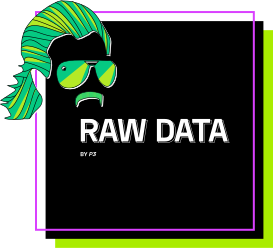
Raw Data By P3 Adaptive
A Framework for Spotting Legitimate AI Use Cases
Listen Now:Navigating the world of AI can sometimes feel like you’re trying to solve a puzzle with half the pieces missing. That’s where, in this episode, Rob and Justin come in, offering a helping hand with their down-to-earth framework designed to uncover the real-world benefits of AI for businesses. They’ll guide you through the practical journey of assessing roles, tasks, and workflows to spotlight where AI can genuinely lend a hand. Their approach is all about making the concept of AI more approachable, helping you differentiate the practical uses from the buzzword-filled fantasies.
In this enlightening episode, they cover essential ground, from understanding the implications of decisions made without full information to distinguishing tasks that could really benefit from AI’s touch, like optimizing gas delivery routes. Plus, they introduce the “AI sniff test” to help identify processes that are ripe for an AI upgrade. This detailed exploration is aimed at giving you the clarity to spot AI opportunities that are custom-fit for your business’s unique needs, whether you’re just dipping your toes into AI waters or looking to dive deeper.
Rob and Justin are essentially extending an invitation to stop wondering “What if?” about AI and start exploring “What can be?” Their friendly guidance demystifies the process, making AI seem less like a distant dream and more like a tangible tool within your reach. For anyone curious about integrating AI into their business but unsure where to start, this episode promises to be an eye-opener, turning curiosity into actionable insight.
And, as always, if you enjoyed this episode, be sure to leave us a review on your favorite podcast platform to help other users find us and hit subscribe for new content delivered weekly.
Framework for Spotting Legitimate AI Use Cases PDF
Rob (00:00): Hello, friends. I wanted to start this week with a heartfelt thank you and a shout-out to Katherine Alexander, who posted on LinkedIn this weekend rating us as one of her three favorite Power BI podcasts. Thank you very much, Katherine.
(00:15): Public recommendations like that are so kind. I really want more interaction with the listeners out there. I want us to hear what's on your mind more often than what we do. I want to hear your questions. I want to hear your feedback. Even a quick little LinkedIn post like that is super helpful to us and we really appreciate it.
(00:33): Okay, transitioning from that, as a top three Power BI podcast, last week, I talked about how our Power BI episodes in the numbers tend to outperform our recent AI episodes, but not by much. We're talking about AI again this week.
(00:47): You know, the thing we try to do most on this show is to help people navigate the space. Navigate it in terms of business value, navigate it in terms of business impact. And navigation is never more difficult nor more important than when you don't have a map.
(01:01): AI is the most frontier-ish thing going on in our world of data these days. It's not the only thing, and it's not even the first thing. We've talked about that at length at this point, that the best way to get ready for AI is to get good and get good value, get organized with Power BI.
(01:21): But just like 15 years ago when I was first piecing together my own picture of how this Power BI revolution was going to change and disrupt the world, one of the most valuable things we can be doing right now for our listeners, the community and our clients, is to be making continual sense of the seemingly rapidly evolving space of AI.
(01:42): Now, in last week's episode, we kind of gave what would, I guess you could think of as the bare case for AI. The fact that the hallucination problem is not just the detail, it's actually like the Achilles heel of everything.
(01:54): This week we kind of tackled the opposite side of the coin, the bull case for AI. When is it useful? How do you spot the places where it actually might help your business? In that talk I gave a couple of weeks ago, I kind of rolled out like, let's call it the beta or the V0 of an evolving methodology, a flow chart, a paint-by-numbers type of approach to help you spot cases in your business where AI can legitimately be of assistance.
(02:23): Now, I didn't have a chance to kind of test out this workflow on Justin before I went and gave the talk. So in today's episode, I walk him through that framework and I get his thoughts on it.
(02:34): Now, of course, a workflow and a framework like this is something that lends itself to a visual really well. Like we want to have like a downloadable version of this at some point. We're early in this process. We don't have like a polished download yet. We'll take the excerpts out of my slide deck that cover this framework. We'll turn them into a PDF and we'll put them in the show notes.
(02:53): Okay. All that aside, let's get into it.
Announcer (02:57): Ladies and gentlemen, may I have your attention, please.
(03:01): This is the Raw Data by P3 Adaptive podcast with your host, Rob Collie and your co-host, Justin Mannhardt. Find out what the experts at P3 Adaptive can do for your business. Just go to p3adaptive.com. Raw Data by P3 Adaptive is data with the human element.
Rob (03:27): All right, Justin. Welcome back. I think we had decided or agreed that it was still my turn to pick a topic and I thought we'd left something sort of uncovered. Like I want to circle back to the presentation that I gave here locally in Indy a couple of weeks back about AI and specifically there, in the second half of the presentation, I wanted to break this down to use cases for people.
(03:52): And so just so happened that Microsoft put a Facebook ad in front of me a week before I gave this talk saying, "Download the AI Use Cases for Business Leaders E-book."
Justin (04:03): They found you.
Rob (04:04): Yeah, and I think this is great, AI Use Cases for Business Leaders, because you've got to break it down. We did an episode on AI is actually many things, and I downloaded this Use Case e-book and it wasn't very good.
Justin (04:20): Did you have to give up your email address for this e-book, Rob?
Rob (04:22): Of course. But don't worry. I told them the size of my organization, and so they're not spamming me. If I had been a 5,000-seat organization, I'd be getting spam. But they're like, "Oh, you're mid-market. You're not worthy of our attention. Cannot extract sufficient revenue from you."
(04:42): This was one of the examples that I shared with you that led to you chortling and led to the epiphany about hallucination. Let me read this to you.
(04:50): "AI-powered content generation can serve as an invaluable resource to speed up communication." At the end of that sentence, I'm thinking, yeah, the thing that summarizes meetings and stuff, right?
Justin (05:02): Right.
Rob (05:03): That's a good use case. I like where this is going, Justin. All right, not so much.
(05:08): "Consider quarterly reports and market trend analyses for a financial firm. AI natural language processing can collate complex data from different sources, transforming it into comprehensive, yet accessible reports, market summaries and personalized investment strategies."
(05:25): No, that came from a Microsoft e-book. It shows you how easy it is for overhyped bullshit to leak into marketing materials. This is in a paragraph that started out talking about communication. And very next breath, it's saying, "Yeah, just feed all kinds of unstructured information into it" and then produce quarterly reports that you're going to, like, I assume use with investors? "Produce personalized investment strategies." You're going to invest your retirement according to this... Just how? How did this get into something official?
Justin (06:03): Well, AI wrote it.
Rob (06:05): Maybe. And there's your consensus, right? It's a really good point. That e-book, if it makes it into the training corpus for these LLMs, we're just going to get more and more of this and it's going to gain this critical mass that everyone's going to think this is happening and it's just not happening for them.
(06:25): No. It spits out an image, and I asked mid-journey to make me an image of a star schema.
Justin (06:31): You did?
Rob (06:32): A very specific concept in Power BI, right?
Justin (06:36): Oh, I remember this, yeah.
Rob (06:37): And it spits out a picture of a constellation in the shape of a star, L-O-L. Like, I know that's wrong.
Justin (06:44): I think that's why any conceivable usability on those things, it's like, oh, you have to like really constrain it's input to answer that question. Otherwise, it's like, "Okay, what's a star? What's a schema? What does Rob mean? Here we go."
Rob (07:00): Here's the place where I'm trying to develop a useful framework. Mostly what we've done to this point is talk about all the ways in which AI is hollow. It's a paper tiger in a lot of ways.
(07:13): But hiding underneath all of this smoke, there is a little bit of legitimate fire, and you want to be able to take advantage of that because there are legitimate use cases.
(07:23): So I put together a visual, and I think what we'll do is we'll link this visual in the show notes, sort of a four-step visual process for coming up with actual use cases for your business.
Justin (07:34): Actual AI use cases for your actual business. I dig it.
Rob (07:39): Or at least candidates.
Justin (07:40): Okay.
Rob (07:41): So first of all, and this is a place where the Microsoft eBook is another instance where I think it fails us, it starts with a list of different types of technologies. Don't do that. Don't start with a list of technologies as your outline for how to approach this.
(07:57): Instead, start with a list of people stuff, roles, people in your business, tasks and workflows. That can be a long list.
Justin (08:08): Oh, yeah, even in a simple business.
Rob (08:12): But if you sort of like preview the whole workflow that I'm proposing here, it makes it easier at the beginning to sort of start preemptively whittling the list down a bit.
(08:22): So the next step is for those lists of roles, people, workflows, et cetera, evaluate the business impact. You can think about this from an offensive and a defensive lens for a moment. The defensive lens is like, okay, look for workflows that consume a lot of time, money, energy, or produce a lot of errors, workflows with flaws, flaws that impact the business and evaluate what the business impact would be if those drawbacks, delays, expenses, losses were greatly reduced, getting a size on the impact, right? Because if something got better and it makes no difference, well, who cares?
(09:06): But then the harder thing is evaluating those same workflows for maybe you're not seeing that they could be a lot better. The flaws might not be obvious, but only because you haven't imagined it being completely different.
(09:19): And then I have this thing called running the AI sniff test.
Justin (09:23): Tell me more.
Rob (09:24): So I have four sort of sniff test examples of like when something might smell like an AI problem. The first two are repetitive and blurry versus smart and tedious. Repetitive and blurry, key examples of that would be customer service.
Justin (09:40): Sure. Like, "Hey, I have a question about my cable bill."
Rob (09:43): That's right. If something's repetitive and non-blurry, meaning like every single time the inputs and outputs are clear, generally speaking, we've solved that with software already. That's what old software did. That's if this, then that type of stuff, right?
Justin (10:00): Yeah.
Rob (10:01): You wouldn't like want your Ferrari dealership. You want that to be a human always, right? High volume customer service. There's a long tail for sure, but most requests are not unique. Most complaints, most questions, they are not unique.
Justin (10:14): It's a massive 80/20 thing going on there.
Rob (10:17): Yes, like probably a 99:1. Yeah.
(10:22): What is unique is the way people describe when they call in, they've got problem XYZ, but they're going to describe it differently than the last 20 people who had problem XYZ. And so because of that blurriness in the way that it's described, we've traditionally put a human shock absorber in the system. The human answers the phone, listens to it, translates it into problem XYZ, and then essentially turns around and presses button XYZ on the back computer.
(10:54): Like that last part doesn't really require a human, but translating it into press XYZ. You know, these LLM's ability to understand the question as it's formulated in many different ways has been a tremendous breakthrough. So this is an example.
(11:12): Another example I had of this was bookkeeping, right? An expense comes in.
Justin (11:16): Oh, where should I code this?
Rob (11:17): There's going to be an exceptional case where something falls off the end or it doesn't have a confident assignment. Even in these cases, the hallucination problem is non-zero risk.
Justin (11:30): That's right.
Rob (11:31): It might confidently be misassigning expenses to the wrong accounts, the wrong codes for a long time before you discover it.
Justin (11:39): It's just classical probability impact, matrix style thinking on those kinds of things. Like what's the probability it's going to hallucinate a hundred percent? How often and what's the impact when it does? It's not going to ruin us, but if it might, you think about it.
Rob (11:55): Yep. So this is what I mean by it's repetitive. In essence, the same task is being performed over and over again, but there's some blur in the definition of the assignment that makes it AIE.
Justin (12:12): Repetitive and blurry. All right, I'm into that one.
Rob (12:14): Now, by contrast, they're smart and tedious. So smart and tedious is much less repetitive, meaning like every instance of this workflow is essentially unique.
(12:27): The first example I gave, because sort of tongue in cheek, making slide decks is an example of smart and tedious. And the new topic, it's a new topic each time, you're communicating with human beings and you're trying to produce clarity. Like you can't just be a good slide maker. You have to know your subject and you have to know how to communicate. You have to think about the audience and all that kind of stuff. There's going to be a human being involved in this process. A process like that is going to have a human being involved in it centrally until or unless we get Skynet-level AI that just, literally, replaces humanity.
Justin (13:04): Yeah, there's a sidebar on that, too.
Rob (13:07): But there is a tremendous amount of tedium interwoven throughout this, producing the graphics and lining them up with each other, getting the fonts right, getting the layout right.
