’s limitations for businesses and technologists alike, fostering a dialogue on how we can navigate this evolving landscape with both optimism and pragmatism. “Hallucination” is a Fancy Word for Being Wrong is more than just a critique; it’s a journey to understanding the nuances of AI, encouraging listeners to embrace the complexity of this revolutionary technology.
And as always, if you enjoyed this episode be sure to leave us a review on your favorite podcast platform to help new listeners find us. Also, click subscribe for new content delivered weekly!
Also in this episode:
Rob Collie (00:00): Hello, friends. When we select topics for this show at a really high level, it maybe is in a gross oversimplification, we can choose to talk about one of three different things. We can talk about Power BI, we can talk about Fabric, and we can talk about AI. And I'm sure you're not going to find this the least bit surprising, but we have dashboards. We know which of these three topics is most popular. The popularity champion out of those three topics remains Power BI.
(00:29): Now, it's not like a runaway popularity edge. When I say it's most popular, one of our recent Power BI episodes outperformed some of our more recent AI and Fabric episodes by about 20%. So the gap isn't massive, but it is significant. And if we were strictly a follow the numbers kind of podcast, which you might suspect for a moment that we would be, we are a data firm after all.
(00:51): I'm here to surprise you with the news that today's episode is about AI. So if we know that the Power BI episodes are more popular, why would we insist on continuing to talk about AI? Well, there's a good reason for that and basically it's this, any responsible practitioner or business leader in the data space needs to be thinking about AI. Whether you want to stay safely cocooned in the Power BI world, which still has tremendous, tremendous runway left in it in terms of the impact it will provide. Completely agree with you. The fact remains that you are going to be asked about AI, and you're going to be asked about it in a world in which there's an expectation that AI is magic.
(01:36): Now, there are absolutely use cases for AI that are super, super, super useful, and being able to spot those provides a lot of business value. But that baseline background expectation that it's going to be magic puts a lot of undue pressure on the expectations and also the breadth of where you engage with it. So if data is part of your professional identity whether as a business leader or as a practitioner, you like us, you need to be developing mental frameworks that help you navigate this space.
(02:09): And a recent series of behind the scenes conversations that I've been having with Justin have led me to some very clarifying epiphanies that are absolutely now helping me make increasing sense of where the really tangible and direct world of things like Power BI and this hazier more background magical expectations world of AI where those two worlds converge. And as that clarifies for me, it's already making me more effective. For example, I recently had a chance encounter with a CFO out in the real world, and we were talking about AI and his organization's long-term ambitions for AI.
(02:50): And this organization was not a P3 Adaptive client at the time, but that conversation about AI, if you fast forward a little bit, leads to us P3, launching a Power BI project with his organization this week. Being able to connect the dots between the tangible world of things like Power BI and that future ambition world of AI is a very, very, very useful professional skill. And since we kind of have an informal policy here at the Raw Data Podcast of me epiphany is sue epiphany.
(03:23): Today, we're going to talk about this thing called the hallucination problem and how it's very often mentioned kind of as a detail or as an afterthought in the AI space, and it turns out it's kind of everything. It is the most important problem. And being able to spot application scenarios in which the hallucination problem is less of a problem is one of the key frameworks you need to develop.
(03:48): So the dashboard numbers be damned. We have a responsibility to you, dear listener. We're going to talk about AI and we're going to keep doing it. Of course. We're also going to talk about the other things, and I just told you talking about AI led to more Power BI. Even if you want to hide in your cocoon. Talking about AI gets more people in that cocoon with you. What could be better? You know what would be better? What is it? If we got into it. Yeah, let's do that.
Announcer (04:17): Ladies and gentlemen, may I have your attention please?
(04:21): This is the Raw Data by P3 Adaptive Podcast, with your host Rob Collie and your co-host, Justin Mannhardt. Find out what the experts at P3 Adaptive can do for your business. Just go to p3adaptive.com. Raw Data by P3 Adaptive is data with the human element.
Rob Collie (04:47): Well, hello there, Justin. Do I detect the slightest hint of a tan? You had an offsite down in Florida. I don't actually detect tan. It looks like you didn't get outside at all.
Justin Mannhardt (04:55): We did have an offsite. It was really great, but we spent most of the time inside of the meeting room and managed to walk out to the beach, I think two times. Not enough for the tan to set in, but it was very nice.
Rob Collie (05:07): But it was good to be ocean side, I bet.
Justin Mannhardt (05:10): Yes. We could see the waters out the window, which ultimately became a distraction because the light behind the people sitting across the table from me, you eventually couldn't make out their facial expression or detail anymore. So I had to close the blinds to the beautiful ocean waters.
Rob Collie (05:28): Oh, it's terrible. That reminds me of many years ago when I was a youngster at Microsoft and I was way in over my head and I was much more anxious of a human being than I am today. I was at this offsite where ultimately, I had to present later in the day, and this was an offsite that I really didn't have any business being at, especially not being a speaker at. It was like this big referendum on the future architectural approach at Microsoft. It was just full of all these monster brains, high profile like celebrities within Microsoft.
(06:04): I was only there because I was the person who knew the most about 32-bit Windows application installation. It was the least interesting, least sexy thing to be the world's leading expert in.
Justin Mannhardt (06:18): Probably goes something like, "Hey, we need someone to talk about this. Oh, it's Rob? All right. Okay, fine, Rob."
Rob Collie (06:25): At Microsoft, this would rank near the bottom one of the absolute shittiest jobs. But man, I was good at it. And so now suddenly I'm there to give a talk about it where people are going to tear me apart. That was what I was afraid of anyway. I'm sitting here at this lakeside banquet room at this lakeside resort and it's like 10:00 in the morning and I'm watching these people on the pier coming out of their sailboats and stretching like, whoa, waking up drinking their coffee. They're living on a sailboat and they're hosing it down and cleaning off their sailboat.
(07:00): I'm sitting there in this glass cage waiting for what felt like my execution later that day. I'm watching these people living just a hundred feet away from me. These people that are living this incredibly relaxed and chill Zen-like lifestyle, and I'm dying. It seems so unfair. The punchline of that one was that I don't think in the end they even left me much time to talk. So they tortured me all day with anticipation and then ran out of time.
(07:31): I mean, I talked for a little bit. People were dismissive. Anyway, it just a tremendous stressful trip for no reason. Hey, that's a good segue. So I'm not nervous about presenting anymore. And I gave a talk here last week in Indie and I thought we'd talk about it. First of all, our podcast statistics, we formerly were pulling from our podcast subscription provider via robotic process automation. We had these RPA scripts that logged into the podcast site and clicked all the download buttons to download all of the CSVs.
Justin Mannhardt (08:06): Love me, a good headless machine. Good bot.
Rob Collie (08:09): And that was awesome until inevitably they re-architected their website. And there's something about writing that RPA scripts the first time was fun. Rewriting them just to get exactly the same result was not fun.
Justin Mannhardt (08:22): Not as fun.
Rob Collie (08:23): And I couldn't really sell that internally. But Kellen was excited about writing the API scripts. I didn't even know that Libsyn, our syndicator had APIs. They're not very well advertised on their site, but now we are re-instrumented. Based on that, I can tell you something very interesting.
Justin Mannhardt (08:40): Is this in Power BI? Is this live?
Rob Collie (08:42): Yeah. It's back and live in Power BI, man. Not publicly, but you can get to it. Of our recent episodes, our best performing most recent episode had nothing to do with AI. It was the episode on are you getting the most out of Power BI? This was really good feedback for us because the media, social or otherwise, the media is saturated with AI.
Justin Mannhardt (09:07): It's everywhere.
Rob Collie (09:08): As podcast hosts and also as business leaders, we feel a certain pressure and obligation to talk about AI and especially as our self-appointed keepers of what's actually happening separating hype from reality. And so guess what? My talk last week was in that vein. And so yes, this episode is ostensibly about AI. So if we were following the numbers, we would be talking about Power BI again today. Separate the hype from the reality. The numbers don't lie. The Power BI episode is the champ.
Justin Mannhardt (09:41): You got column charts to prove it.
Rob Collie (09:43): Well, I mean not just column charts, Justin, I have these logarithmic looking shifted by days live. You can't compare episodes that are released in different weeks because they have more time to accumulate downloads. But if you plot them all, you shift it by days live, now you get these line graphs. I think SQLBI recently did a post about video views or something like that.
Justin Mannhardt (10:09): Oh, sure.
Rob Collie (10:09): And the shape of their chart is exactly the chart that we've been using, but we've been using it for years here, Justin.
Justin Mannhardt (10:16): We were first, let the records show.
Rob Collie (10:18): Yeah. So the good news here is that we agree the end of my presentation last week was to advise people whether they're deep into AI or AI obsessed or not to be getting their Power BI house in order. A, for the benefits that it provides. And B, because it's the number one thing you can do in the Microsoft ecosystem to be prepping for AI.
(10:43): And so I started and ended my presentation last week with the same theme. There are two big disruptive waves of innovation, positively disruptive at least in one case. And so we've been living the Power BI power platform wave for about a decade. Now this AI wave is running up behind us and hasn't really given us time. The market hasn't remotely finished getting the value out of that first wave. And so this new wave running up behind it is getting all of the noise and all of the attention, and I think that's a shame.
Justin Mannhardt (11:16): I would agree.
Rob Collie (11:16): Right now it sounds like wave two is the big one. And in some ways it is, but mostly because of it's uncertainty. Whereas wave one, the Power BI wave, I mean, that is still absolutely the place where the ROI is just guaranteed.
Justin Mannhardt (11:34): Yeah. It's guaranteed, highly attainable, no reason not to. The AI wave, you almost need to break that sucker down into categories. I sense two major conclusions that seem to trend on social or when people are talking about what you should be doing to get ready for AI. They're either saying something to the effect of good AI relies on good data. Maybe they're not talking about Power BI. Just in general, they're like, "If you want to get... You're going to need to do that."
(12:06): And then the other thing when people are like, "I'm interested in generative AI. How it can help my business?" And you ask, "Well, what should we do?" You hear something to the flavor of you should have an AI council or team to try and figure that out." It's very exciting. It's very elusive. I'm still betting that over the next period of time, there's going to be a lot of change and a lot of disruption. It's so uncertain that the for sure thing is to get on your surfboard on the first wave and there's value there without a doubt.
Rob Collie (12:37): That second recommendation, you better have yourself an AI council, an AI team. I mean, that just gives me the heebie-jeebies. Imagine how that plays out at an average corporate environment. There's going to be an AI council. Oh no, I better be on that. All of the fear and FOBO cycle that's playing out on social media now plays out for real right in your backyard. I'm going to get left behind if I'm not... I need to be on that leadership council. If you're honest with yourself, you don't know what to do with AI, but you know need to be on that council.
Justin Mannhardt (13:13): OpenAI, they're a little over a year into their big splash with releasing GPT to the world. I do agree, leaders need to be paying attention to this and understanding how it will or it won't disrupt. I think the creative entertainment space buckle up. Our space I think is just a little different. I'm not saying ignore it, but if you manufacture something, your manufacturing organization, you sit around. How should we be using AI, ChatGPT, Copilot? What are we talking about here? If you're not mature on the first wave, spend your energy there, I think is the smart move.
Rob Collie (13:50): One of the reasons why I like to do these sorts of talks is it forces a style of thinking like a discipline that you really can't fake unless you're preparing a talk and then delivering it and finding out how people respond to it.
Justin Mannhardt (14:04): You really got to embrace reality in your own conviction.
Rob Collie (14:08): Yeah, that's right. And the pressure to clarify and to deliver a message of value in public. We should be holding ourselves to this own standard even within our own heads, but it's really, really not practical.
Justin Mannhardt (14:22): No.
Rob Collie (14:22): And so there are a couple of things that came out of this that really kind of were epiphany level for me. I love those moments where a really important puzzle piece kind of snaps into place. I do want to get to a couple of those. So my big picture goal was last week in this presentation was to talk about the two waves. Acknowledge that the second wave, this AI wave is terrifying, but then dial it down, de-terrify it so that we can see it in perspective and then not forget about that first wave.
(14:52): It forced me through the why is AI scary? First of all, it just threatens replacement of jobs. No one talks about AI in the media without talking about job replacement. You sent me the Scott Galloway that I put in this talk where he just, in my opinion, takes a pretty huge leap connecting the dots between tech companies with their record profitability and their layoffs. That's not a difficult connection to make. We're making the same revenue.
Justin Mannhardt (15:24): Yeah. I've seen that over time.
Rob Collie (15:26): We're a tech company, so our staff is largely R&D. So if we cut back on that... Our revenues stay the same, at least for a while. You might need new R&D at some point, but I mean, imagine how profitable Microsoft would be for six months if they just got rid of all their engineers. They'd run the most record profits of all time, then they'd fall off a cliff. But then he also makes, Scott made the leap in my opinion, that these layoffs were because of AI.
(15:50): Now, I asked the room that I was presenting to, if anyone in the room had known anyone at this point that's lost their job to AI and one person raised their hand. It wasn't a huge room. It was like maybe 40 people. We dug down into the detail of that. It was kind of like a call center scenario where they reduced staff there because the chatbots took on some percentage of the front line of customer service.
(16:21): It's a huge leap from that to thinking that Facebook Meta is laying off tens of thousands of tech workers because AI... I'm usually really impressed with Scott Galloway. I wasn't impressed with that. That was too much of a leap for me.
Justin Mannhardt (16:36): So you made me think about something interesting here. So something like customer service. Let's just follow the arc of that function back a few years. You would call a company and you would talk to a human right away, and then you were introduced to a recording with a series of prompts. There's been this arc of trying to drive people out of that function for a while. I can see like, "Oh, let's just accelerate this."
(17:04): Side note, companies are getting sued because these chat bots are giving poor information to people and that's sort of an interesting tangent. The other thing with tech companies is if these chat-based services have proven to be semi-competent at anything, its routine code is like a thing. So you do wonder if they're like, "Oh no, we're just going to figure out how to be more efficient." There is a legitimate sort of functional, how does this impact what we do?
Rob Collie (17:35): And you've also seen that a lot of organizations eventually had to backtrack from that automated menu and get you straight to a human sooner.