(13:19): This is where I ended up on LinkedIn the other day, just absolutely blasting Copilot in PowerPoint as completely and utterly worthless. It wouldn't even change the fonts.
(13:32): Now I've got the designer that'll just automatically generate cool potential designs for my slides, but I can't tune it. I can't give it any iterative feedback, yeah, and I can't get it to adopt a certain style that I've already defined on one slide and say, "Now keep using that. Apply it to the others."
(13:50): Like these are the things that I should be doing, right? Building Power BI models, intelligently constructed Power BI models and writing the formulas, maybe I can get some help with the formulas, more on that later, but this is a very, very intelligent process.
Justin (14:04): With lots of tedium.
Rob (14:05): Yes. Oh my God, laying out the reports.
Justin (14:08): Yeah, it's interesting. It's different than hallucination. It's like a recall problem. Like even in the chat, I've got some things going with GPT-4. I'm like "Hey, remember what we were talking about before? Can you bring that back?" It will never bring it back verbatim.
Rob (14:23): Right.
Justin (14:23): And then even if you prompt it, like, "No, verbatim, exactly what you said before," when you say like you get a style and a deck you like, or a theme you want to follow or a brand guide.
Rob (14:33): I think that's coming. Like that has to be coming. That is a solvable, solvable, solvable, solvable problem, and it's actually really frustrating that it hasn't been solved better than it has or seemingly even attempted at this point. It shows you what the hype cycle is like these days.
Justin (14:49): Oh, yeah.
Rob (14:49): The PR value of saying that we have Copilot in Office was so great that they gave me a feature that's outright insulting. PowerPoint Copilot is insulting. It makes me angry.
Justin (15:06): Well, don't use it. Give your license back to Kellan.
Rob (15:09): Yeah, it's expensive, right? It's an expensive feature that is worse than Google. It's worse than OpenAI and it won't make any changes to my deck. Okay, shows you where we're at on the hype cycle, but that one's going to be solved, and that's a perfect example of smart and tedious.
(15:26): I think writing marketing copy is another example of smart and tedious. You have to decide what the core themes are, what the touch points, the emotional touch points that you want to lean into, but having it spit out the text for the whole webpage once you've established that and then go back and fine-tuning is a great use, right?