Justin Mannhardt (17:45): Yeah. A lot of overcorrection will be... That's a great insight.
Rob Collie (17:48): You can see the object on the end of the spring sort of oscillating back and forth through the origin.
Justin Mannhardt (17:55): Turns out these things hallucinate. We got sued a couple times, so we're going to just walk this one back.
Rob Collie (18:02): So threatening job replacement is bad. Early returns, though, from the real world. I still don't know anyone at one degree of separation from me who's been replaced by AI. The articles are written like it's already happening. I don't see a lot of evidence of that yet. So remember, the whole game here is to kind of de-fang it a little bit. Not to take it easy, not to be complacent.
(18:24): When you're scared, you don't think clearly. Let's de-scare. Second thing I identified about why it's so scary is that it just seems like it's accelerating like crazy. Like you said, it wasn't that long ago, we never even heard of ChatGPT. And now we've got, what's it, Sora?
Justin Mannhardt (18:40): OpenAI's video generation model. Yep, Sora.
Rob Collie (18:45): I describe it as like you're on a roller coaster in the dark and you don't know is this one of the big drops? You're at the beginning of a drop. Is it going to just catch really soon or is this one of those three second like, "Oh my God." You can't tell. Right?
Justin Mannhardt (19:01): Right.
Rob Collie (19:02): So bottomless and accelerating.
Justin Mannhardt (19:04): There's maybe a lack of depth in that acceleration. Innovation of any kind go as far back in history as you want to, has always resulted in some economic disruption of some kind like industrial economic disruption of some kind. This will be no different. There will be some effect of that. It feels like that acceleration, it's already arrived. There will be change as a result of these technologies, but it's not like we woke up one day, we didn't have robots making cars, and then the very next day we did.
Rob Collie (19:38): Yeah. So my next point of why it's so scary is because AI has become the greatest generational gift. This is a once in a generation gift to marketers and influencers. All you have to do is play the scare factor. You don't even really need to know what you're doing. You can be vague. It's just magic, and you get those clicks.
Justin Mannhardt (20:02): Like and subscribe, baby.
Rob Collie (20:04): That's right. We don't need a like button for this. We need the "ew".
Justin Mannhardt (20:06): Yeah. We need the "ew" button
Rob Collie (20:11): It literally was ew. What's going on here? So jumping to the, I think the first super, super juicy one. This is one of the epiphanies that I had as a result of preparing this. When you think about AI and you think about the things that, the most dramatic examples, the ones that are getting your attention and unsettling you, they're usually things that are generating images or video.
(20:35): Pictures are worth a thousand words. That's a real thing. Pictures are compelling where visual creatures. And what's more compelling than images? It's video. It occurred to me, this is cheating. Images and video, while they seem like the richest, most difficult content to produce, they're actually the easiest things for AI to produce because when an AI produces, when some sort of model produces an image and the people in the image have seven fingers, or their coffee mug is floating in midair with nothing holding it up, we can look at that and spot it and say, bad bot.
(21:18): We are not going to use that image. And so it's okay to ask it for bajillion variations until you get the one where there's not seven fingers and the coffee mug isn't floating in the air, or maybe you have to go and hand edit somehow, use it as a starting point.
Justin Mannhardt (21:34): Have you seen the ASCII art thing yet?
Rob Collie (21:37): No.
Justin Mannhardt (21:38): I saw it this morning. So it's, "Hey, ChatGPT. Do you know what ASCII art is?" It says, "Yes." "Oh, can you spell Honda in ASCII art?" It's just like a bunch of slashes and it just doesn't spell anything. Eventually it's like, "Okay. Do you know what the letter H is?" "Yes." "Can you make an H in ASCII art?" And it does. It's like, "Oh, now make an O." It takes it so many times and it finally gets it, but the letters are different sizes and it looks terrible. It's just like...
Rob Collie (22:07): Yeah. Well, so that'll be something that right now some researchers are working on furiously and we're going to have a tightly tuned.
Justin Mannhardt (22:14): We'll fix that one.
Rob Collie (22:15): Yeah. That'll be no problem. Really, the problem with AI isn't getting it to generate an answer. It's knowing that you can trust it.
Justin Mannhardt (22:24): That's right.
Rob Collie (22:26): And that is such a simple thing to say, and it sounds like it's trivial, but it's actually everything. Getting these things to spit out answers is nothing. Getting them reliable and trustworthy is the entire challenge.
Justin Mannhardt (22:43): You're introducing, not you specifically, the proverbial you, you're introducing the depth of answering the question, what is truth? If these things are going to be trustworthy, not only do they need to generate a response or an image or a piece of audio, a piece of video, there needs to be some sort of computational engine that says, "Was that correct?" According to who? And it's really interesting.
Rob Collie (23:12): Mid-journey blows my mind.
Justin Mannhardt (23:14): It really does. Yeah.
Rob Collie (23:16): All these image generators. The other day we asked Luke for the female version of mullet man for the podcast without telling him that we already had this article.
Justin Mannhardt (23:28): Sorry, bud.
Rob Collie (23:32): Off he went.
Justin Mannhardt (23:34): He really did.
Rob Collie (23:36): There were some good images generated by that. I mean, they're all kind of more 3D than our original. Right?
Justin Mannhardt (23:43): Right.
Rob Collie (23:44): There's no real in those applications because you're only going to use the images that you as a human being, validate. We can look at an image and know if it's wrong. I mean, okay, fine. Maybe every now and then we won't notice that someone has three fingers. Something doesn't line up. But in general, the risks are low and human beings can quickly validate the output.
(24:07): Whereas self-driving cars, they didn't have any trouble getting those things to go around a parking lot. They were already steering, connecting the reasoning model that they've built up to the steering of the car was trivial, getting output, getting it to produce answers. But how do you trust it in a more tangible, short-term thing? Let's say I just want to do away with dashboards. I want to stop writing formulas. I just want to feed a bunch of data into some sort of LLM model and start asking it questions.
(24:43): How do I know the number that it gives me is correct? I look at the number. I have no way to validate it. I can validate a picture. I can validate a video like, "Oh no, the physics look wrong in that. That ball bounced way too high from the height that it was dropped," or whatever. Our biological machinery is built to evaluate visual fields like that. Human beings are the far away champions of the entire animal world in terms of our ability to throw things accurately.
Justin Mannhardt (25:14): Makes sense.
Rob Collie (25:15): They've tried to teach chimps and stuff to throw things. No matter how long they train, how long they practice, whatever, they are just terrible at it. We are built to evaluate those sorts of things and we can do it in a snap. We look at a number, we have no idea if it's right. Take that number and feed it into your production system. That's how many of these widgets we should produce this month. You're going to trust that? Even if it's right, nine times out of 10, if it's off by a lot on that 10th one.
Justin Mannhardt (25:44): I do use these generative AI LLM style tools. I'm using them every single day at this point, and it's helping me in different ways. To me, it's filling in these little cracks and crevices or it's giving me a little boost here and there. It's like, "Oh, I need a rubber duck. Thanks for being my rubber duck." I can't put my finger on this is a seismic shift use case yet.
(26:11): That's where I start to be personally more calm about this. The other day, I needed to... I was trying to run a script that goes off and gets some information from Power BI. We moved all of our files at P3 and so I couldn't find where this thing that I used to know where it was. I couldn't find where it was, and so I just asked Copilot. I was like, "How do I do this?" And it was like 99% right.
(26:32): But it saved me five minutes of looking around for something. And the images and the video and the stuff, that's compelling. If they can figure that stuff out, you think like advertising, influencers, social, all that kind of stuff where it's supposed to be whimsical and fantasy and that kind of stuff, I can see where that starts to come in.
Rob Collie (26:52): It's also already kind of worn out in a way. You scrolling through LinkedIn and you see an image go by, you know that it came from mid journey or similar and it just lands hollow for some reason.
Justin Mannhardt (27:05): You can tell with writing as well. I feel...
Rob Collie (27:07): Oh yes, you can. Oh yes, you can. I think that just was a really key clarity for me is that it's not building systems that can produce answers. It's the validation and trust building process. Being able to tell the difference between valid results and invalid results because the system is going to be just as confident when it's telling you to fly the plane into a cliff. And this is very charitably called the hallucination problem. When AI is wrong and it just produces incorrect results, we call it hallucination as opposed to an error or... I don't know if this is a charitable way to describe this or if it's an uncharitable way.
Justin Mannhardt (27:59): All right, Rob, what's the over under... In the next five years, there's a function in Microsoft Excel called if hallucination.
Rob Collie (28:07): Well, again, how do we know? I think the chance is zero that that function exists.
Justin Mannhardt (28:13): If hallucination, if error.
Rob Collie (28:16): Yeah. If we could write the function that detects whether the AI is hallucinating, then it's all over. Right? Because you just run a million trials and only keep the ones where I just don't think there is such a thing.
Justin Mannhardt (28:28): Yeah. It seems like a very difficult problem to solve.
Rob Collie (28:31): People are using LLMs, not everybody, but a lot of people are to apply for jobs with us.
Justin Mannhardt (28:38): It's obvious.
Rob Collie (28:39): Just like you can see the AI generated images and go, "That one came from a mid-journey or a clone." The answer to some of these long form questions, and I even put this in my presentation last week as an LOL as a use case. Some of them are long form, but we also have the desire to technically screen a little bit just to make sure that someone knows what they're talking about before letting them go further.
(29:01): Well, depending on how you structure the question, these LLMs will give you precisely the right answer, meaning there's no point in asking the question if everyone's just going to go off and ChatGPT it and bluff that they know when they don't. But it's telling that if you engineer the question the right way, the LLMs start to produce incorrect answers and they sound just as correct as the correct answers did.
Justin Mannhardt (29:30): And there's a level of confidence in its response, which is interesting.
Rob Collie (29:34): So we're actually in a business situation right now where I am engineering so that the LLMs of today produce hallucinations, and I'm able to do that without touching their code.
Justin Mannhardt (29:47): Yeah. When you write a software program, you can unit test the damn thing. Inputs, outputs. I expect... You can't do that.
Rob Collie (29:56): No, you can't. Otherwise, we'd already have the self-driving cars, right?
Justin Mannhardt (30:00): Yeah.
Rob Collie (30:01): If you had "if hallucinate" function. This is just like what was two weeks ago for me, this sort of detail on the map, like the "hallucination problem". But if they had described it instead as the getting to the point where you can trust it problem, that's everything. That's the whole thing. It's not building the system. That's easy.
Justin Mannhardt (30:23): So you've seen this stuff where it's like, "Oh, this model passed the bar exam or this model correctly diagnosed serious illness like obscures illnesses more correctly than doctors." You see these types of studies, and I was thinking about this the other day. You step back and you say, "Okay, those scenarios have a large volume of human record and consensus. The debate is over." I'm like, "How do you pass the bar exam? Based on how these things work, it's forming its opinion based on an established consensus." And so that kind of squares for me on those types of examples.
(31:04): Will this type of technology ultimately fuel or stifle, or obscure innovation in some way? Because its opinions are formed by the consensus of information that's already been produced.
Rob Collie (31:19): Yeah. And that's the other problem with it is biases leak in.
Justin Mannhardt (31:24): Yeah. Some people that have wildly failed, like the Google Gemini examples, if you've seen some of those, to what extent are we controlling for bias? To what extent are we trying to control for truth, what are we really getting as a society? I think it's good. I think it's neat, but how? How much?
Rob Collie (31:44): Yeah. This is the single biggest moment where I've just been like, "Okay, the idea of trusting AI in grandly unstructured scenarios."
Justin Mannhardt (31:54): Yes.
Rob Collie (31:55): It's the most hypey thing. In short, I think the easy stuff in AI is what we're seeing right now.
Justin Mannhardt (32:02): Meaning what you're seeing is easy for this technology to deliver.?
Rob Collie (32:07): Getting it to generate results is still a technical marvel and not to be sneezed at, but getting it to generate results is the part that we've got. Getting it to generate trustworthy results across a wide range of scenarios is completely different, and it's why you're seeing in the case where it's generating art or it's generating marketing content, copy or whatever, a human being can very, very, very quickly referee and/or edit because you can look at it and tell if it's good or bad. The human is amazing judge of its output. A human being has no way to judge a numerical output. A human has no way to judge output that's code.
Justin Mannhardt (32:48): Or written.
Rob Collie (32:49): Even a written summary. Unless you already know enough to not have needed the AI to help you, and this is why when you see Sam Altman saying, "We need trillions and trillions of dollars of research." You don't need trillions and trillions of dollars of research if we're already there. Even in inflation adjusted terms, the Manhattan Project did not cost trillions of dollars. Searching for the cases or the hallucination problem isn't this world killer, which it is the world killer in so many scenarios right now. Separating that wheat from that chaff, that's the key.
Justin Mannhardt (33:21): I would agree.
Rob Collie (33:22): And also a way to sleep pretty easy, I think about the hype and the threat and everything. All right. Well, we solve that problem.
Justin Mannhardt (33:30): What's next?
Speaker 3 (33:30): Thanks for listening to the Raw Data by P3 Adaptive Podcast. Let the experts at P3 Adaptive help your business. Just go to p3adaptive.com. Have a data day.
Sign up to receive email updates
Enter your name and email address below and I'll send you periodic updates about the podcast.