Justin (15:43): Yeah. Here's what I'm thinking. Give me five ideas, like riffing with GPT. I actually like that quite a bit. Like I never go, "Ah, cut, copy paste. That's what I'm..." It's always like, "Well, I like where this is going." Now I, the human, am wired into this and I can go off finishing my business plan or whatever I'm working on.
(16:03): But honestly, Rob, my favorite use case is "Get me off the blank page. I want to do this and here's like the three things that are important to me. I need to crystallize this in like a clear abstract. Like give me five options."
Rob (16:15): So I talked about underinformed decisions and actions, so decisions and actions that are made or taken with incomplete data. Remember, we could have started talking about this as this is the machine learning tech that produces numbers, but I think that's the wrong way to think about it.
Justin (16:33): Yeah, it's different.
Rob (16:34): We're trying to think about it from the business lens angle. We have been looking at workflows and decisions and actions. You don't have all the information.
(16:43): Now, some caution here. Incomplete often looks like complete.
Justin (16:50): Say more.
Rob (16:51): Well, of course, we had all the information. We had our sales data, we had our customer breakdown, we had this, we had that. You can't see the things you didn't have. For example, if we fast-forward in time, you didn't know that 25% of your customers were about to defect.
(17:08): So sometimes this is easier to do looking backwards. So it's like you look back and say, "Okay, this workflow, when we were doing it a year ago, problems came along that we didn't know about." And then sort of like if you apply that forward, it's like, "Okay, so what are we maybe missing today?" Even though it's incomplete, you think it's complete because you've given it all the information that you would think that you could get.
Justin (17:32): Yeah. The correlation doesn't equal causation-type thing, right? Data's always just been part of the story.
Rob (17:39): Yeah.
Justin (17:39): It's just a part of the decision-making process.
Rob (17:42): The same way that incomplete data often looks like complete. The actions that you're taking without all the data often look a lot like we're not doing anything, meaning, like it's an absence of action. Because if you'd knew, you'd be doing something different.