Other Episodes
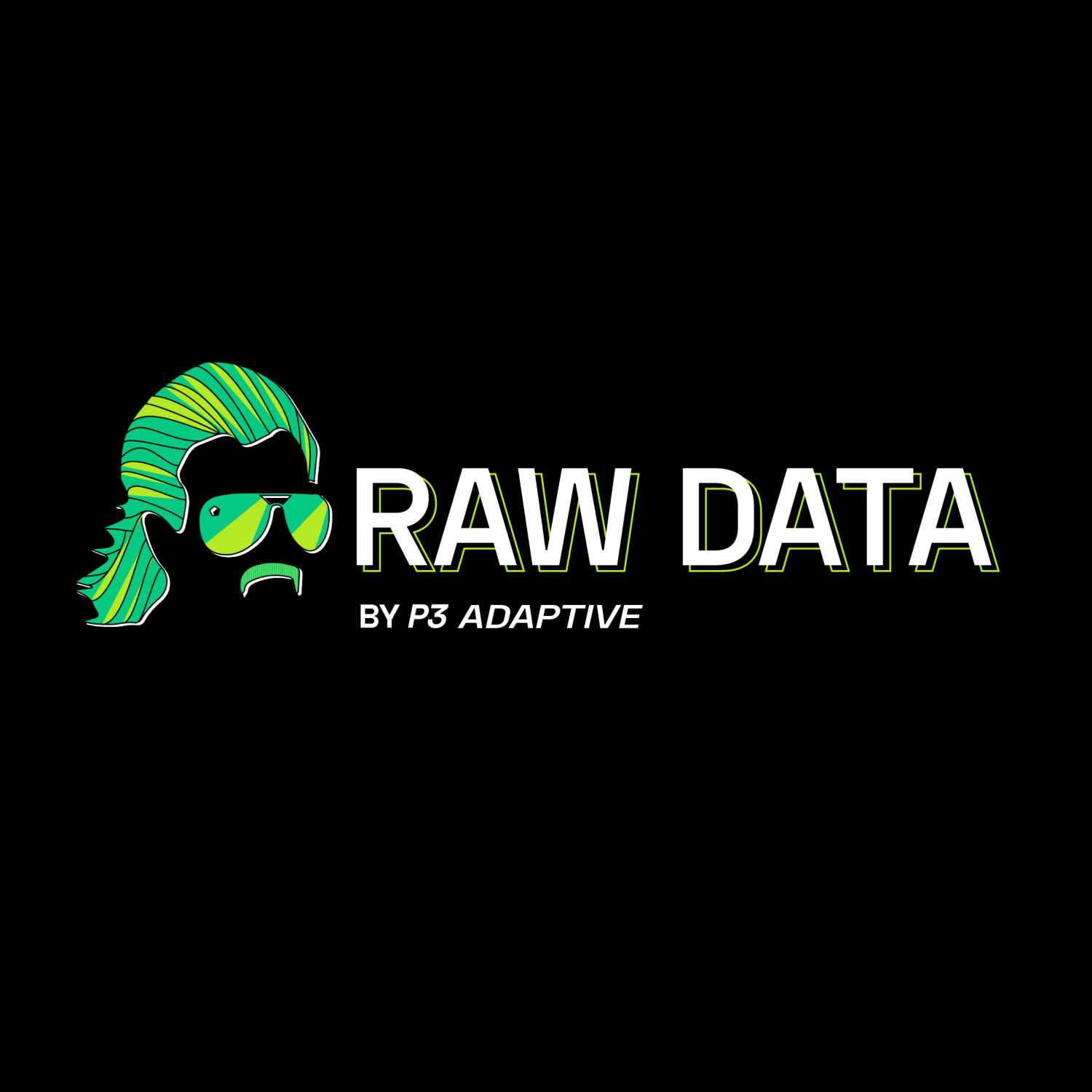
Adjacent and Between: Demystifying Digital Transformation with Power Apps and Power Automate
03.26.24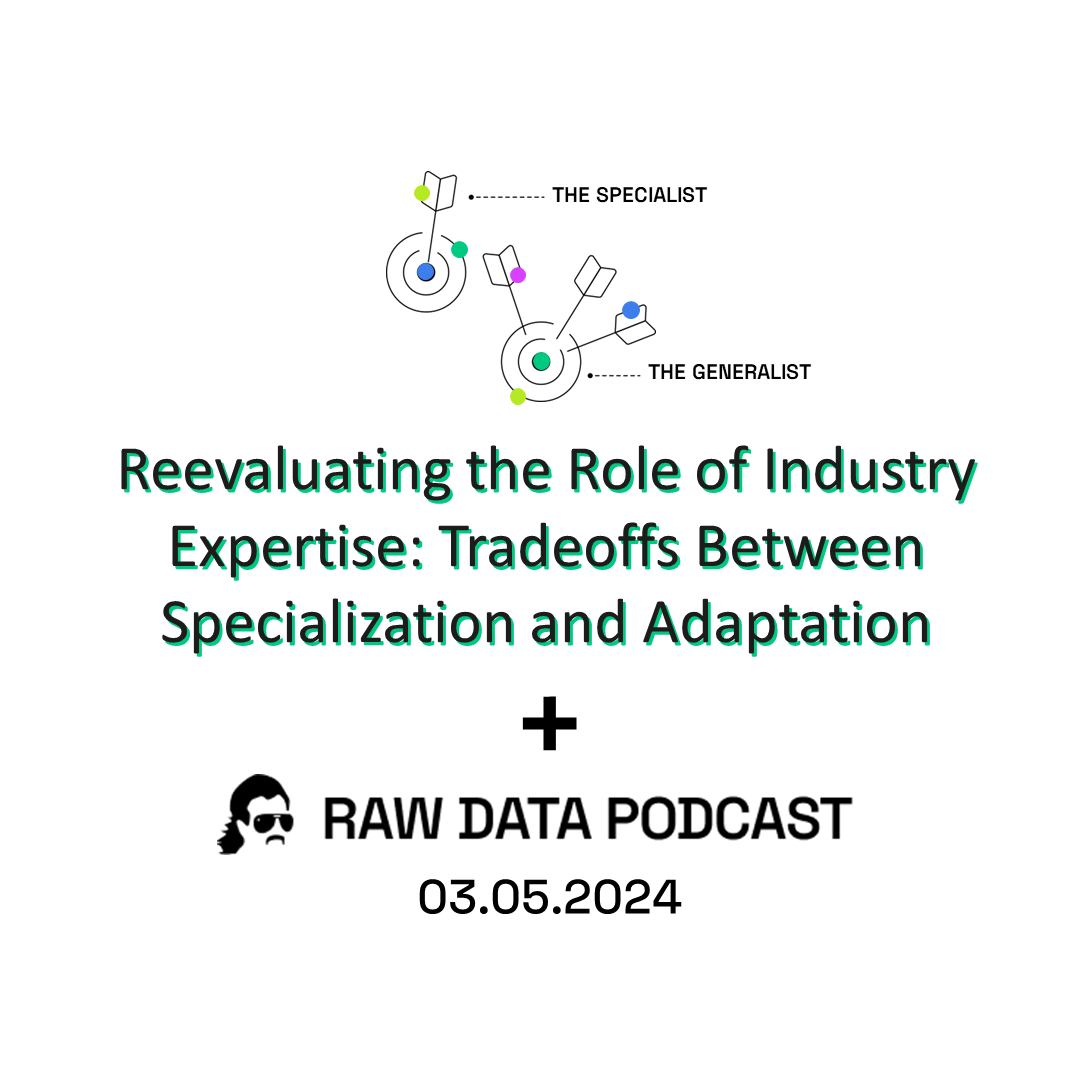
Reevaluating the Role of Industry Expertise: Tradeoffs Between Specialization and Adaptation
03.05.24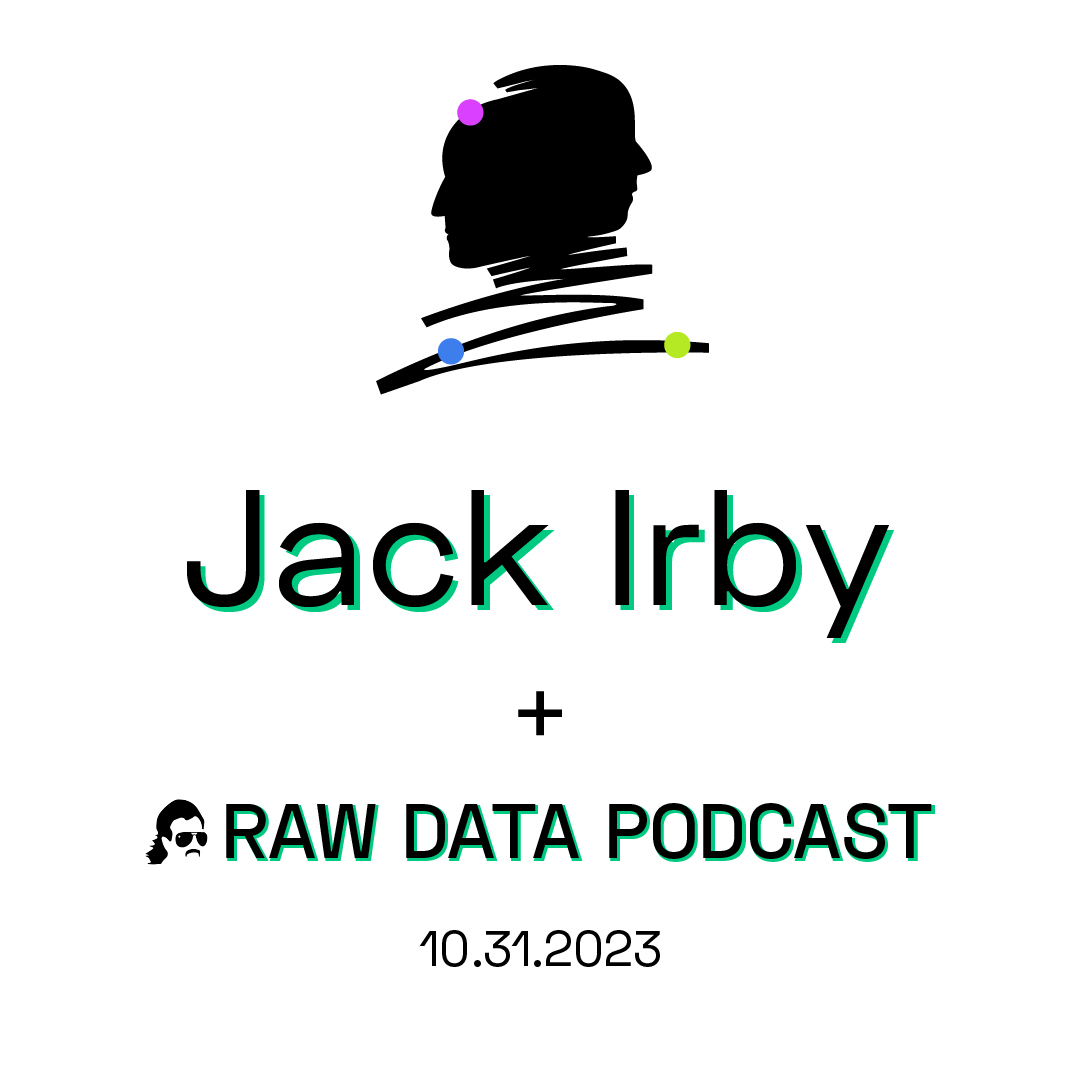
The Fruit of the Electric Grapevine: Industry-Leading Visibility on Limited Resources, w/ Jack Irby
10.31.23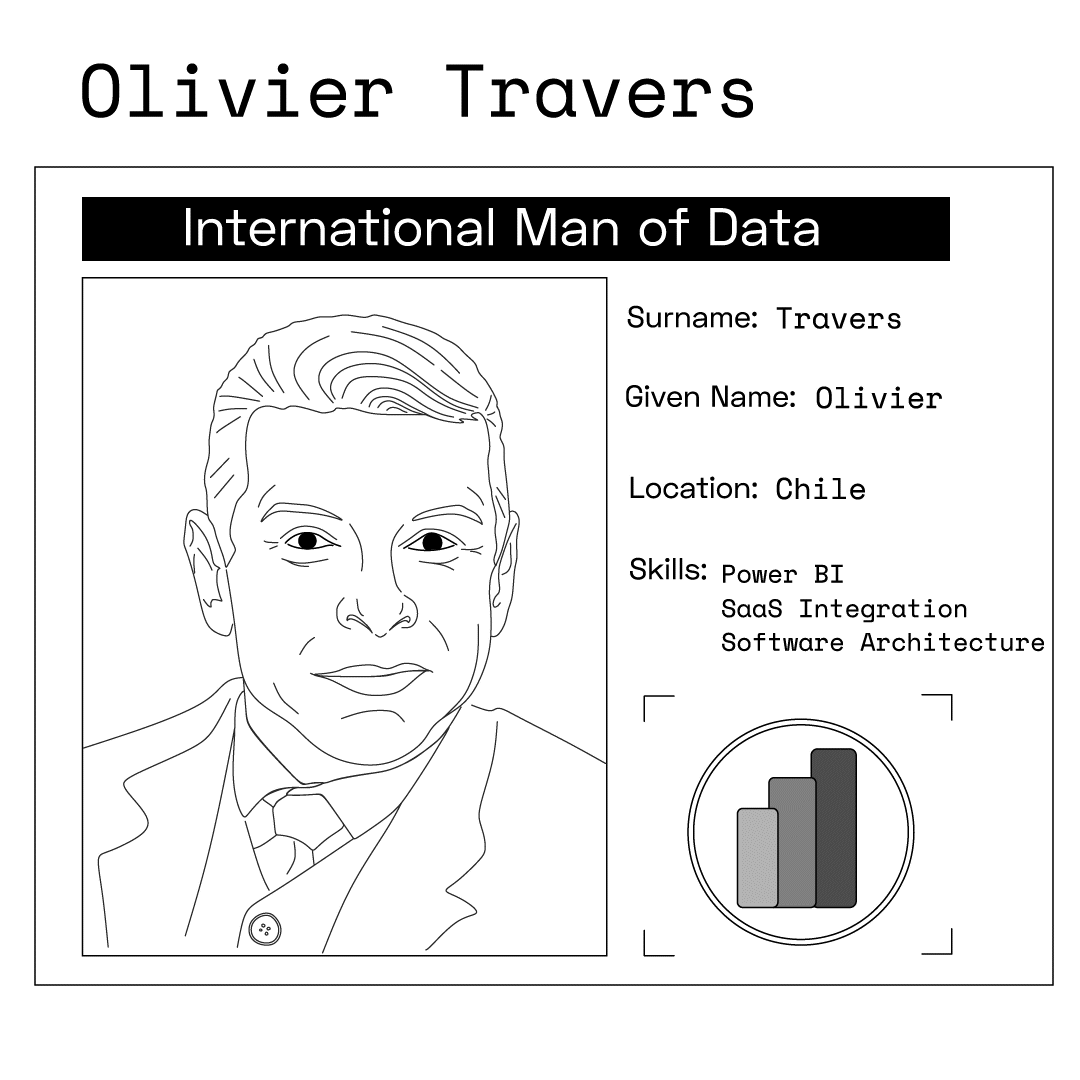
Stay Away from Guadalcanal, w/ Olivier Travers
MS Data Platform International Man Of Mystery
01.04.22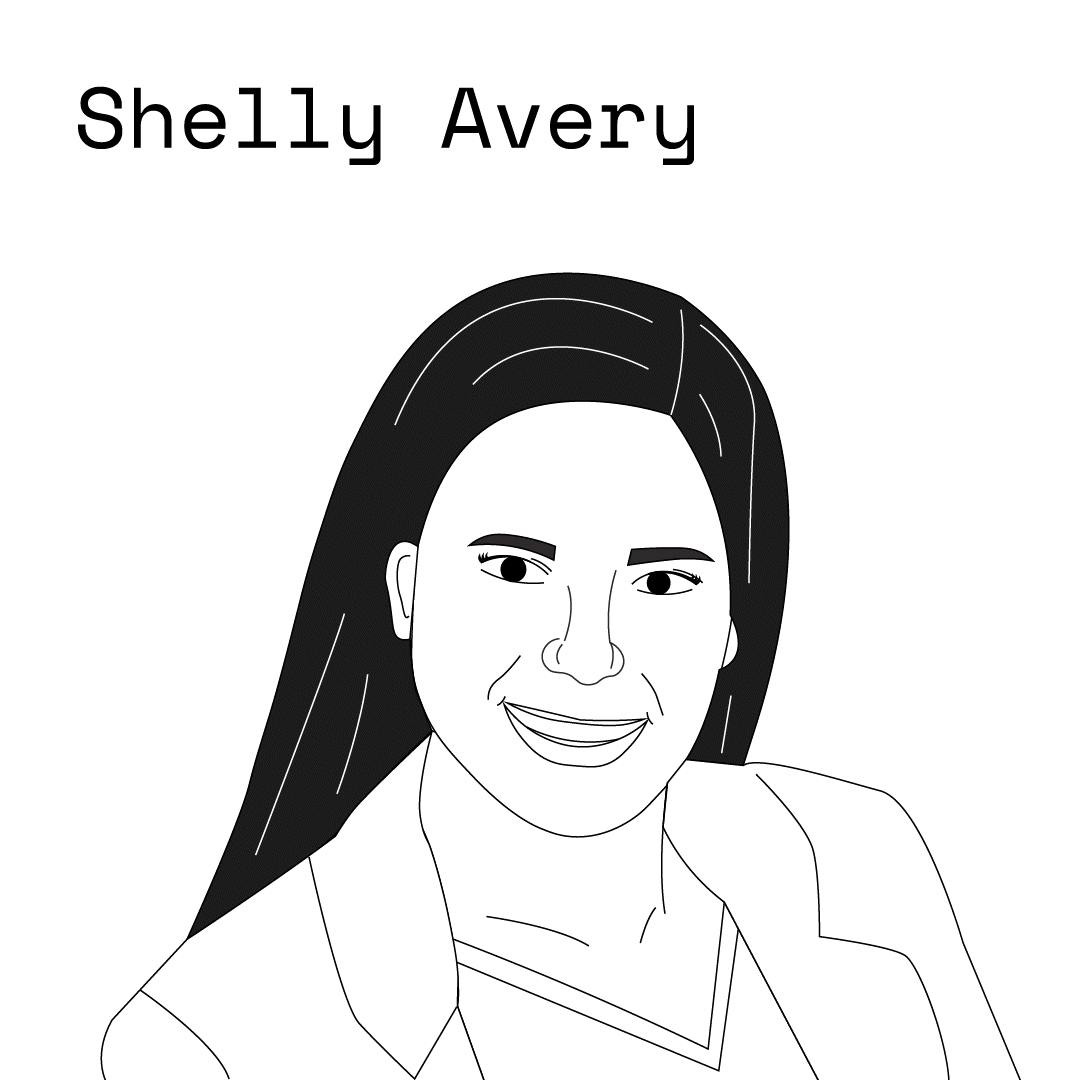
99% is the New Zero, w/ Microsoft Director Shelly Avery
Director-US Healthcare Specialist, Microsoft
09.14.21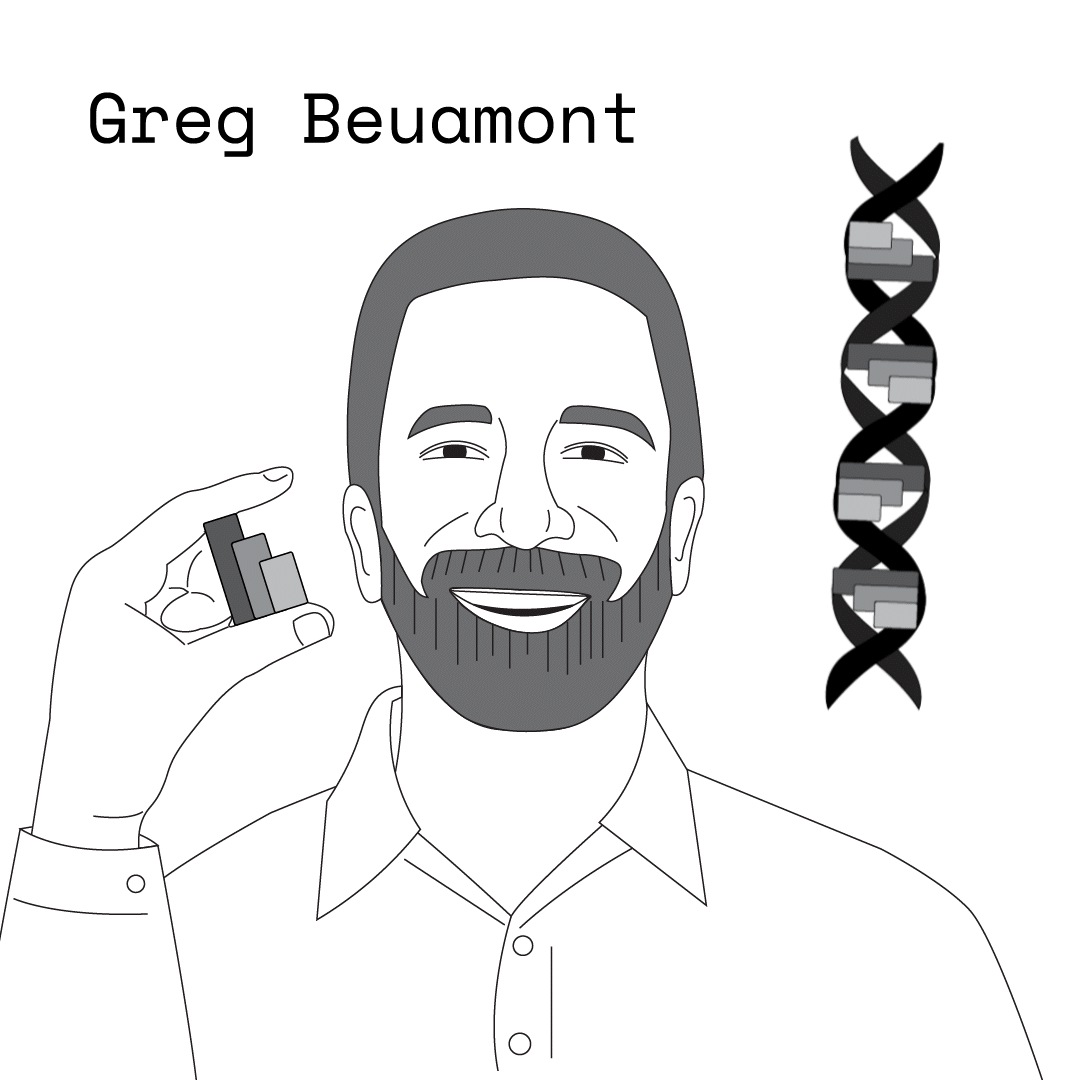
For Those About to DAX, w/ Microsoft’s Greg Beaumont
Senior Business Intelligence Specialist, Microsoft
08.17.21
Spreadsheet Celebrities & Power BI Playdates, w/ Chandoo
Hear from the Mozart of Excel Dashboards: Chandoo
08.10.21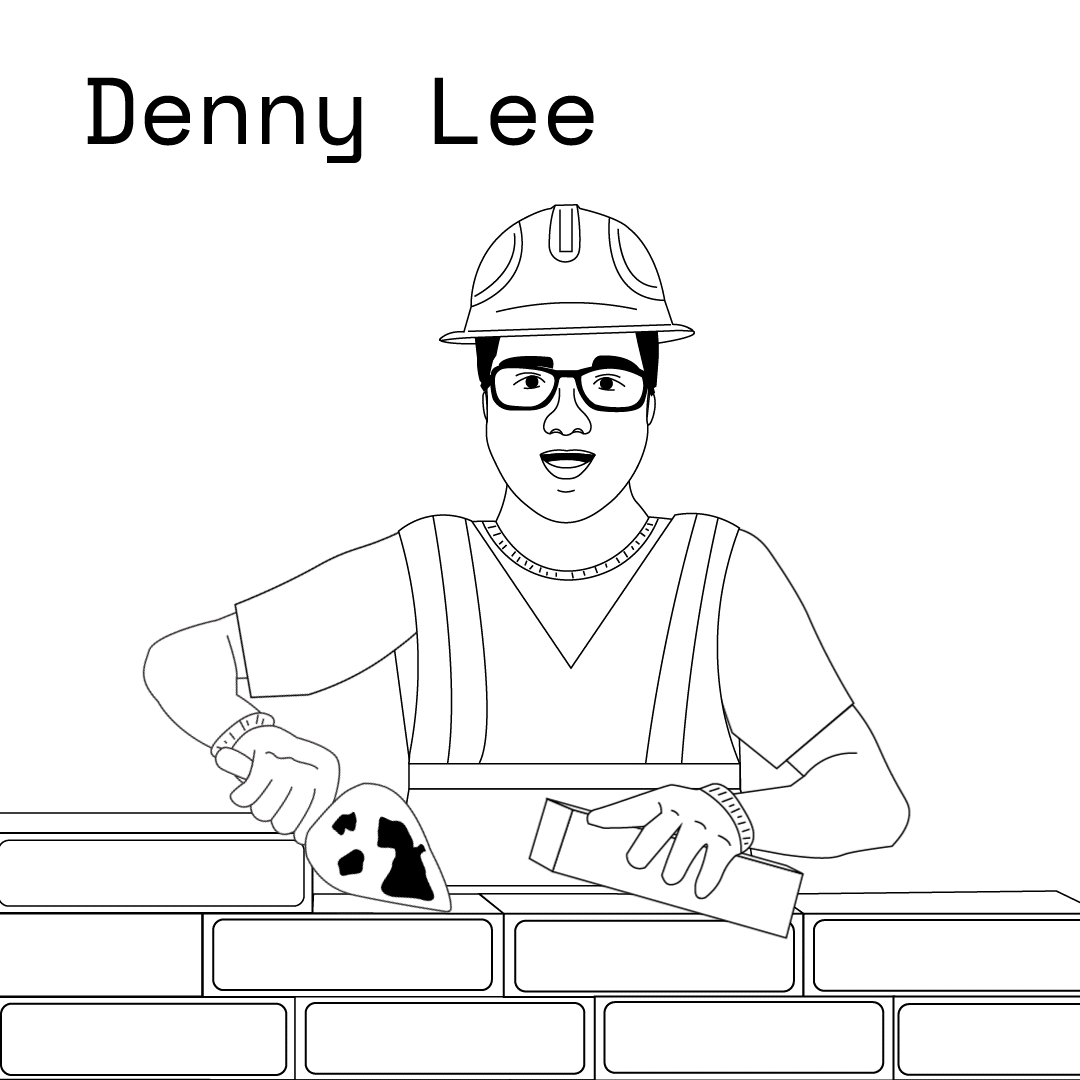
The Data Lakehouse: Brick by Brick, w/ Databricks’ Denny Lee
Sr. Staff Developer Advocate, Databricks
08.03.21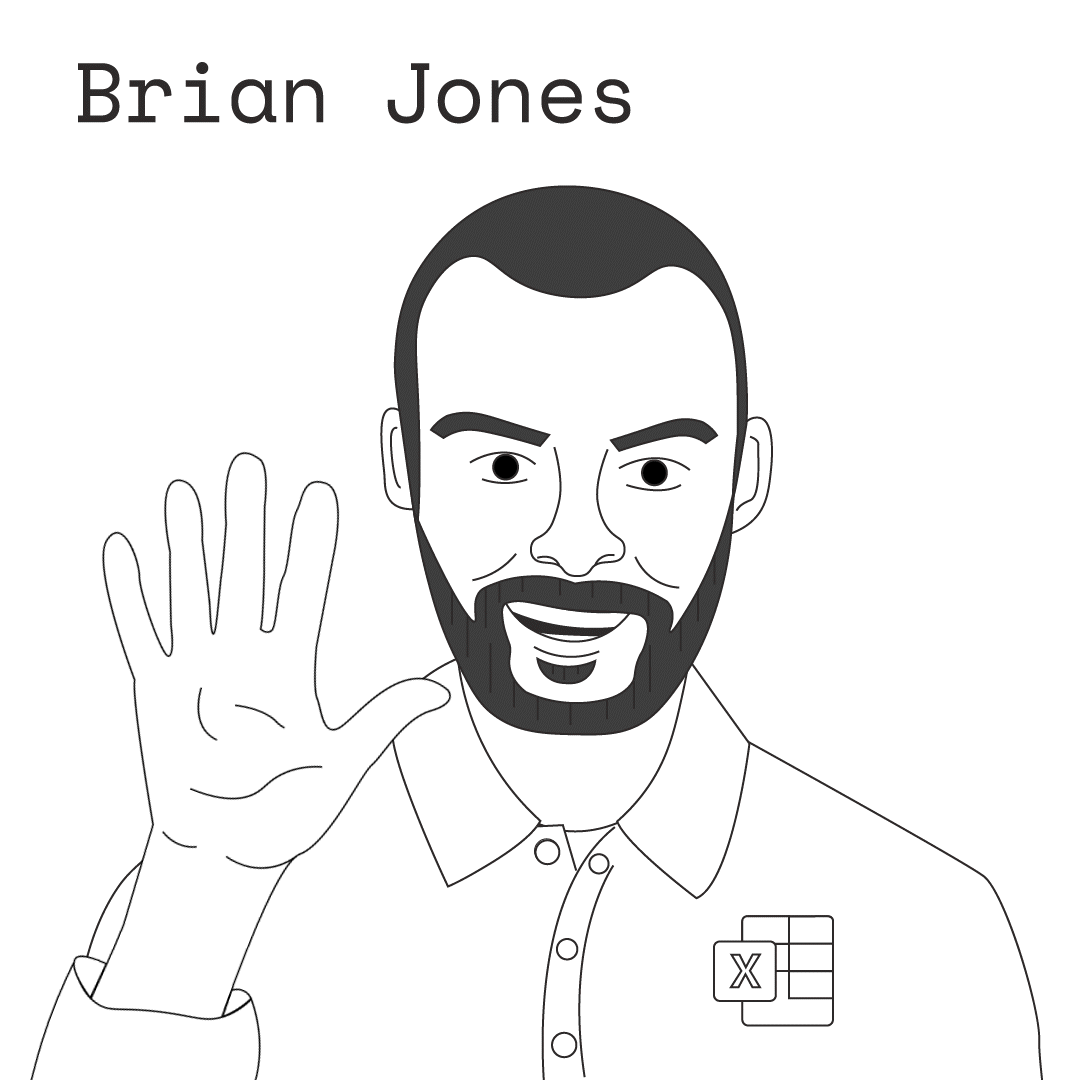
Excel Past/Present/Future, w/ Excel Head of Product Brian Jones