(17:58): One example we have of this was the predictive data models we were working with a gasoline retailer, delivery algorithm, like deciding which stations to deliver how much to on an ongoing basis. There's only a couple of very simple strategies. One of them is just like go around, touch every station.
Justin (18:16): Right, yeah.
Rob (18:17): Or wait for them to complain that they're getting low, you know? It's not a long list of strategies that you come up with.
Justin (18:23): Pretty sophisticated.
Rob (18:26): If you're constantly visiting the same station and filling its tanks that were only 15% empty, then that trip was an inefficient trip.
Justin (18:36): That's right.
Rob (18:37): I mean, ideally, you arrive there to refill the tanks at the last possible moment before they run out. So a machine learning model that's adapting sort of in real time to usage trends and like it's learning from previous years and areas of the country and all that kind of stuff, it's a completely different workflow. You change the workflow. That's an example that I think was very effective.
Justin (19:01): The practical applications from machine learning are getting lost in the noise, in the AI noise.
Rob (19:08): Well, you've got all this image generation and video generation.
Justin (19:11): And that's just like a great example of efficiency in the workload or the work process and efficiency in the result, hopefully, right?
(19:19): But it's even like financial forecasting is another example where machine learning has been really effective for a lot of companies. And they might not be getting dramatically more accurate forecasts, but they're just spending less time and energy to get the same result. And then they're spending more time in this sort of under-informed decisions and action, like they're getting the assembly of the predictions more quickly. That's underappreciated right now, and I worry we'll forget.
Rob (19:46): Workflows without all the information. They've got multiple different tech paths that might help them. So we're talking about generating predictions of who's going to run out of gas next. We're talking about predictions of which customers are likely to change their behavior in positive or negative ways.
Justin (20:05): What's the price of a barrel of oil going to be tomorrow? All this kind of stuff.
Rob (20:09): So those are machine learning ones, but then there's also search and summary tools that help your employees get access to information that you already have.
Justin (20:18): Yeah, like the natural language query. Yeah.