Head of Product, Excel-Microsoft
07.27.21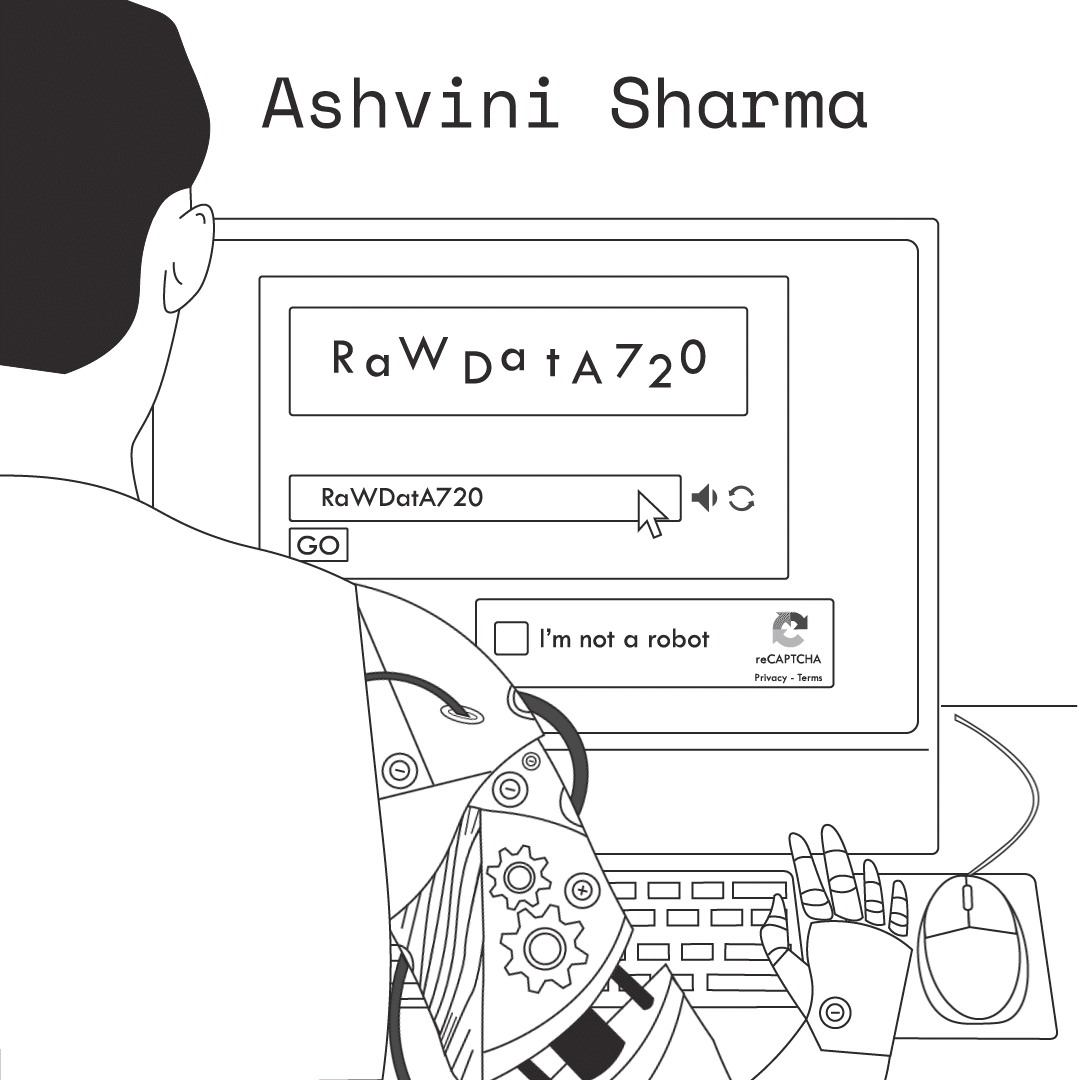
Robotic Process Automation for All, w/ Microsoft’s Ashvini Sharma
Group Program Manager-Power Automate RPA, Microsoft
07.20.21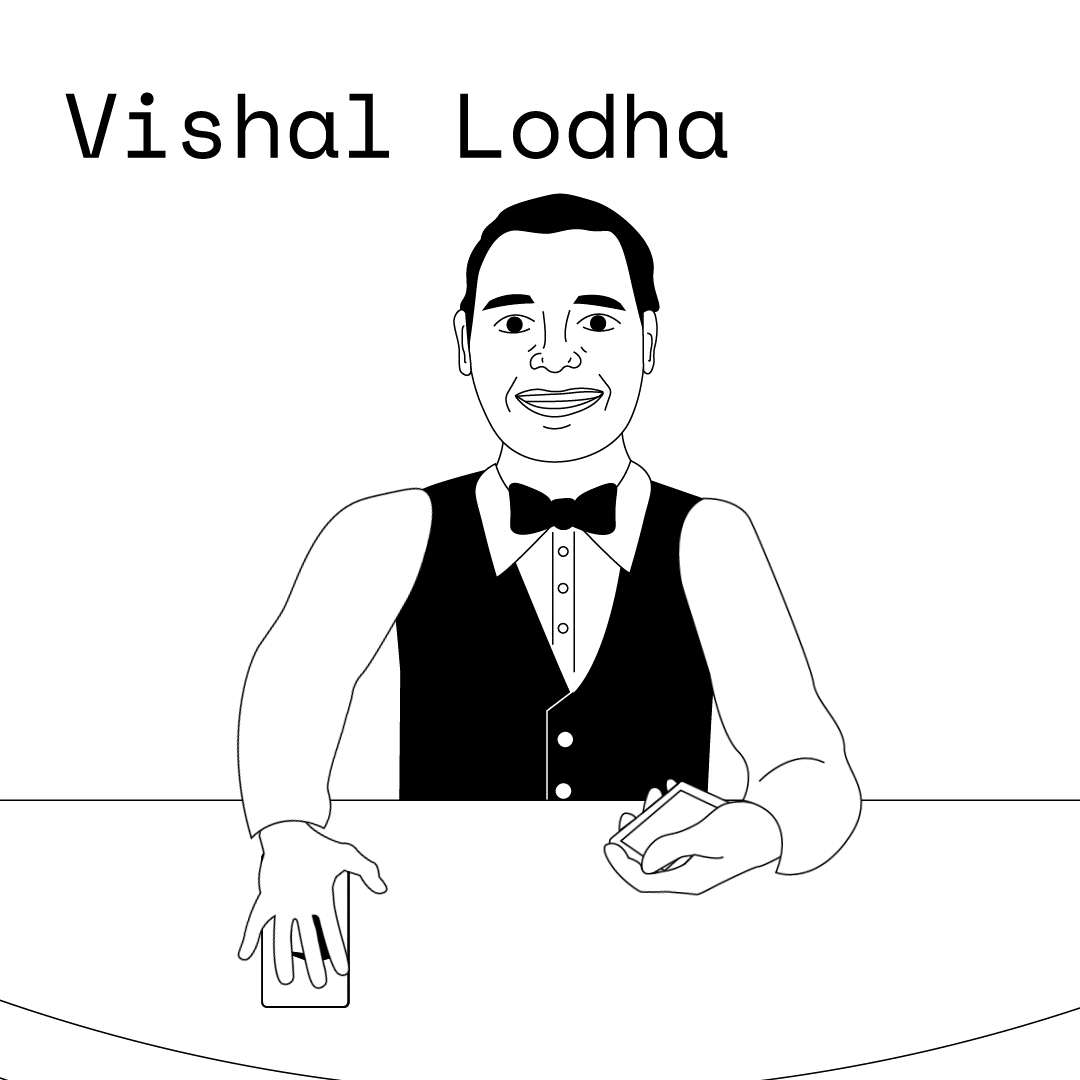
COVID Basement Racquetball, w/ Microsoft’s Vishal Lodha
Strategic Account Director, Microsoft
06.03.21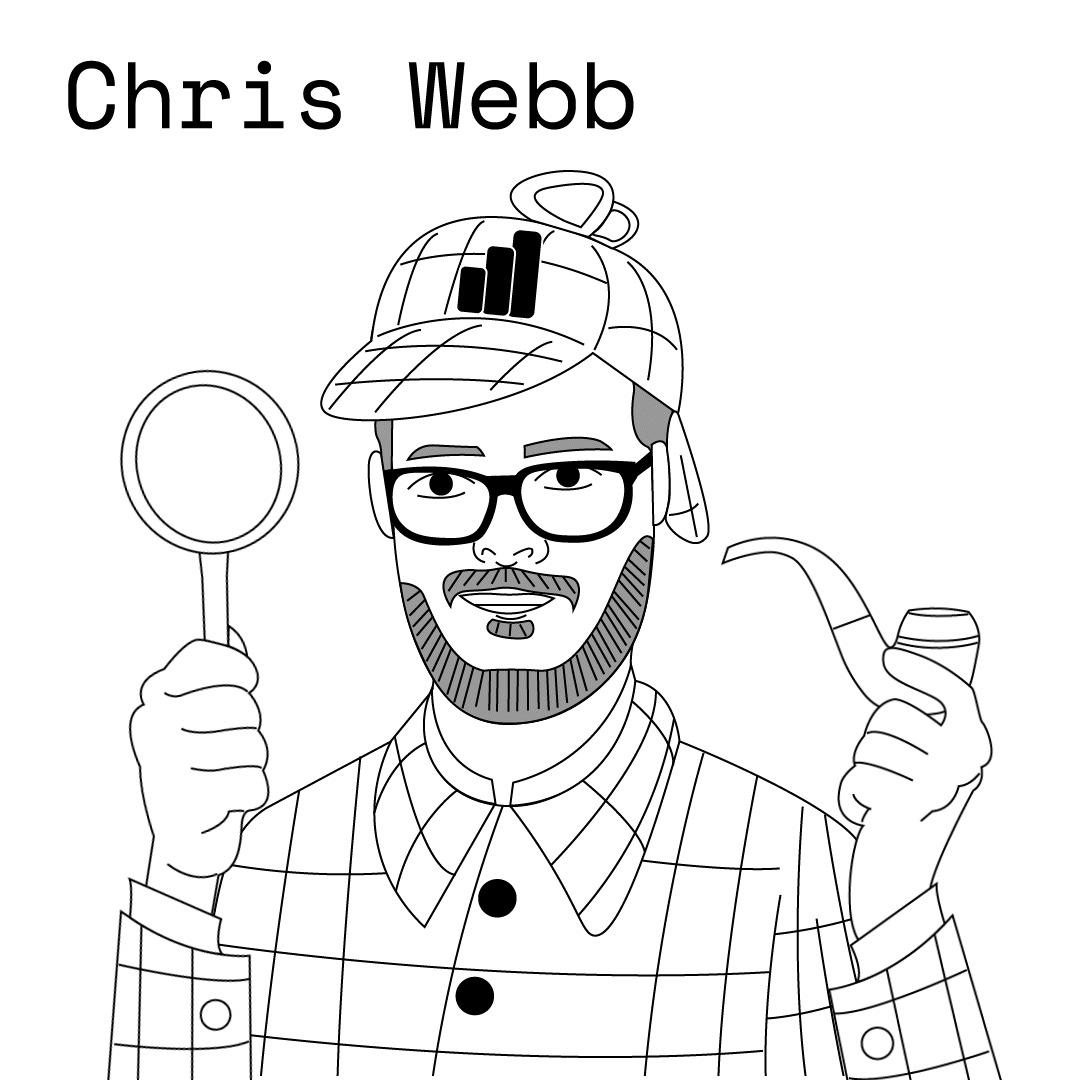
Surfing the Changing Seas of Data, w/ Microsoft’s Chris Webb
Principal Program Manager-Power BI Customer Advisory Team, Microsoft
06.01.21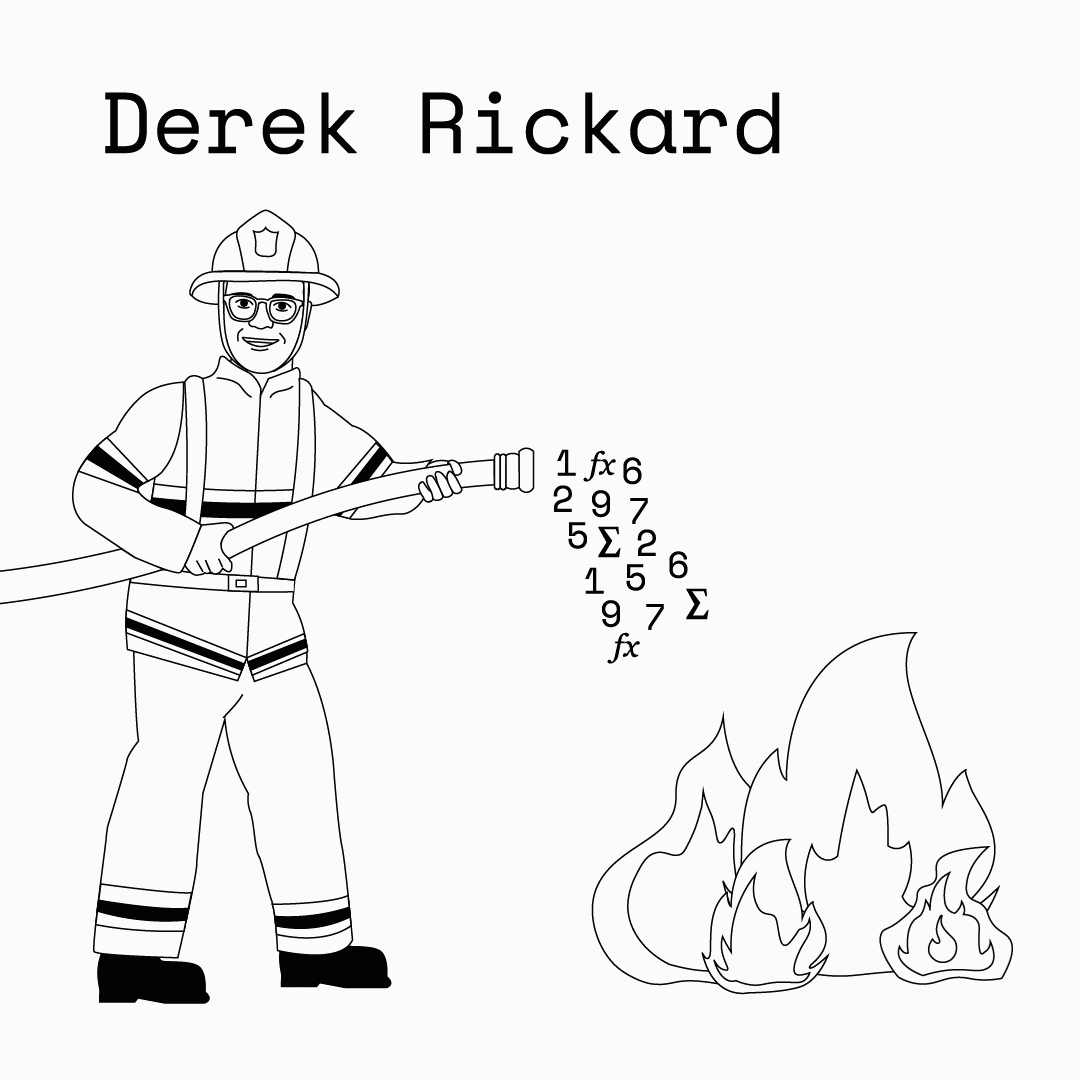
A Different Kind of Data Emergency, w/ Derek Rickard
Chief Data Officer, Emergency Reporting
05.04.21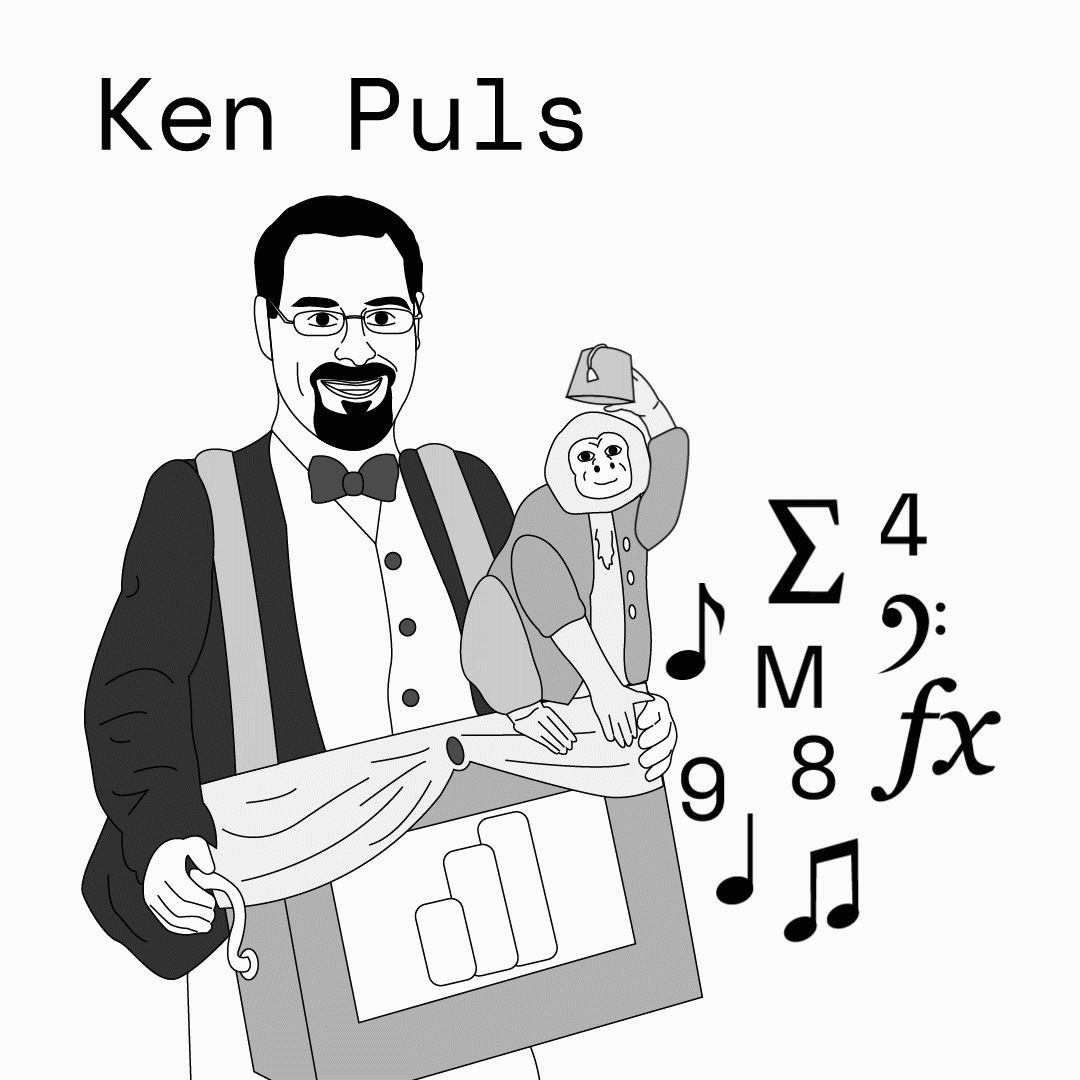
Earth’s Only Hope vs. Alien Excel Invaders, w/ Ken Puls
President and Chief Training Officer, Excelguru Consulting Inc.
04.27.21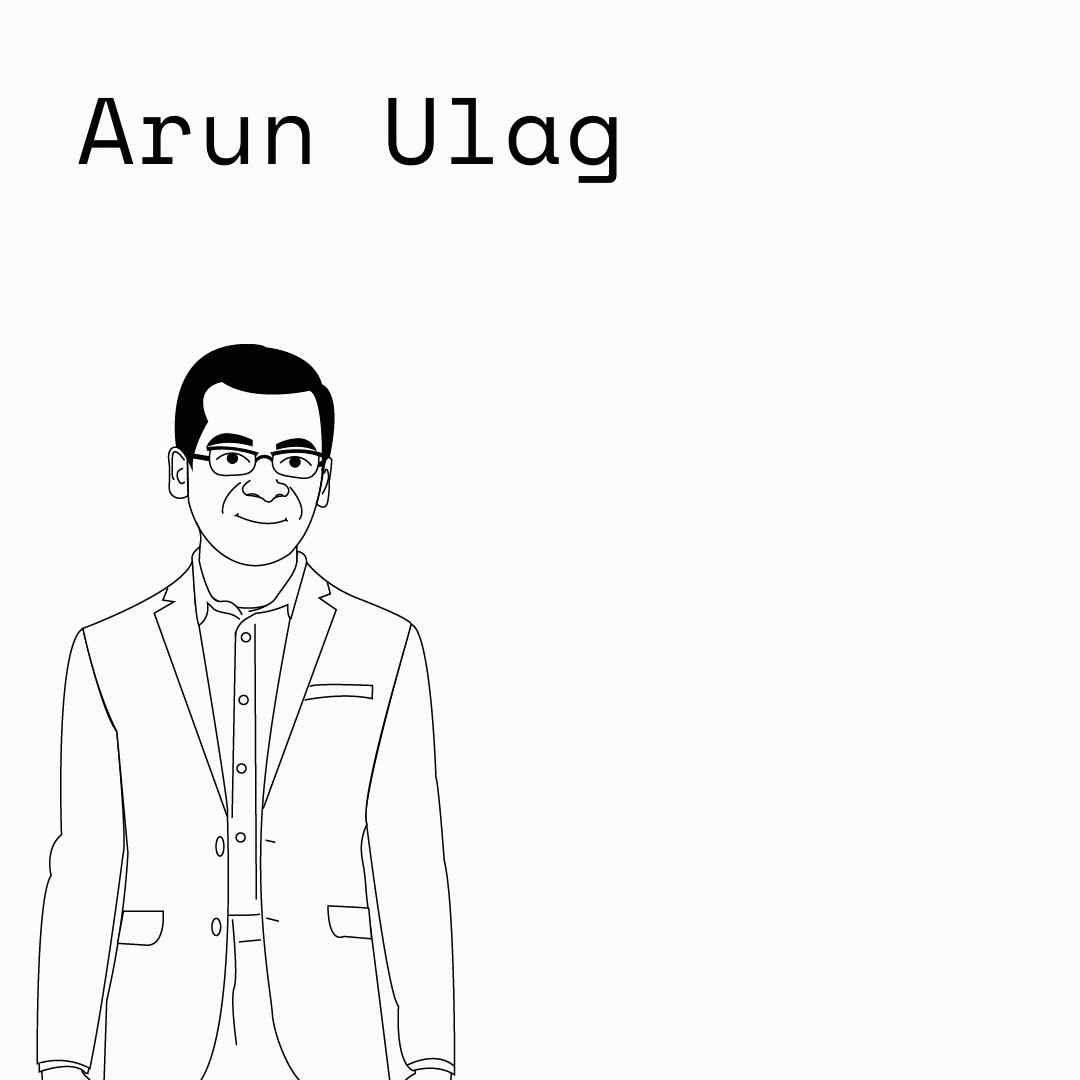
Intelligence Platform is a Cool Name, w/ Microsoft CVP Arun Ulag
Corporate Vice President of the Business Intelligence Platform at Microsoft
04.01.21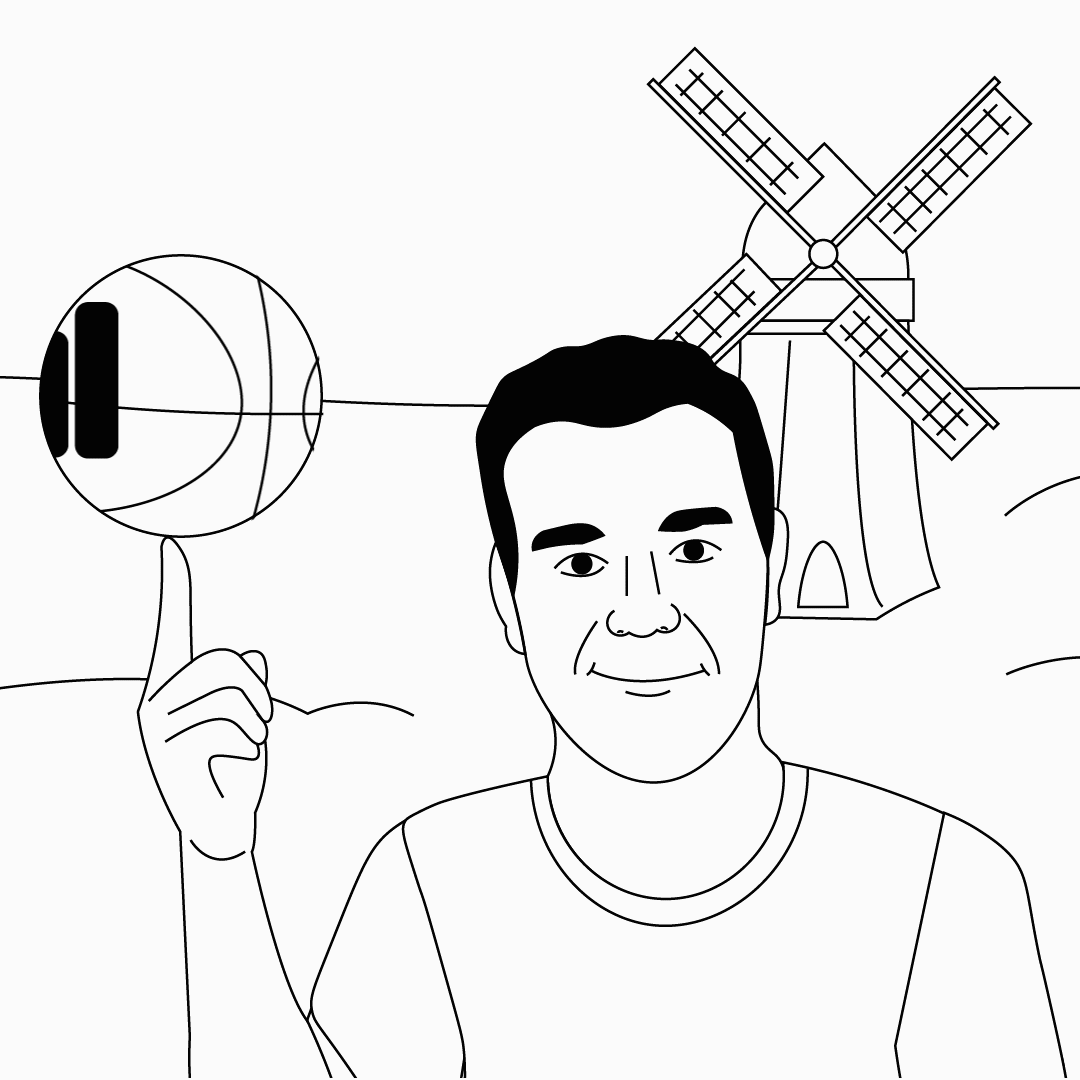
The Dutchman of Data Dunked on Me, w/ Kasper de Jonge
Analytics Advisor and Principal Program Manager-Power BI, Microsoft