Rob (20:21): Sitting down at an internal chatbot and saying, "Hey, I'm pretty sure I've seen charts somewhere that show this trend" and having it find and point me to the right Power BI report, for example. Maybe it was a PDF internally somewhere. That's huge. Because if you can't find it in a timely manner, or maybe you don't know it exists. Maybe you go, "Do we have anything that shows me this?" Right?
Justin (20:49): Right.
Rob (20:50): And in either case, if an answer to that question isn't readily available, that decision or action that they need to take has a deadline and they're going to do it without the info, right?
Justin (21:00): Right.
Rob (21:01): So internally trained LLMs, chatbots that understand the corpus of the information that's available to employees, I suppose there's a row-level security problem here, right? Like-
Justin (21:13): Right.
Rob (21:14): Are there equivalents of that? Are you aware of I've got like all these internal assets, unstructured data and otherwise, and I train up a private ChatGPT on all the information available inside my company. Are there ways to sort of blind it to information that certain employees shouldn't have access to? Are you aware of anything like that?
Justin (21:36): I don't know. But for example, like Microsoft Copilot, my understanding is it is subservient to the role access controls that already exist.
(21:48): Now there's some questions about how and when that could get superseded and that's a proceed carefully territory, but I don't believe Microsoft has sort of like left that out.
Rob (22:00): It's so tricky.
Justin (22:01): Let's say you're working on a document and it's in our folder that like only you and I have access to this folder, the first default link that it gives you, it'll say like, "Anyone at P3 Adaptive can access this file with this link." So now there's a link that exists in the record, and so you wonder like how these breadcrumbs play into this whole thing. You didn't intend to share it with the whole company. You just wanted to share it with me.
Rob (22:26): Yeah.
Justin (22:27): A lot of times those aren't like super sensitive things, but they can be. So that's a proceed with caution to your research point, I think.
Rob (22:34): Okay. So we'll put a link to the visual process, maybe even a couple of slides for identifying use cases.
Justin (22:40): That'd be great.
Rob (22:40): I'm really curious to see if this is useful to anyone, right? Like these presentations I create are forcing functions. They're also forcing functions to get feedback and improve. I'm positive this framework is one that we're going to continue to evolve and improve over time. So we can't do that without feedback so let's hear something.
Justin (22:58): Bring it on.
Rob (22:59): Well, thank you, Justin.
Speaker 2 (23:00): Thanks for listening to the Raw Data by P3 Adaptive Podcast. Let the experts at P3 Adaptive help your business. Just go to p3adaptive.com. Have a data day.
Sign up to receive email updates
Enter your name and email address below and I'll send you periodic updates about the podcast.
Other Episodes
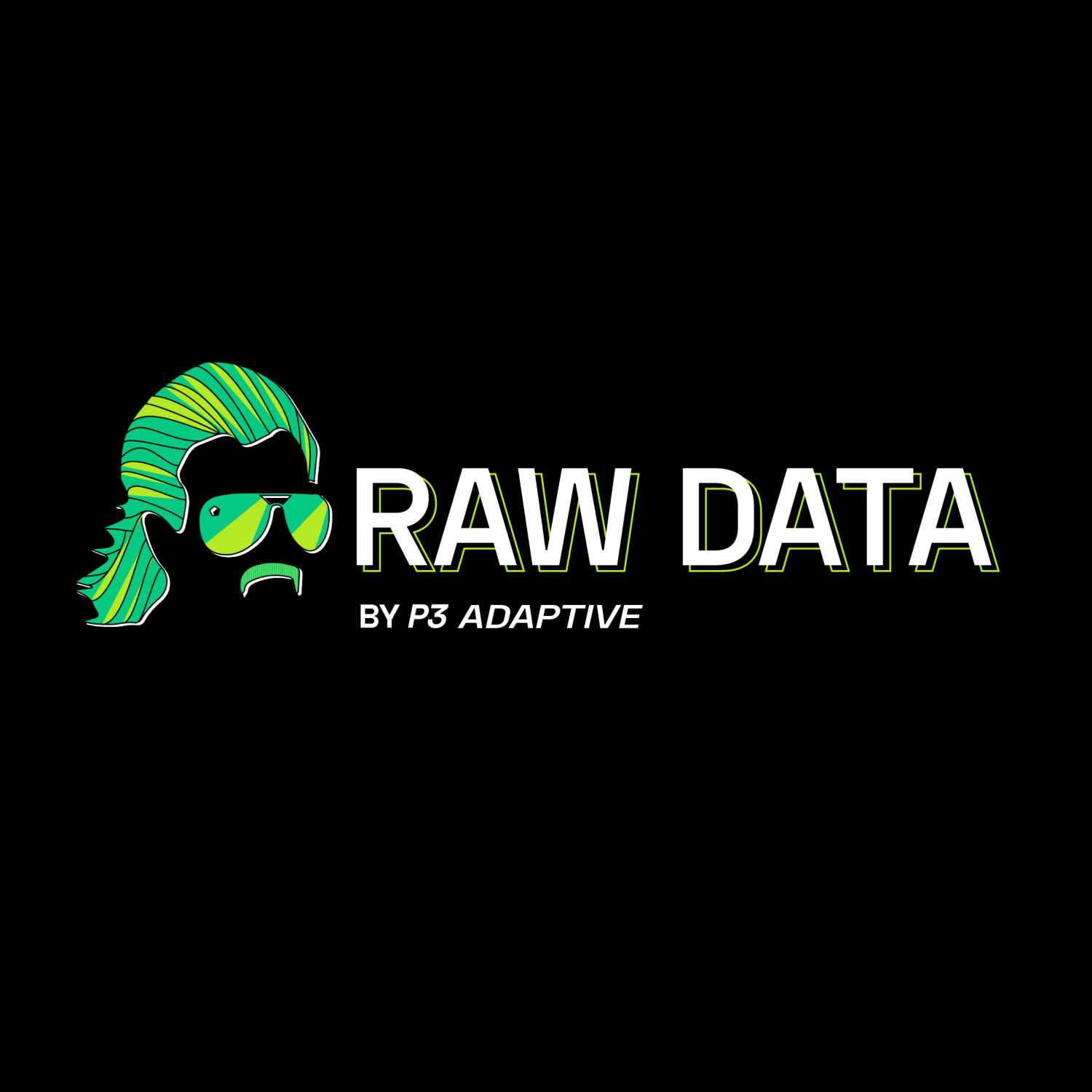
Adjacent and Between: Demystifying Digital Transformation with Power Apps and Power Automate
03.26.24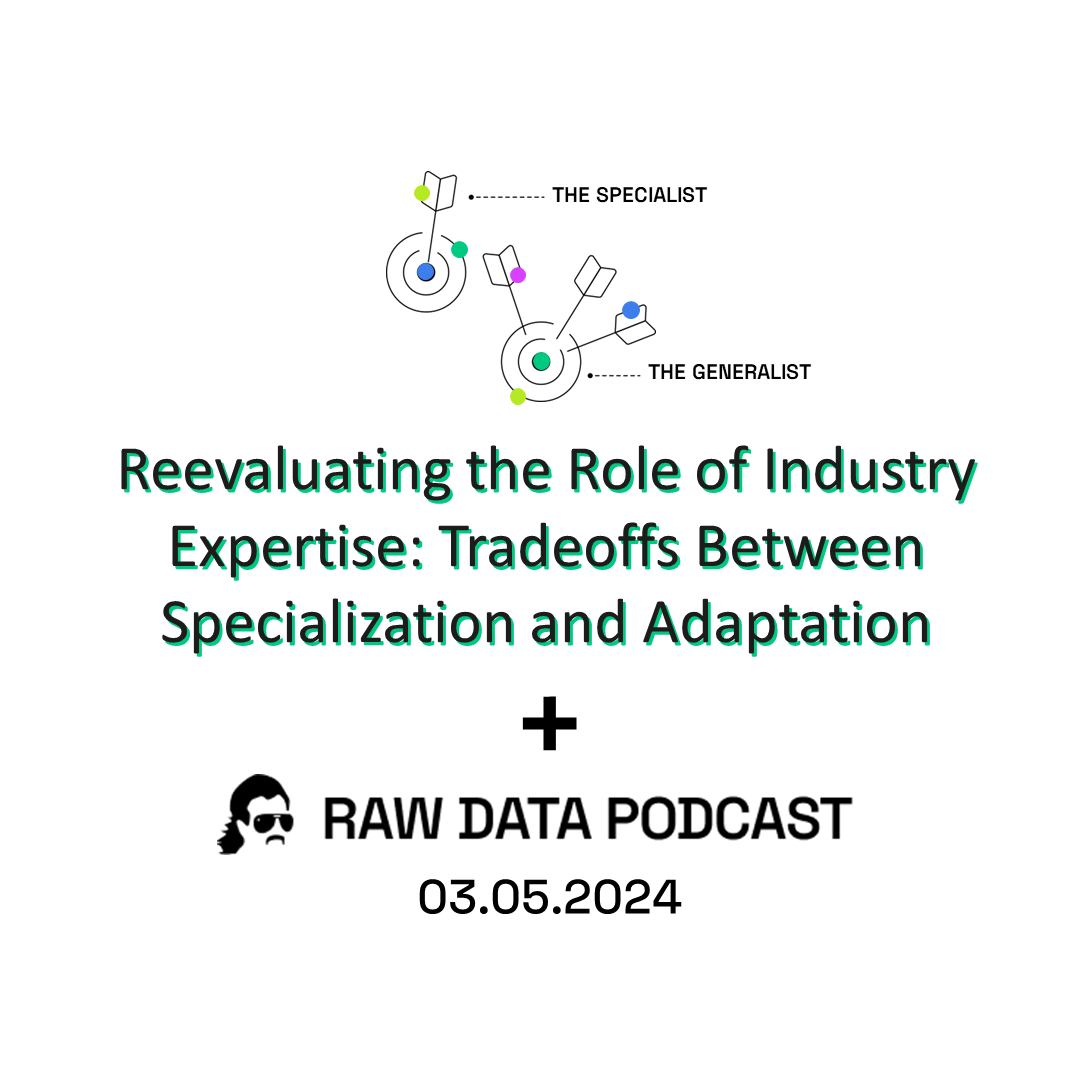
Reevaluating the Role of Industry Expertise: Tradeoffs Between Specialization and Adaptation
03.05.24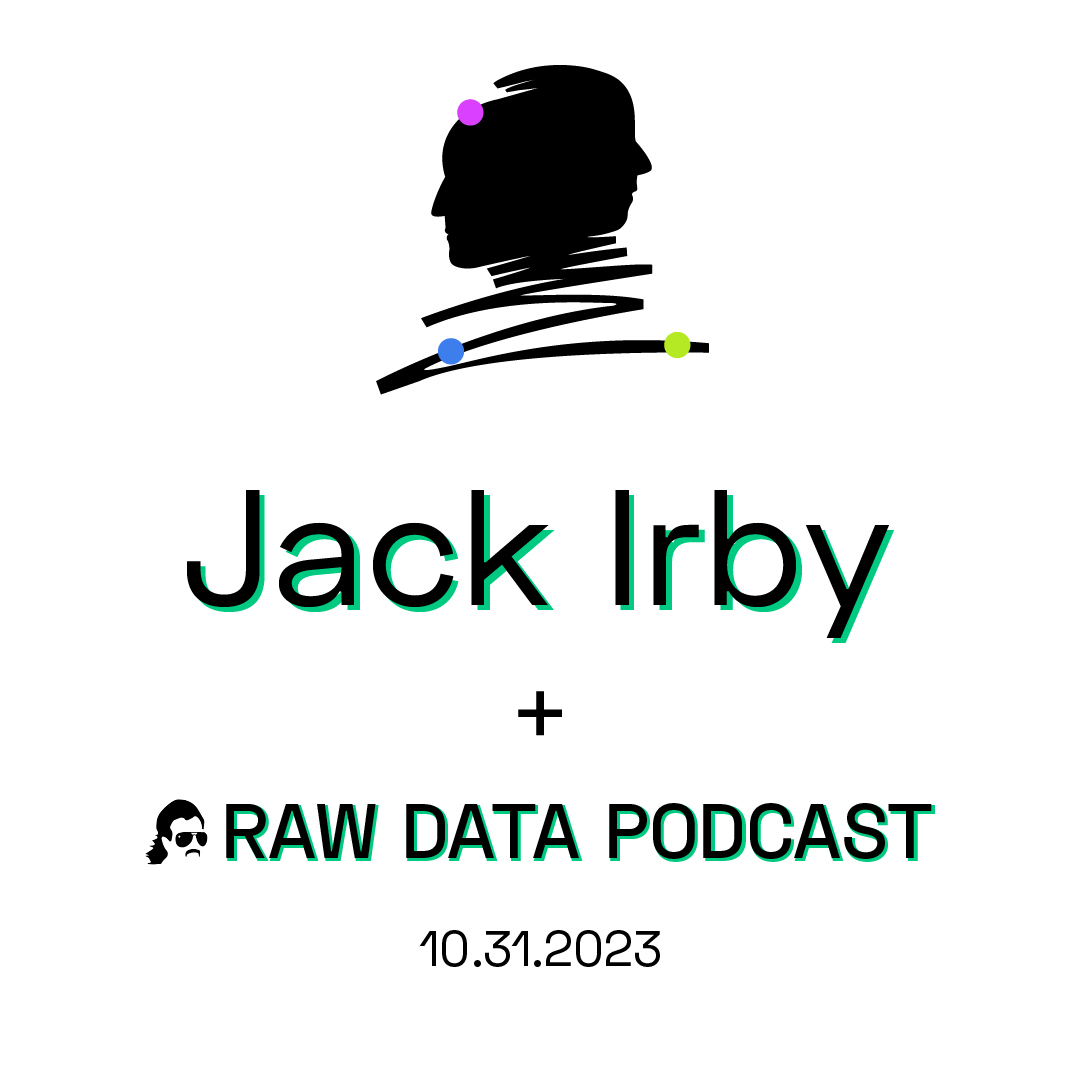
The Fruit of the Electric Grapevine: Industry-Leading Visibility on Limited Resources, w/ Jack Irby
10.31.23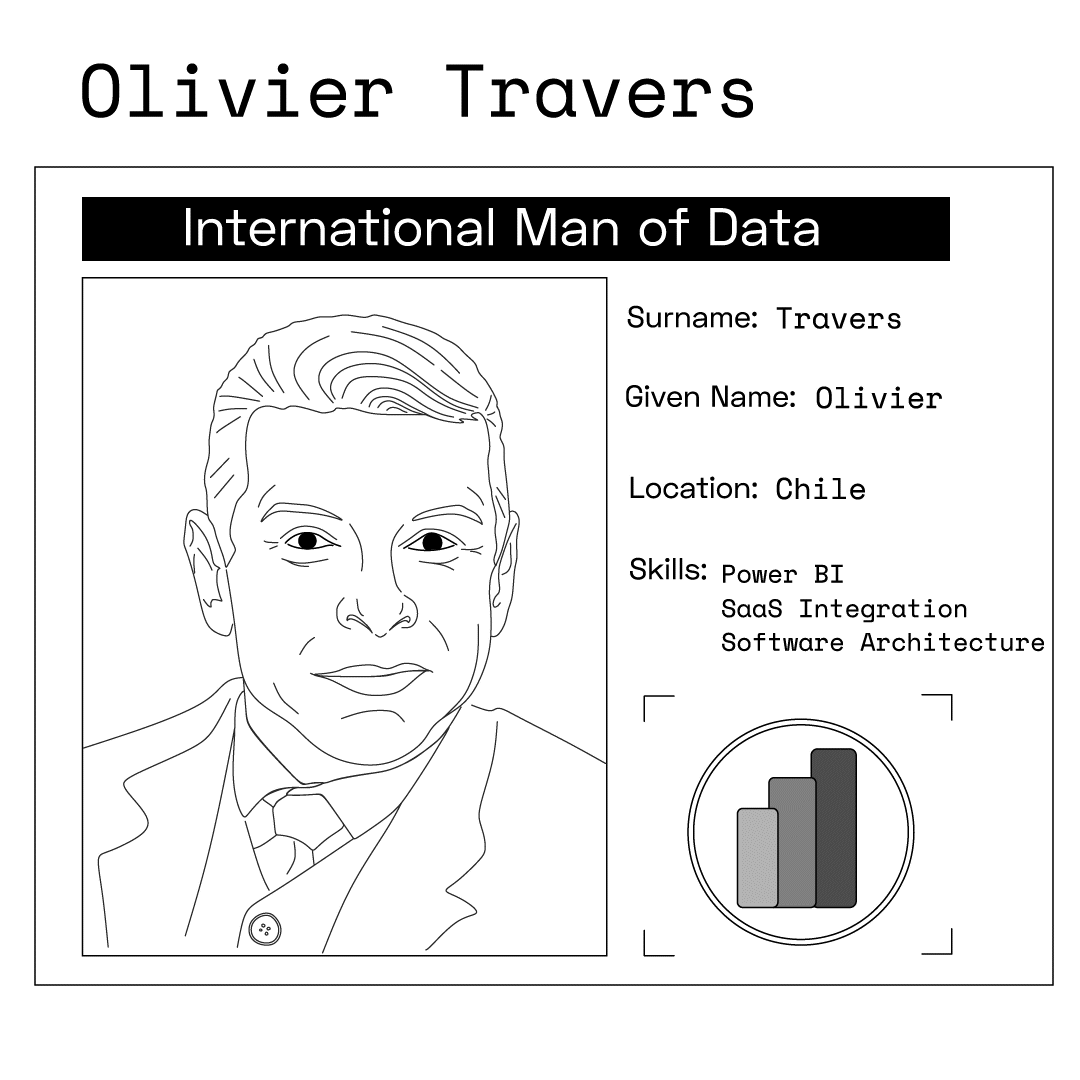
Stay Away from Guadalcanal, w/ Olivier Travers
MS Data Platform International Man Of Mystery
01.04.22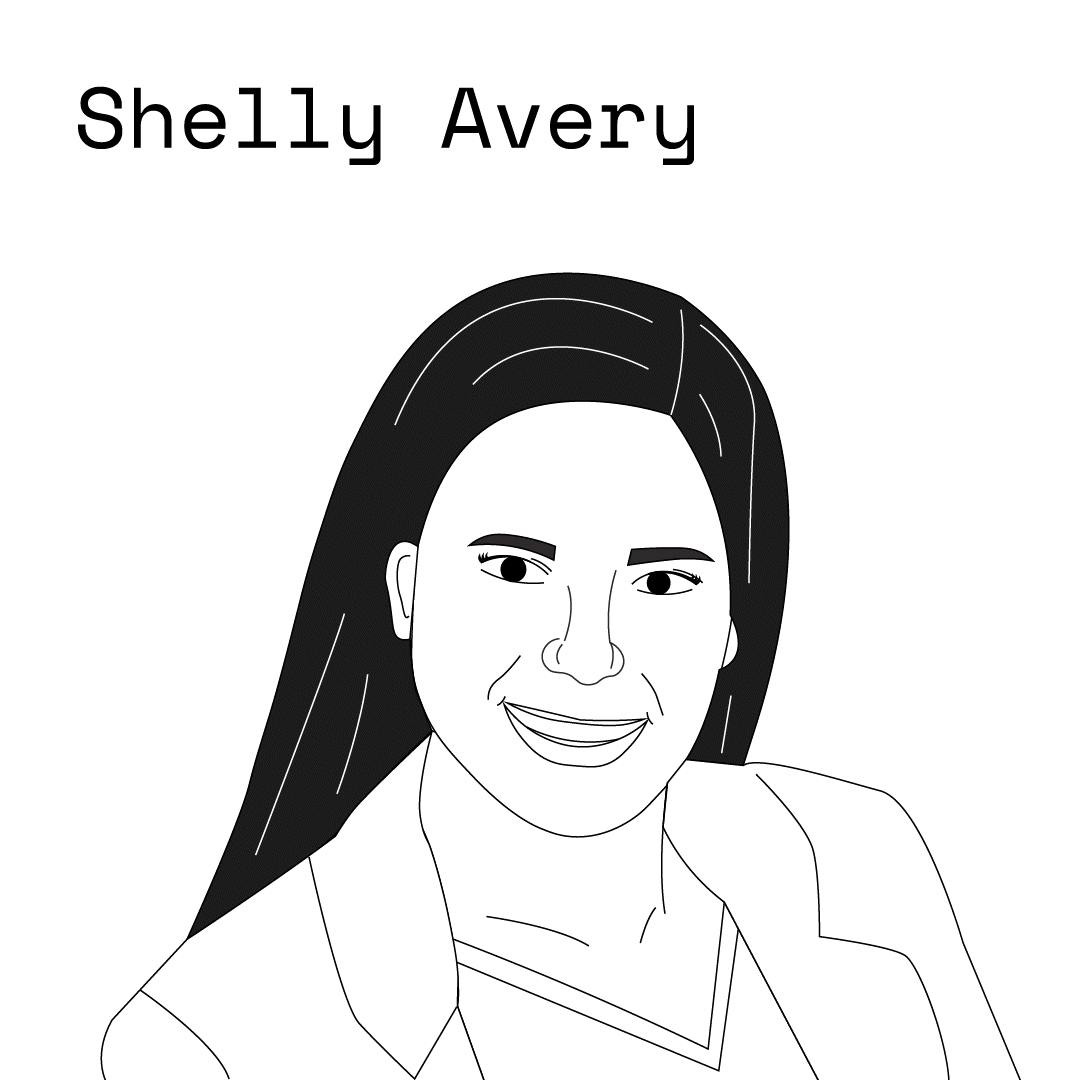
99% is the New Zero, w/ Microsoft Director Shelly Avery
Director-US Healthcare Specialist, Microsoft
09.14.21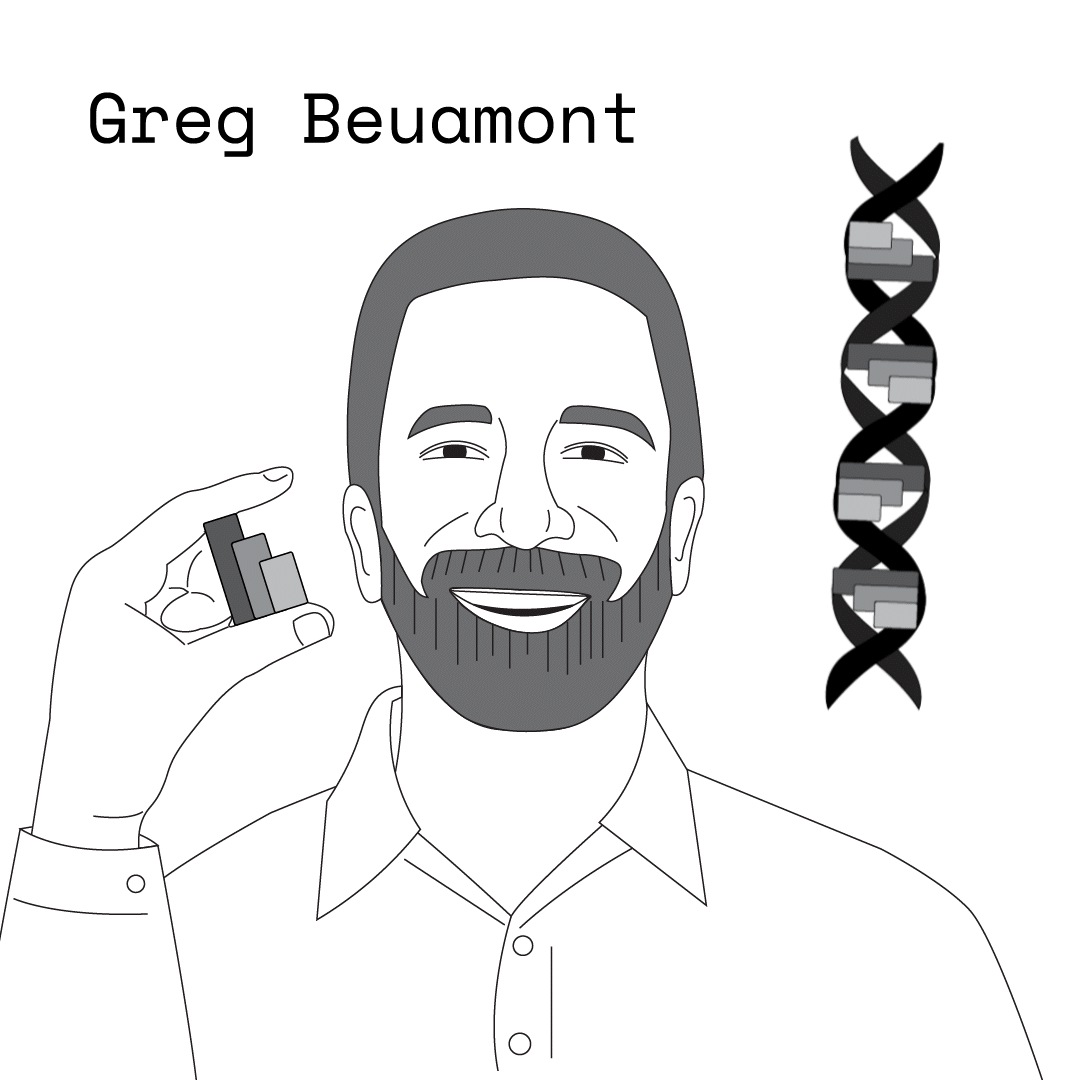
For Those About to DAX, w/ Microsoft’s Greg Beaumont
Senior Business Intelligence Specialist, Microsoft
08.17.21
Spreadsheet Celebrities & Power BI Playdates, w/ Chandoo
Hear from the Mozart of Excel Dashboards: Chandoo
08.10.21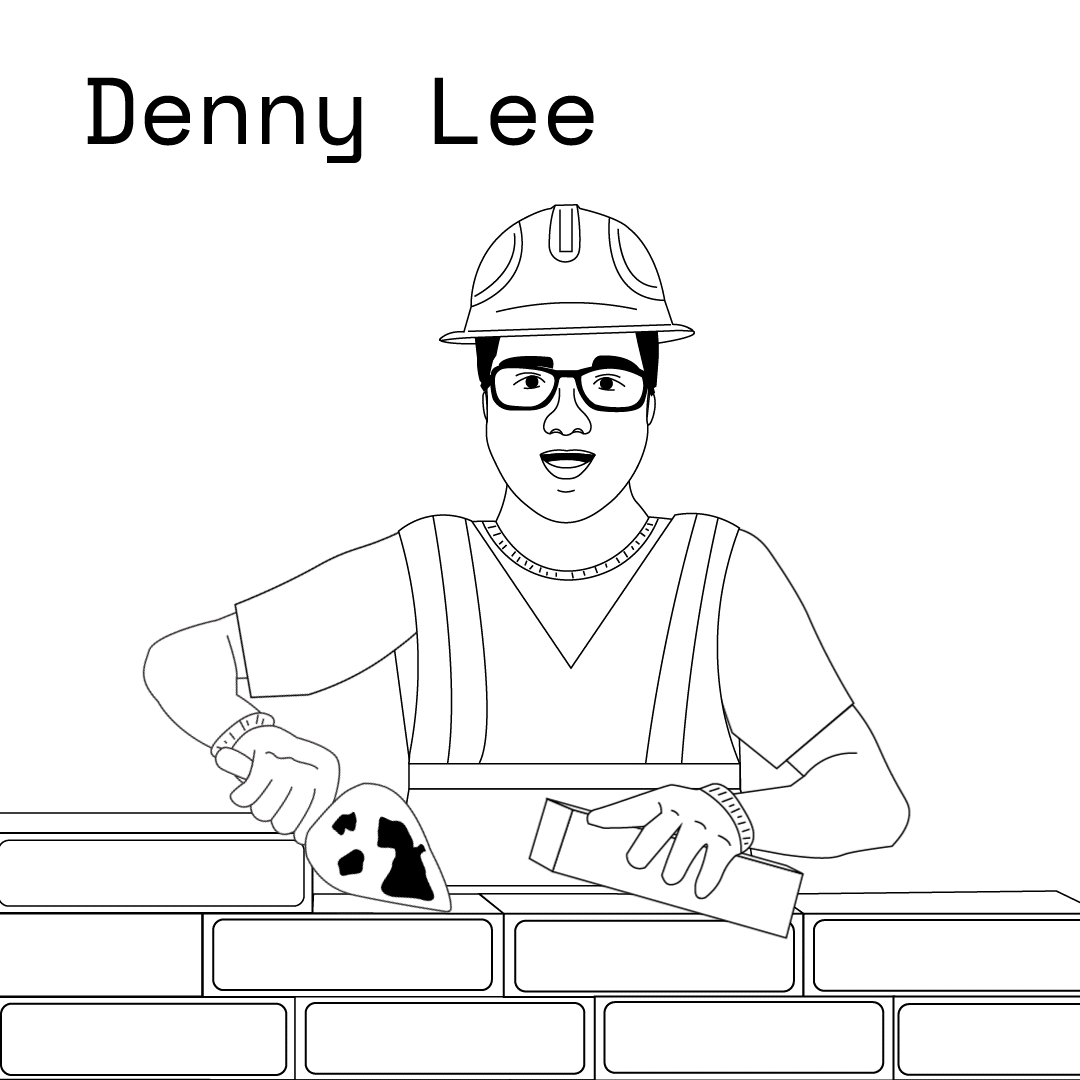
The Data Lakehouse: Brick by Brick, w/ Databricks’ Denny Lee
Sr. Staff Developer Advocate, Databricks
08.03.21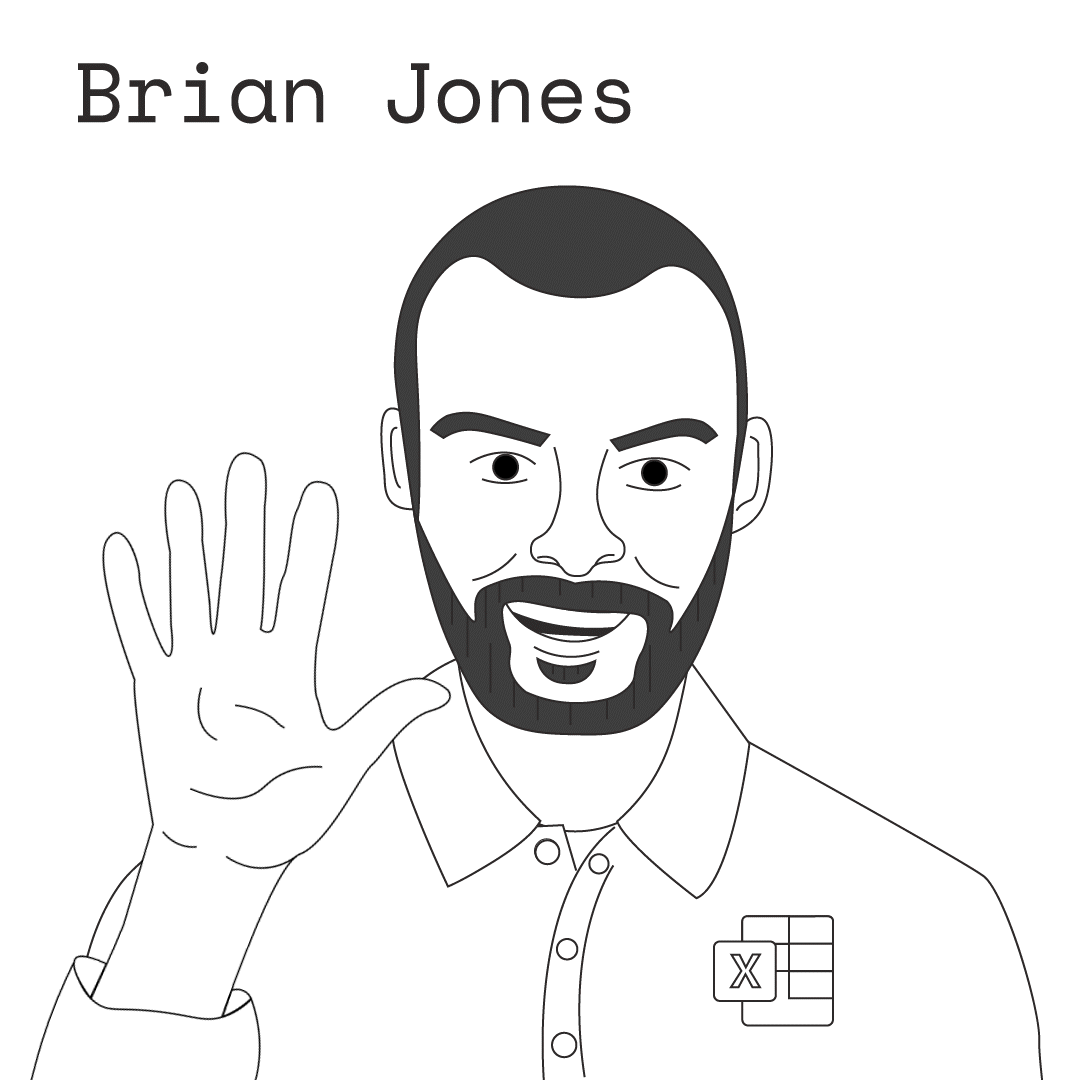
Excel Past/Present/Future, w/ Excel Head of Product Brian Jones
Head of Product, Excel-Microsoft
07.27.21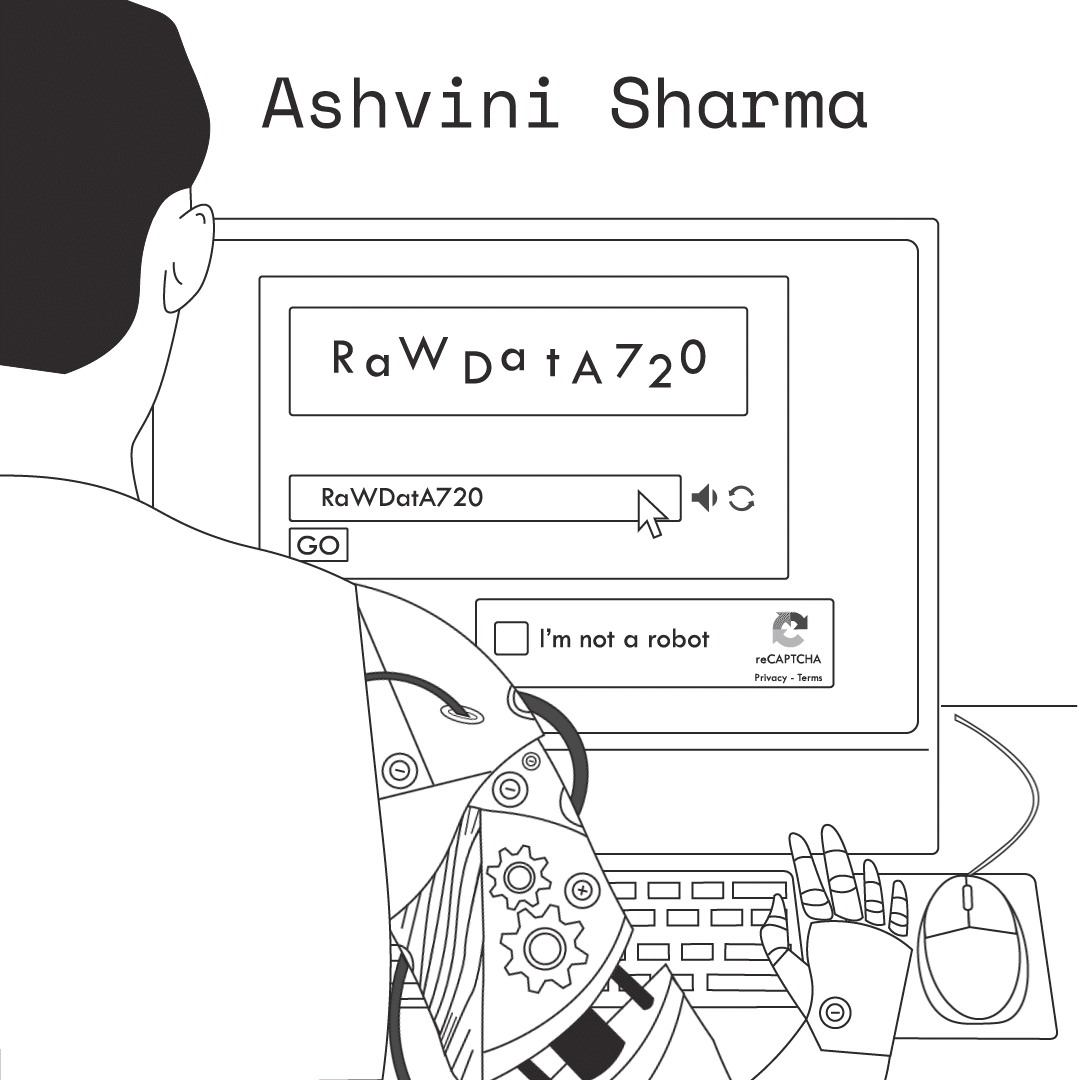
Robotic Process Automation for All, w/ Microsoft’s Ashvini Sharma
Group Program Manager-Power Automate RPA, Microsoft
07.20.21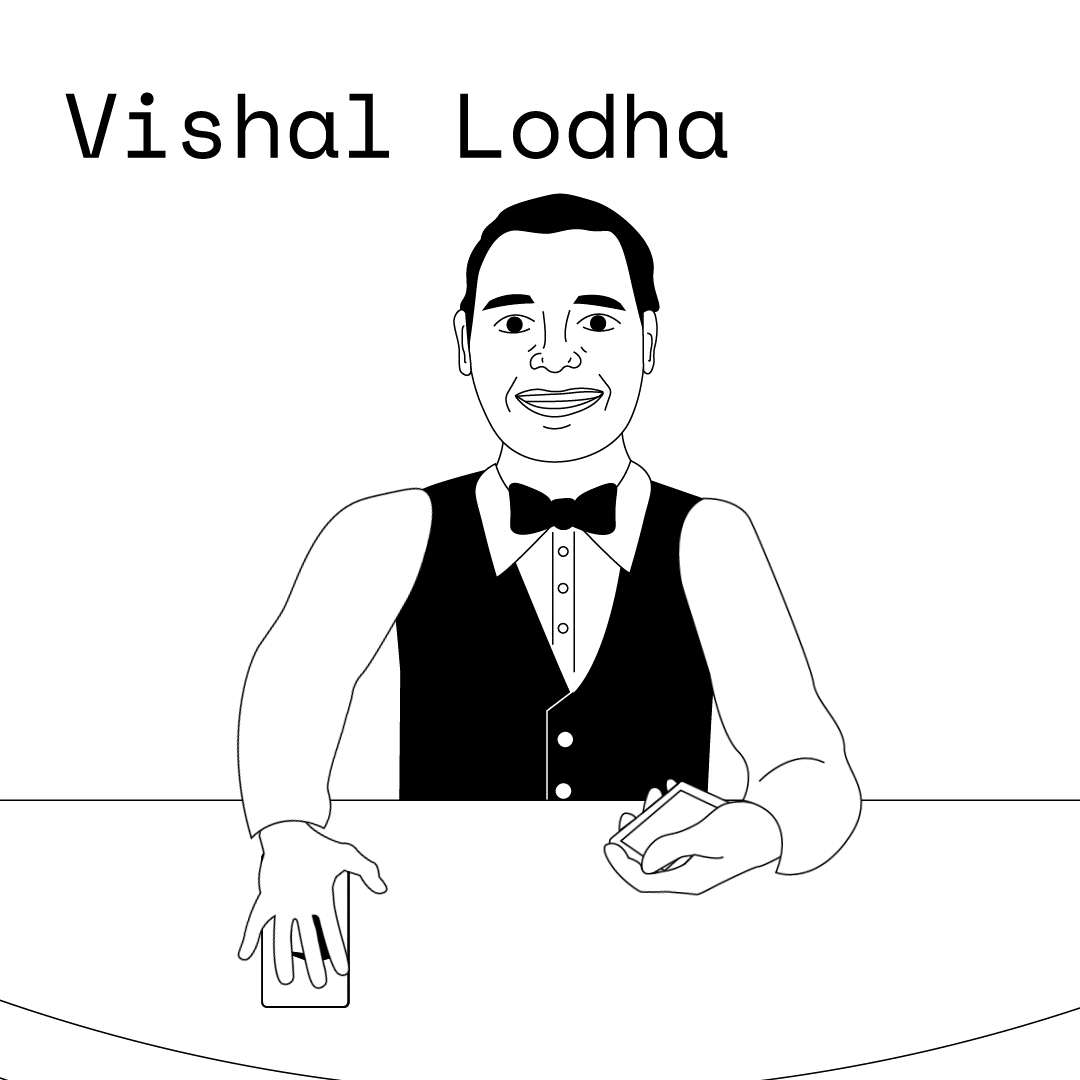
COVID Basement Racquetball, w/ Microsoft’s Vishal Lodha
Strategic Account Director, Microsoft
06.03.21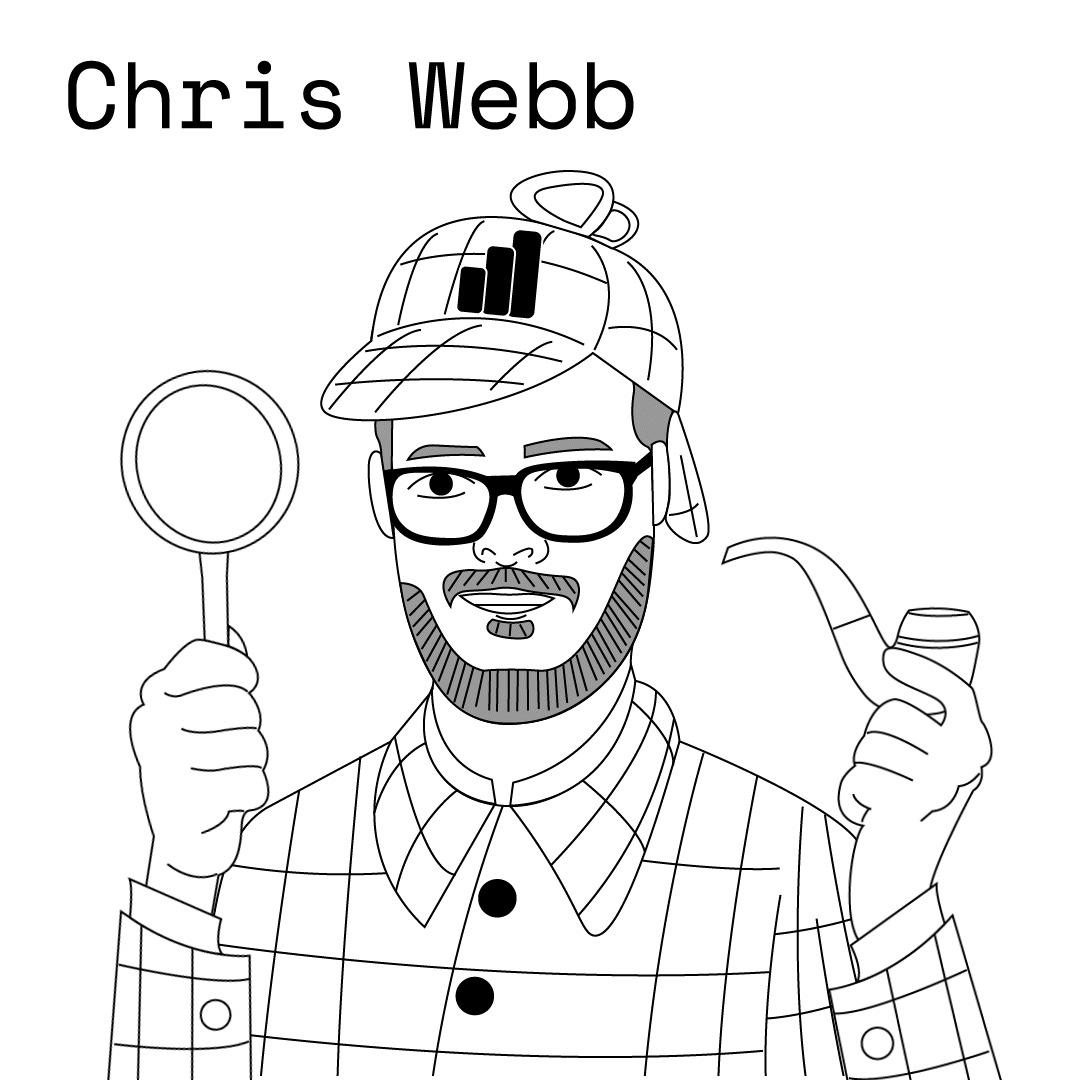
Surfing the Changing Seas of Data, w/ Microsoft’s Chris Webb
Principal Program Manager-Power BI Customer Advisory Team, Microsoft
06.01.21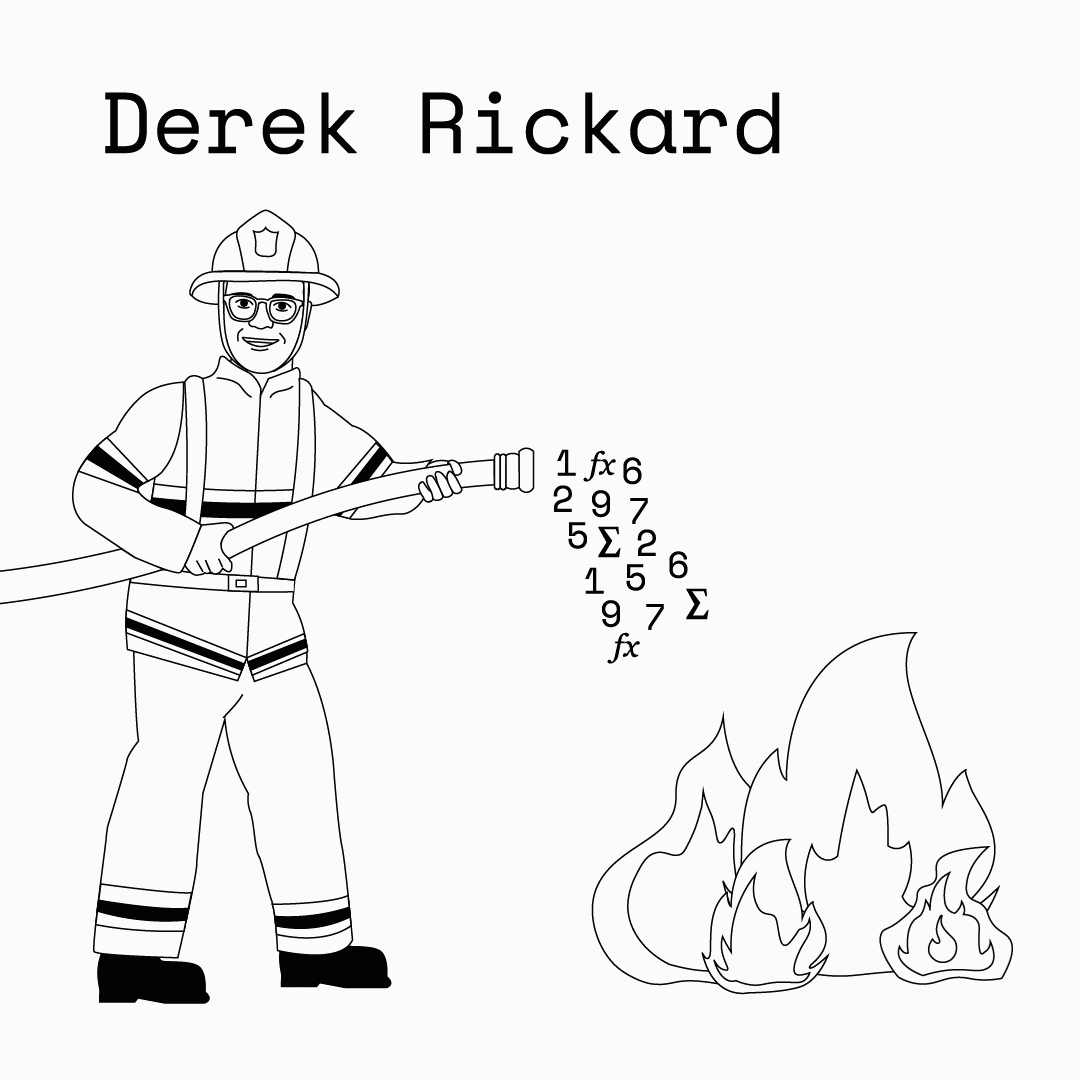
A Different Kind of Data Emergency, w/ Derek Rickard
Chief Data Officer, Emergency Reporting
05.04.21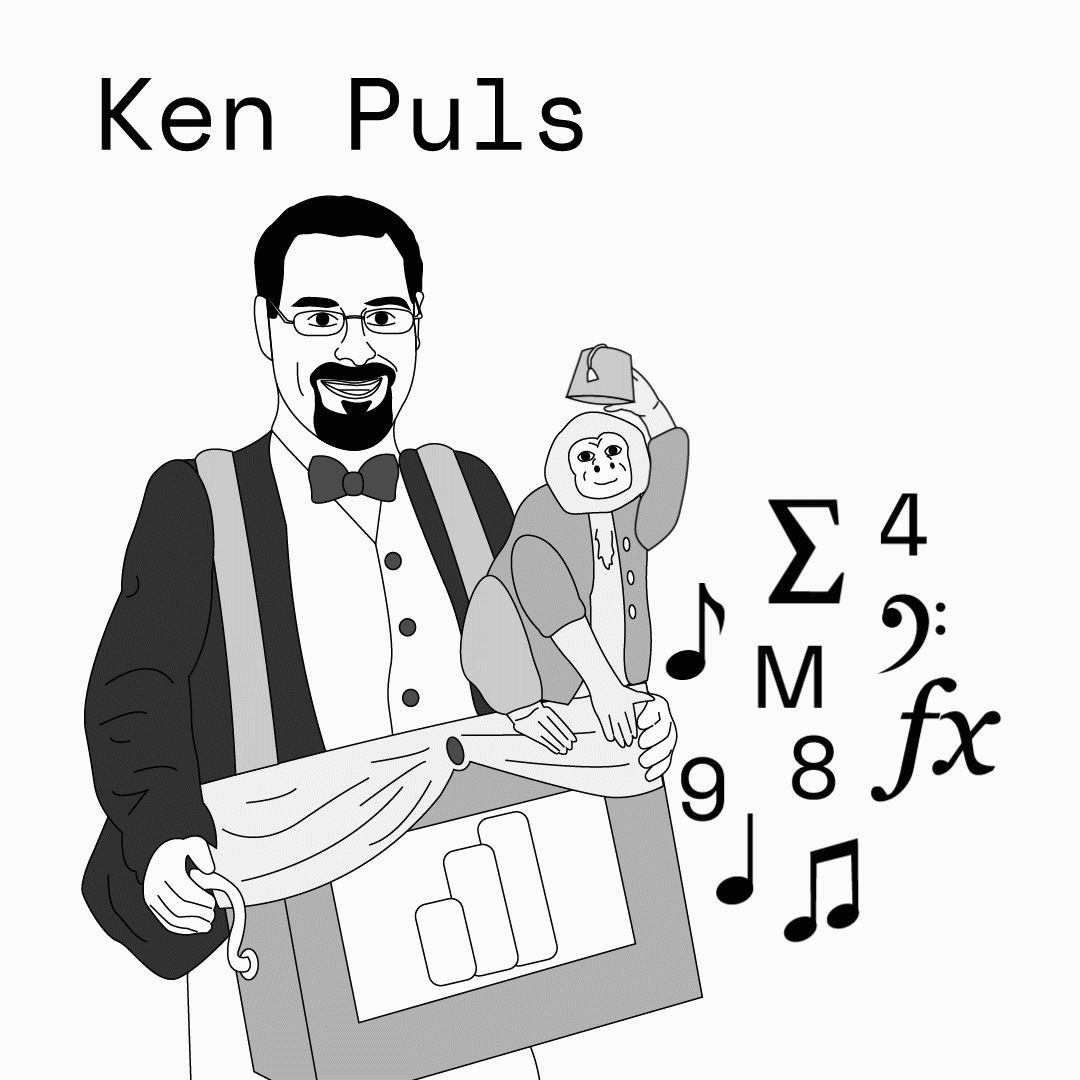
Earth’s Only Hope vs. Alien Excel Invaders, w/ Ken Puls
President and Chief Training Officer, Excelguru Consulting Inc.