03.23.21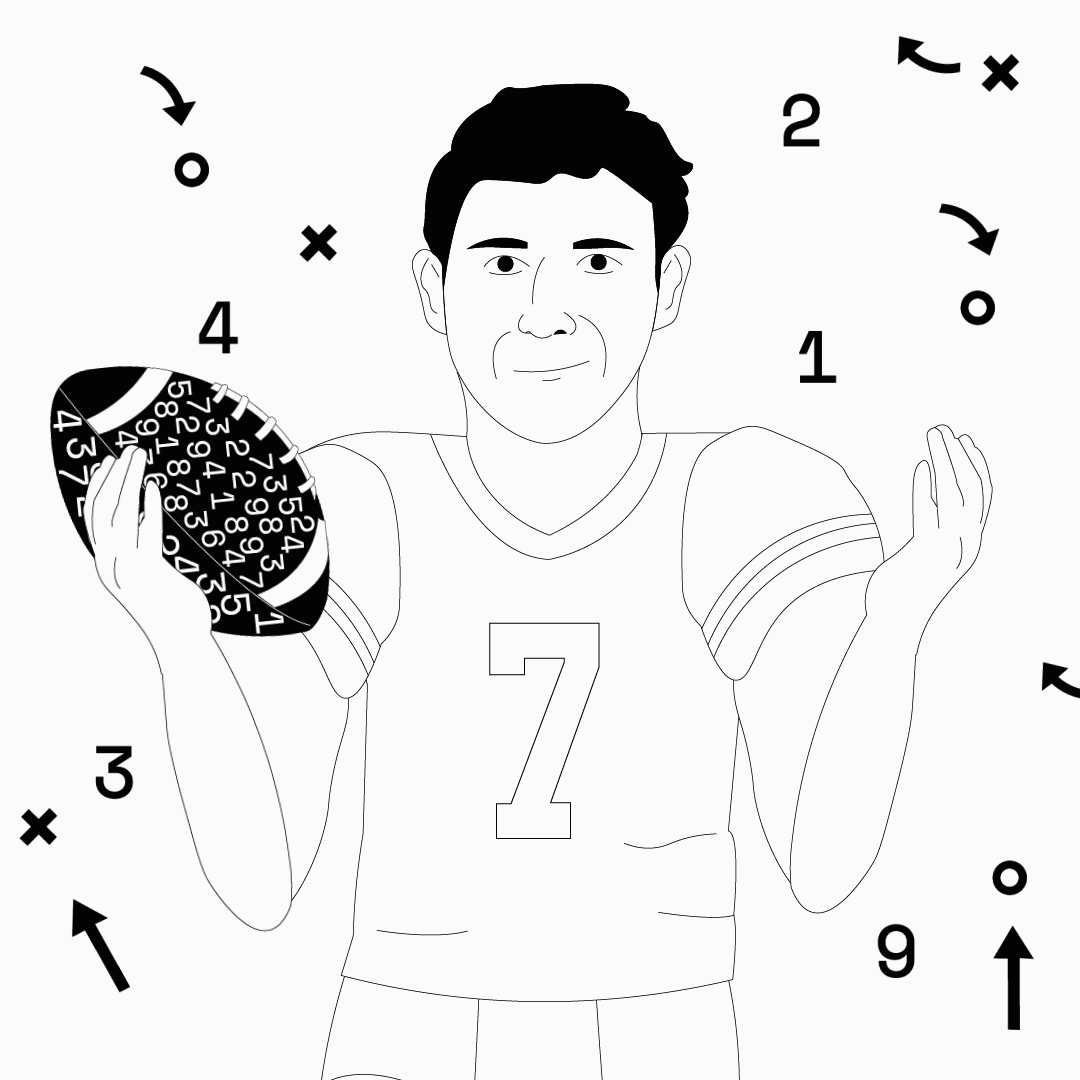
Everyone’s in the Middle, w/ Former NFL QB Hugh Millen
Former NFL Quarterback and Data Nerd
03.09.21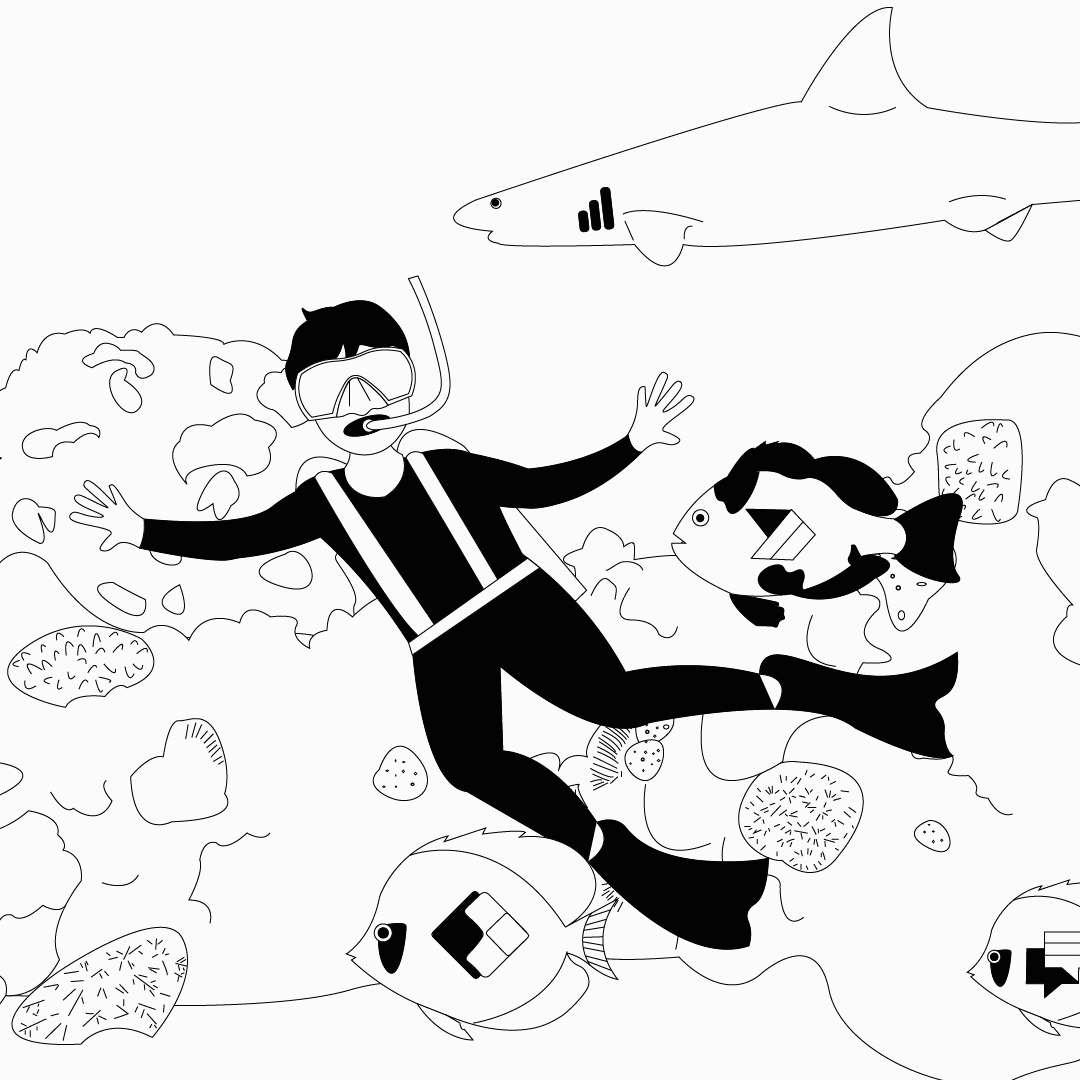
Marine Biology Leads to Power Platforming, w/ Chuck Sterling
Senior Program Manager, Microsoft
02.23.21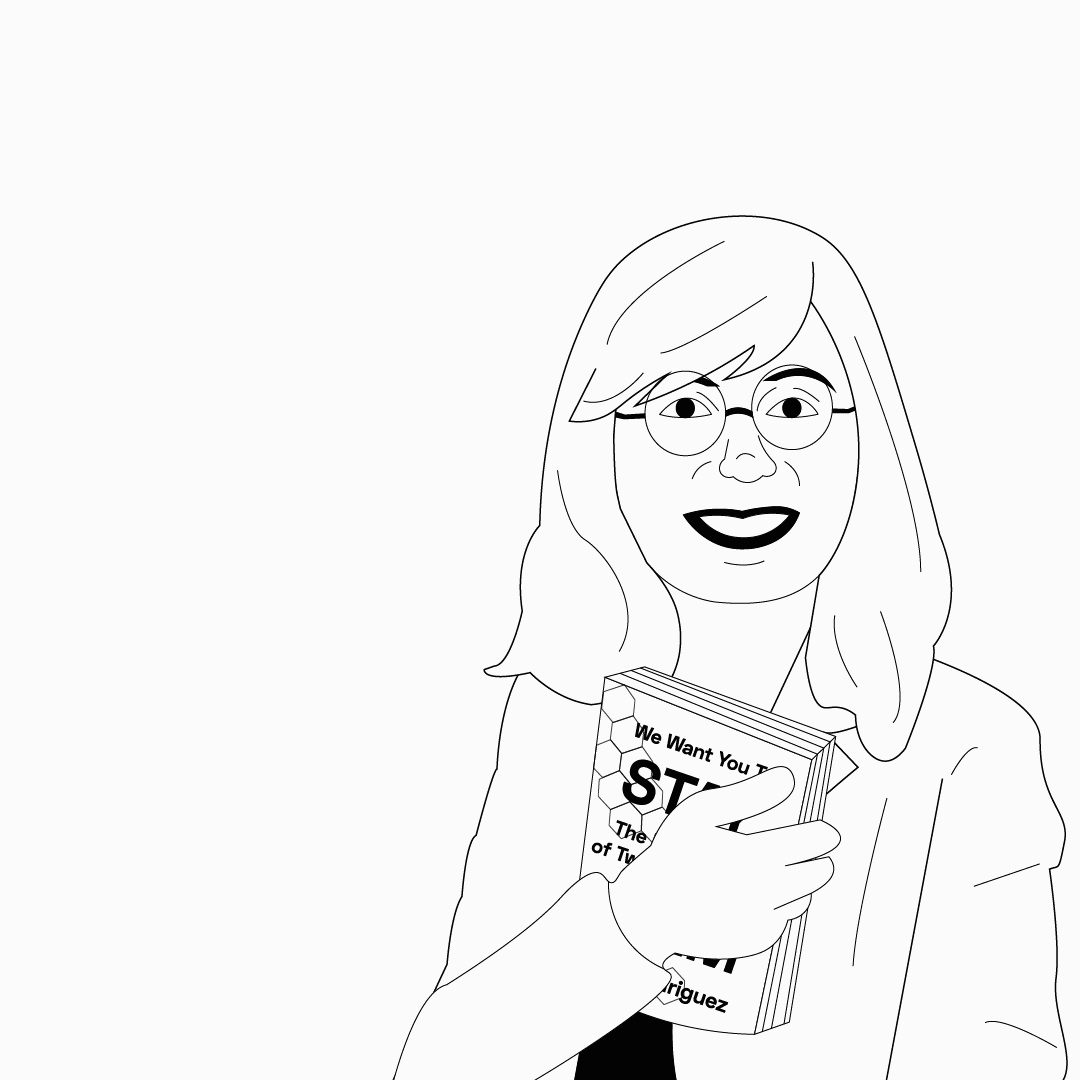
Data Chefs Can Come From Anywhere, w/ Gartner’s Lori Rodriguez
Vice President Executive Programs at Gartner
01.26.21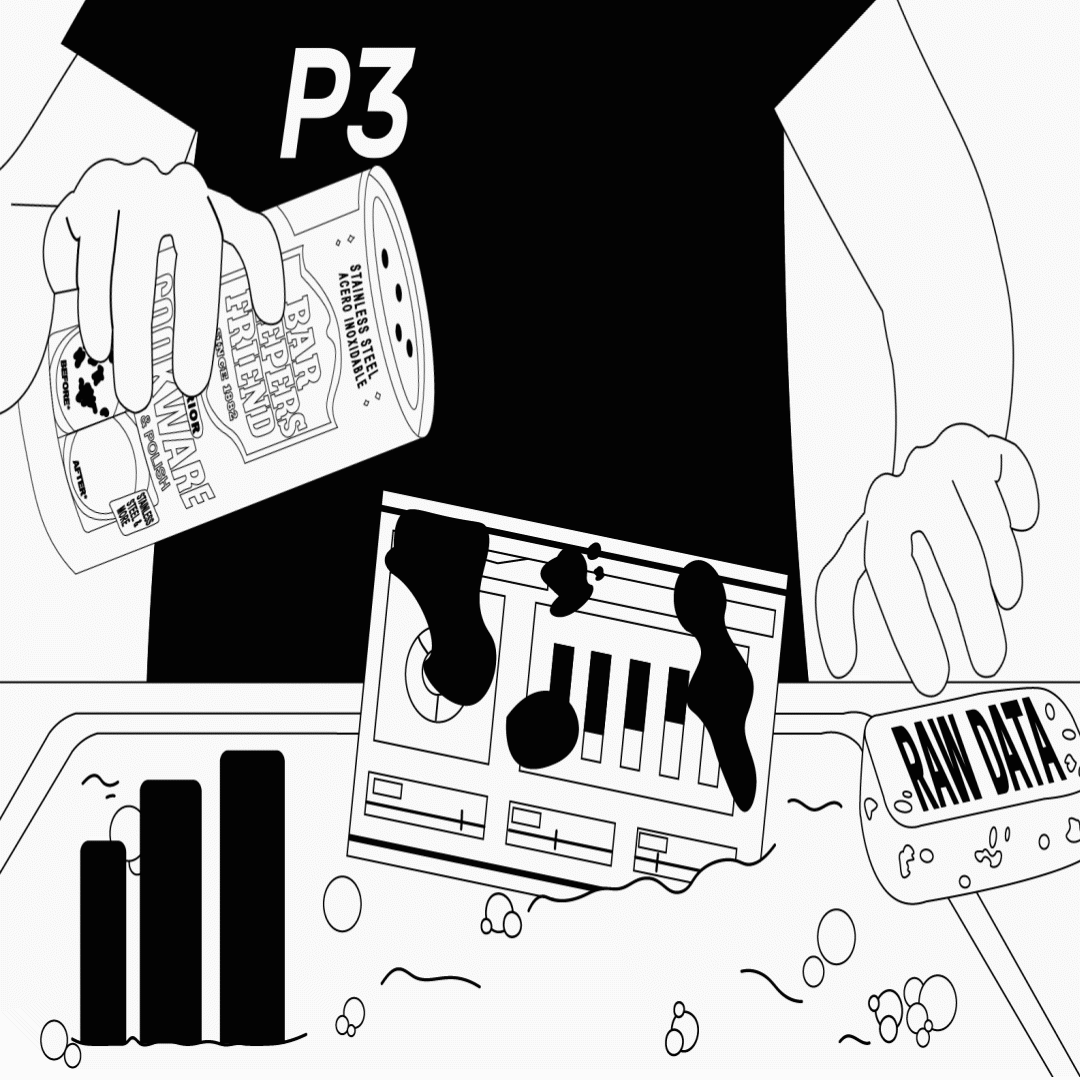
Scrubbing Data & Beating COVID, w/ Matt Selig of Bar Keepers Friend
Exec. Vice President, Chief Financial Officer & Chief Human Resources Officer at SerVaas Labs