04.27.21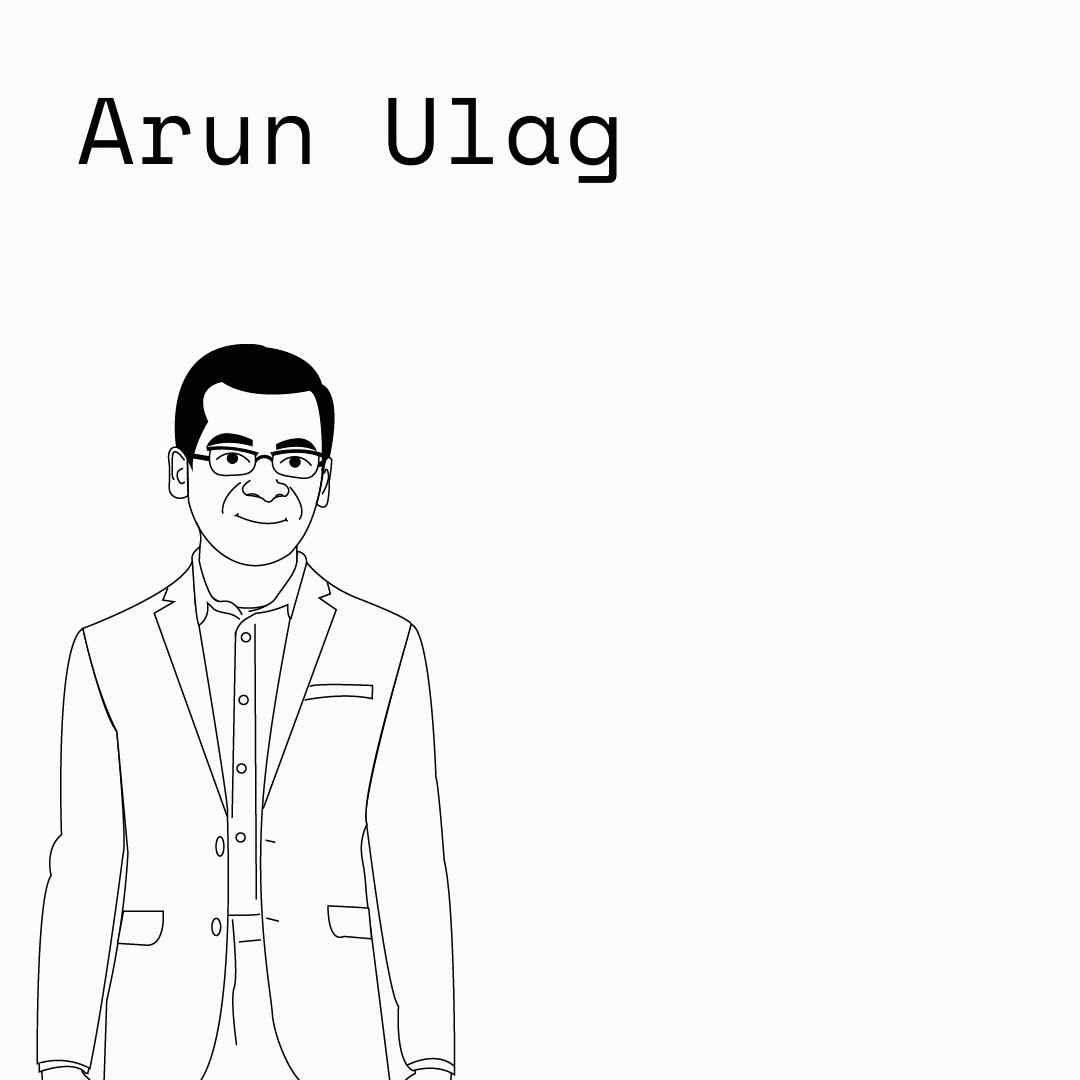
Intelligence Platform is a Cool Name, w/ Microsoft CVP Arun Ulag
Corporate Vice President of the Business Intelligence Platform at Microsoft
04.01.21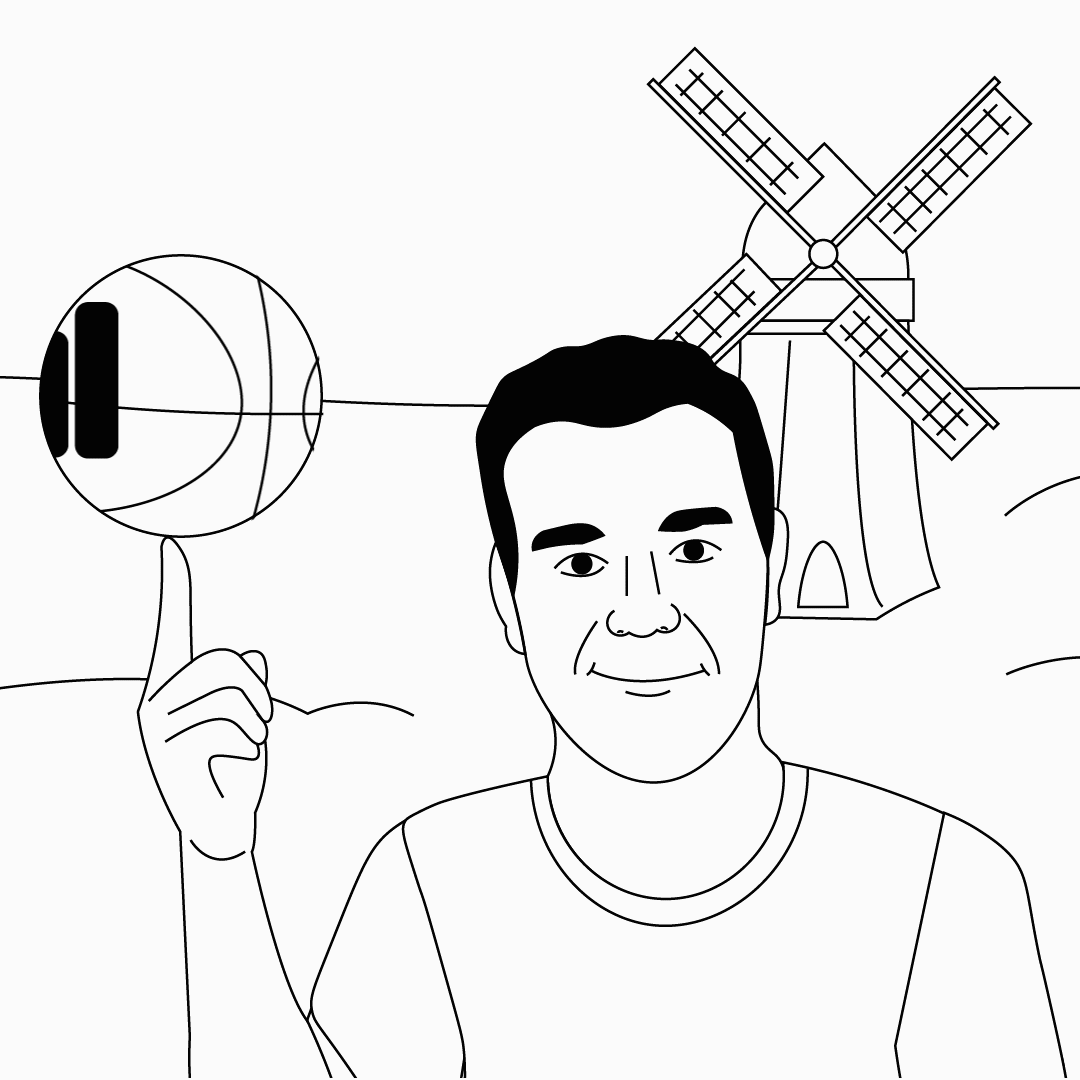
The Dutchman of Data Dunked on Me, w/ Kasper de Jonge
Analytics Advisor and Principal Program Manager-Power BI, Microsoft
03.23.21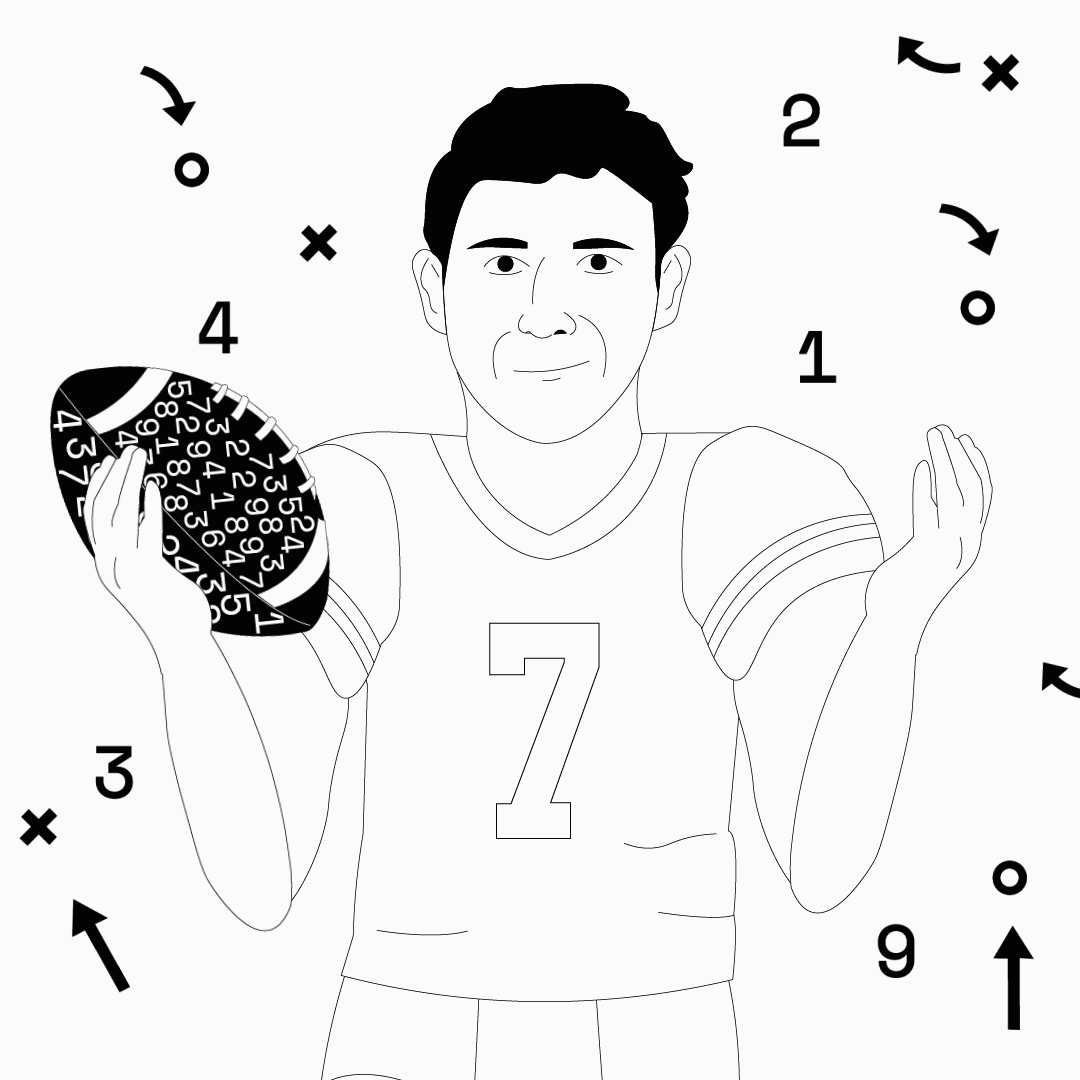
Everyone’s in the Middle, w/ Former NFL QB Hugh Millen
Former NFL Quarterback and Data Nerd
03.09.21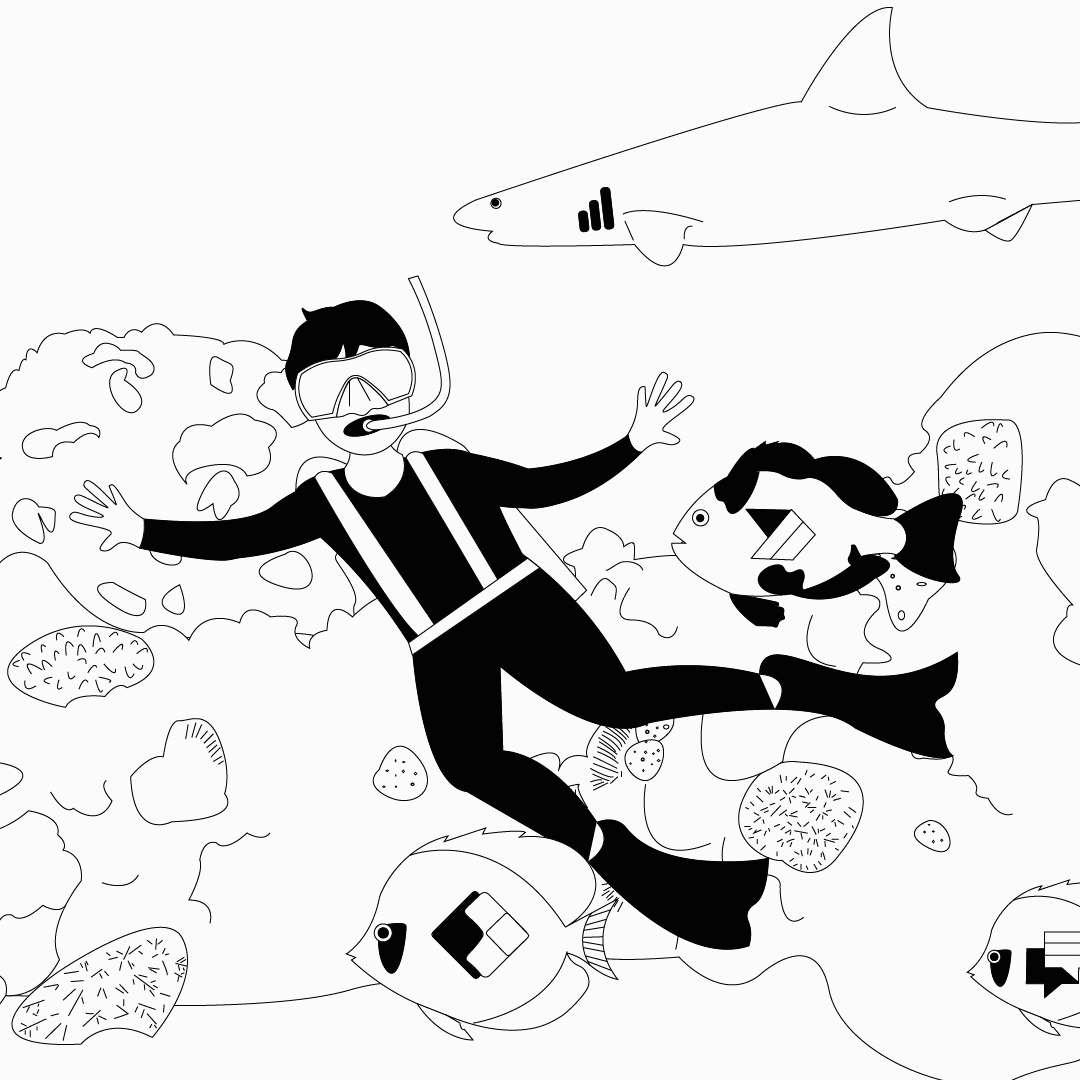
Marine Biology Leads to Power Platforming, w/ Chuck Sterling
Senior Program Manager, Microsoft