01.05.21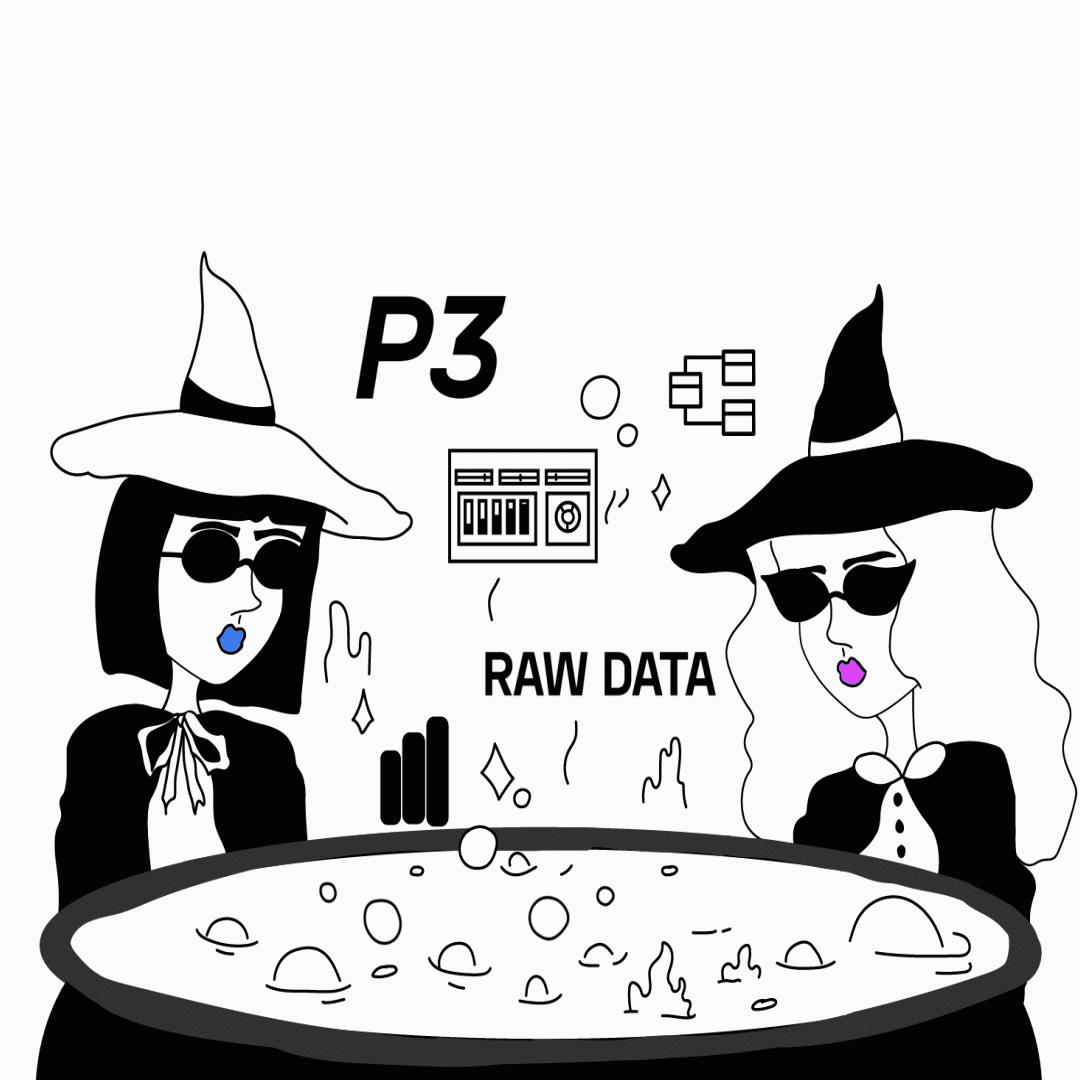
The Data Witches, w/ Shannon Lindsay & Stephanie Bruno
Shannon Lindsay-Microsoft Certified Data and Analytics Professional &
Stephanie Bruno-Associate Director of Informatics at the Elizabeth Glaser Pediatric AIDS Foundation (EGPAF)
12.22.20
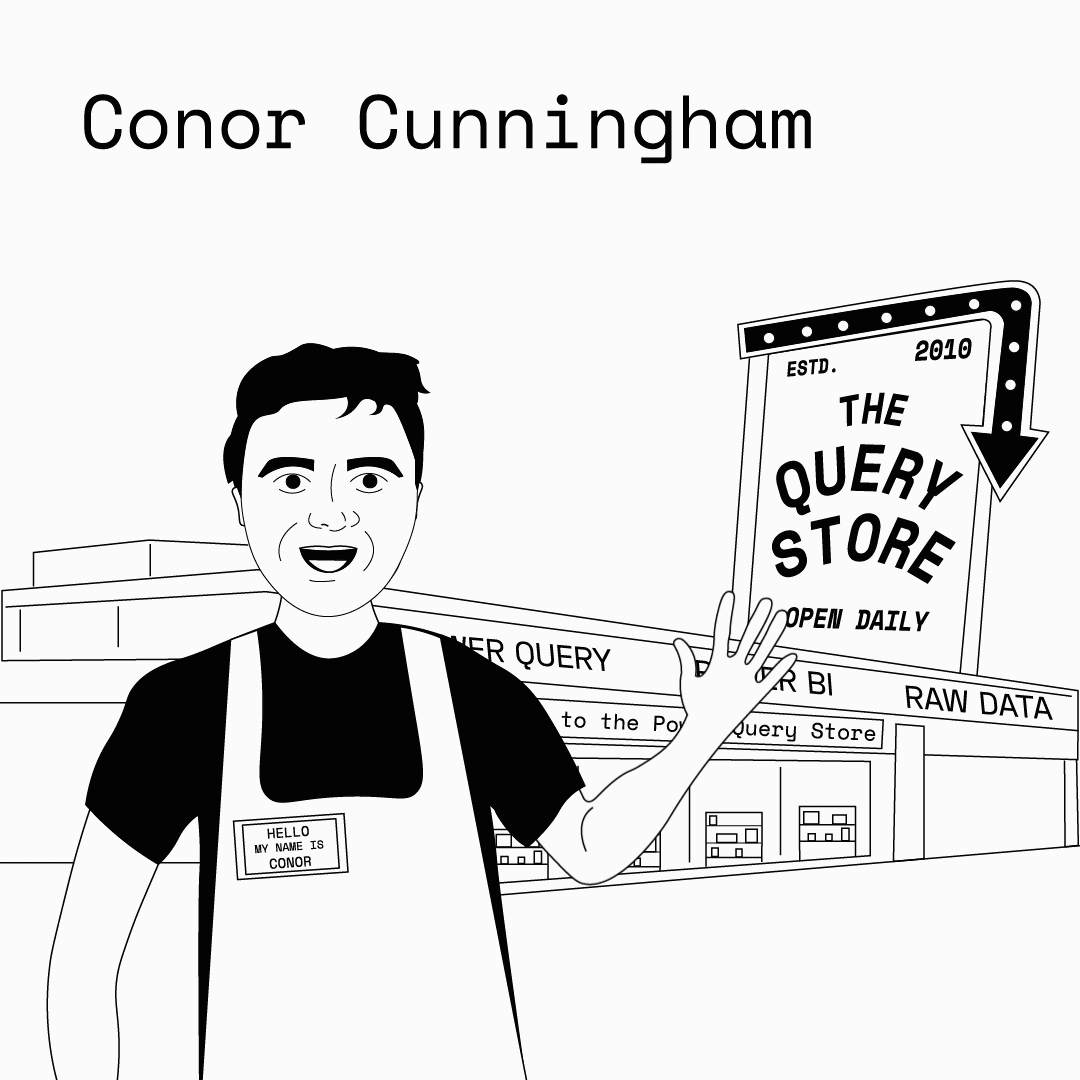
The Query Store’s Open for Biz, w/ Microsoft’s Conor Cunningham
Partner Software Architect, SQL Server Engine-Microsoft
12.15.20One of the World’s Most Amazing Humans, w/ Matt Allington
Power BI Consultant, Trainer, & Microsoft MVP
12.01.20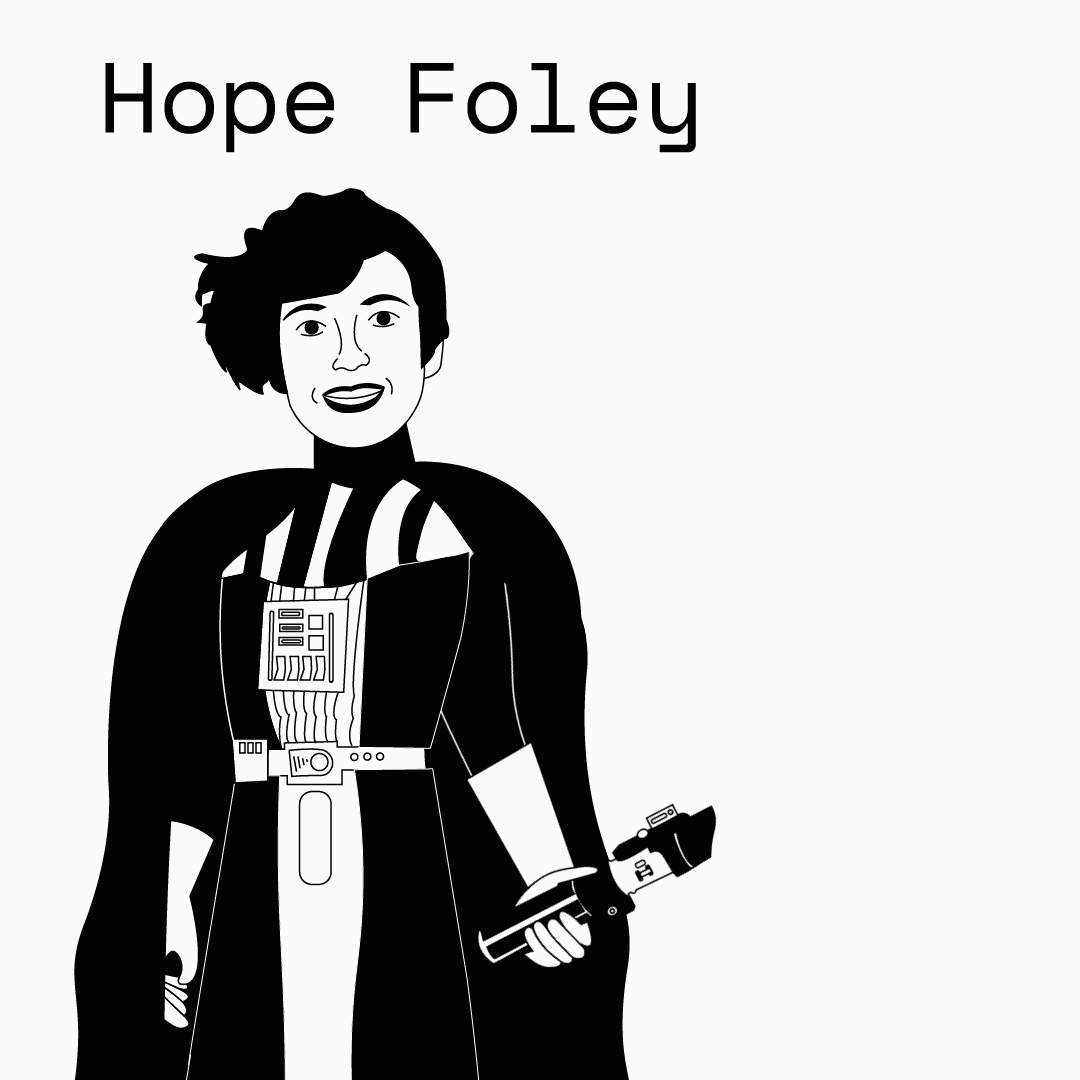