02.23.21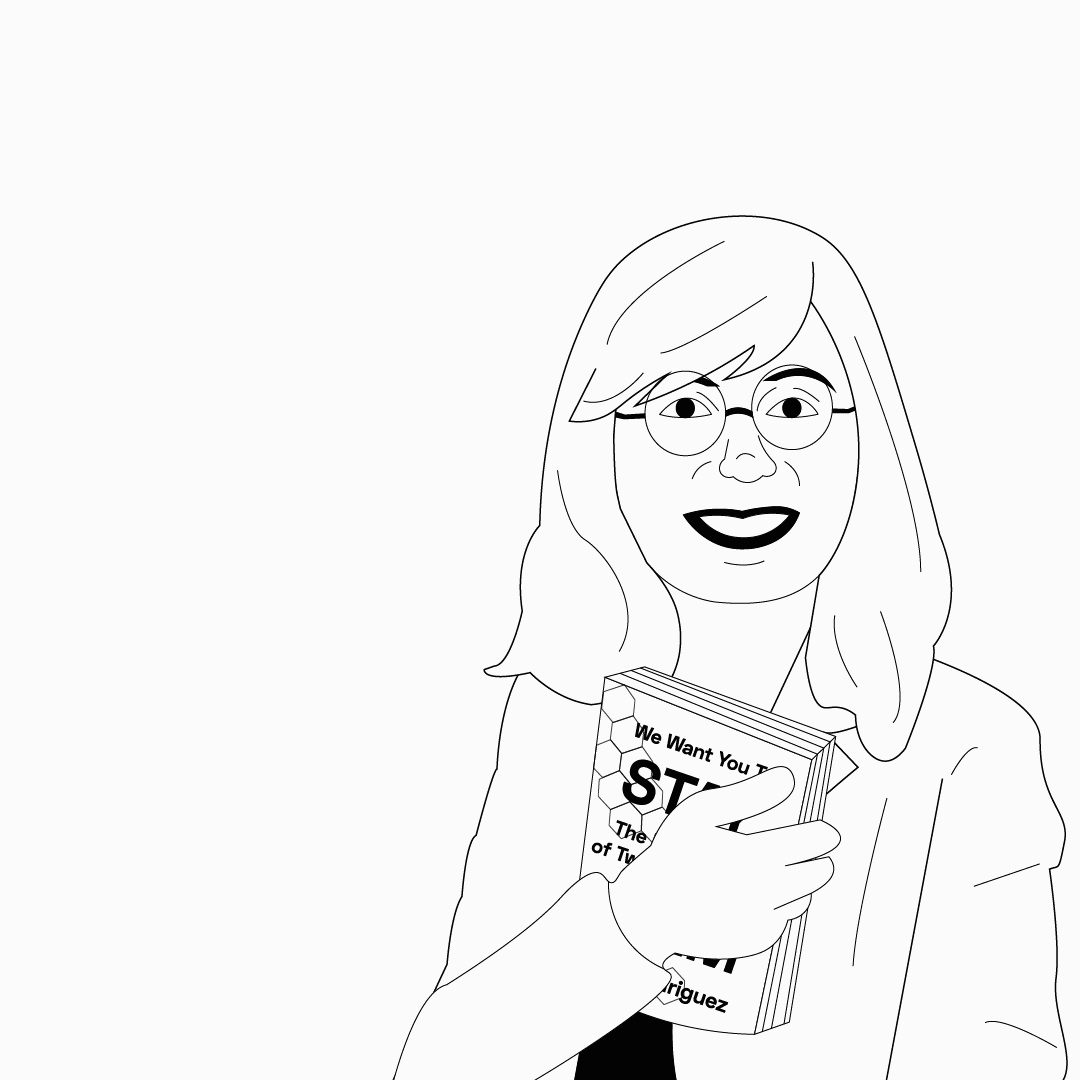
Data Chefs Can Come From Anywhere, w/ Gartner’s Lori Rodriguez
Vice President Executive Programs at Gartner
01.26.21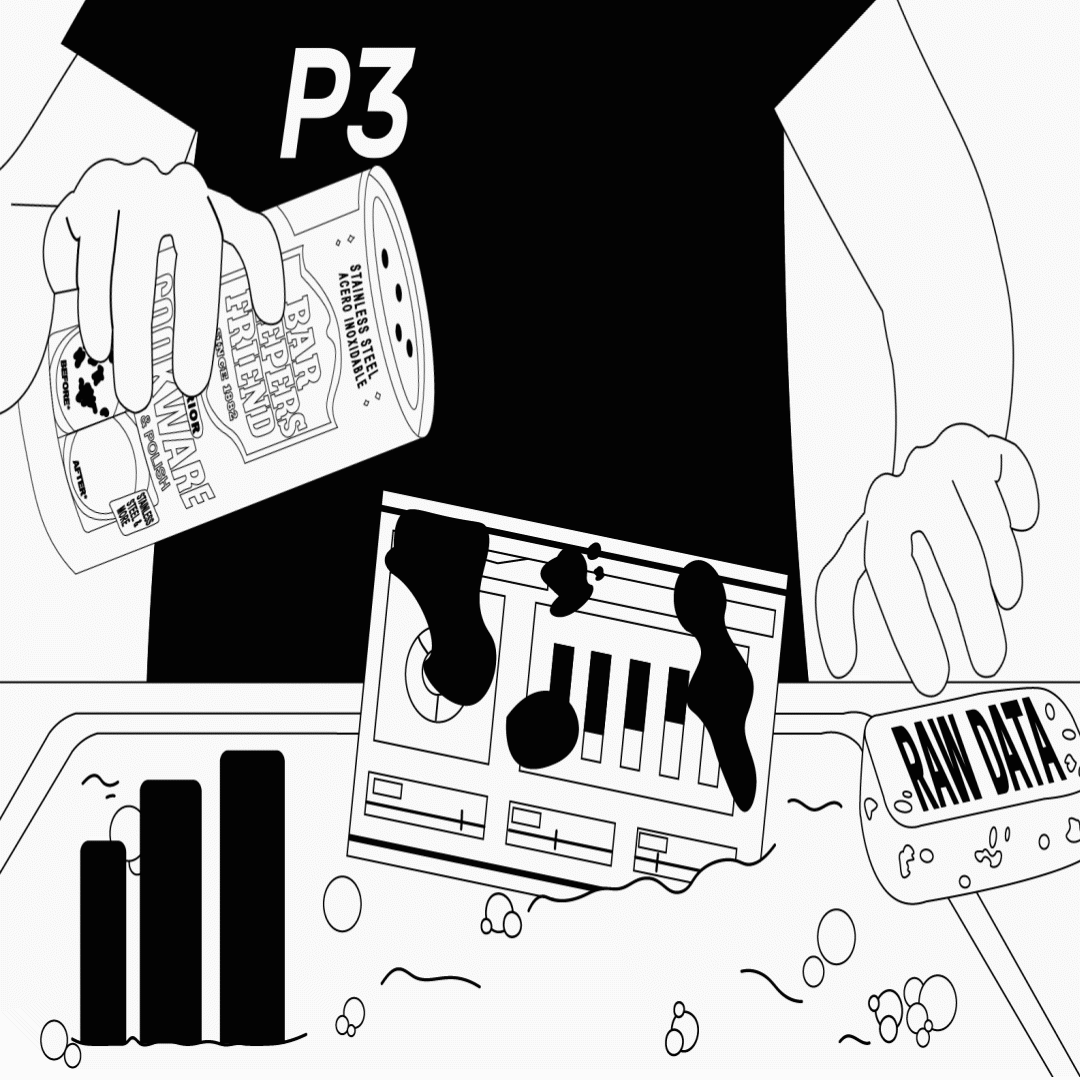
Scrubbing Data & Beating COVID, w/ Matt Selig of Bar Keepers Friend
Exec. Vice President, Chief Financial Officer & Chief Human Resources Officer at SerVaas Labs
01.05.21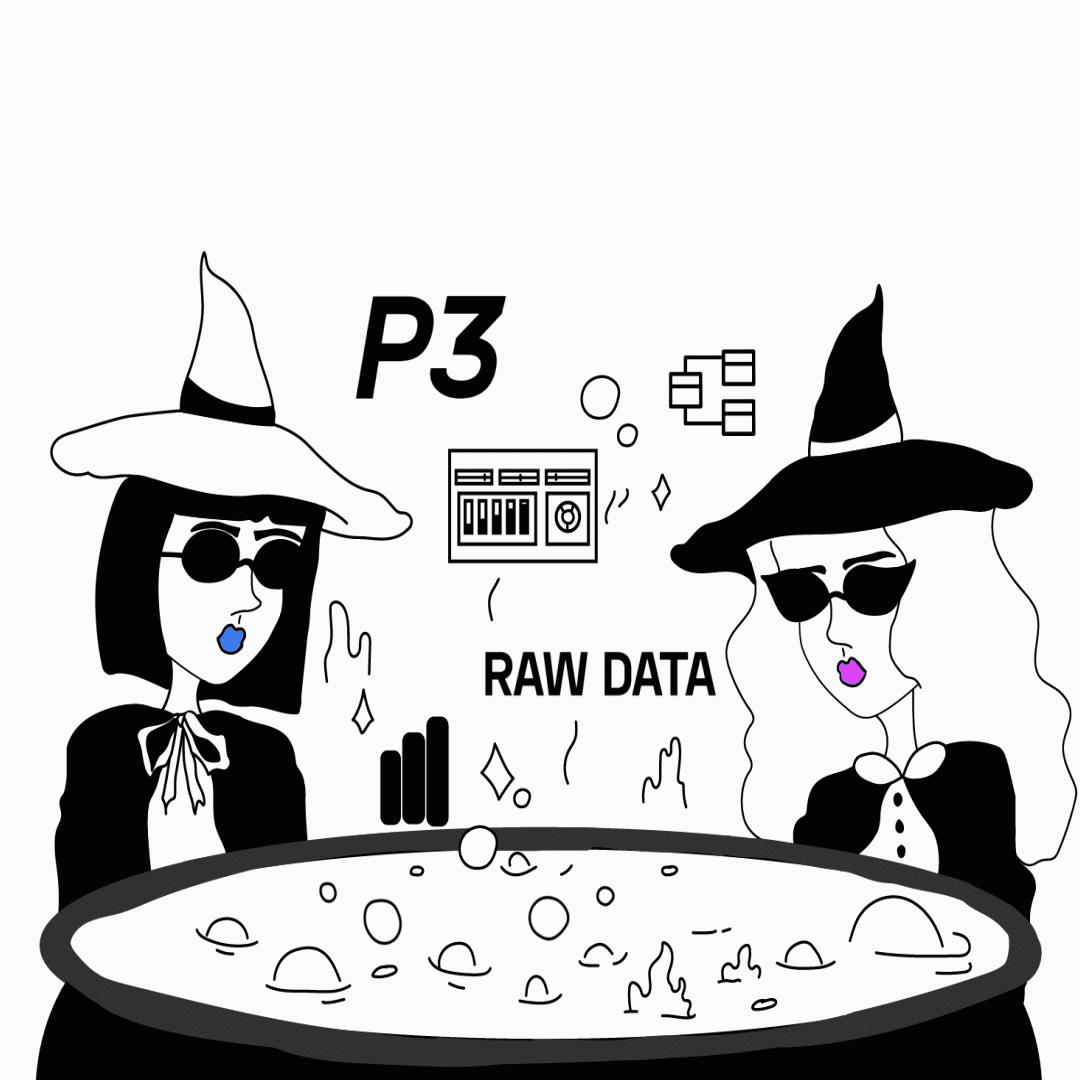
The Data Witches, w/ Shannon Lindsay & Stephanie Bruno
Shannon Lindsay-Microsoft Certified Data and Analytics Professional &
Stephanie Bruno-Associate Director of Informatics at the Elizabeth Glaser Pediatric AIDS Foundation (EGPAF)
12.22.20
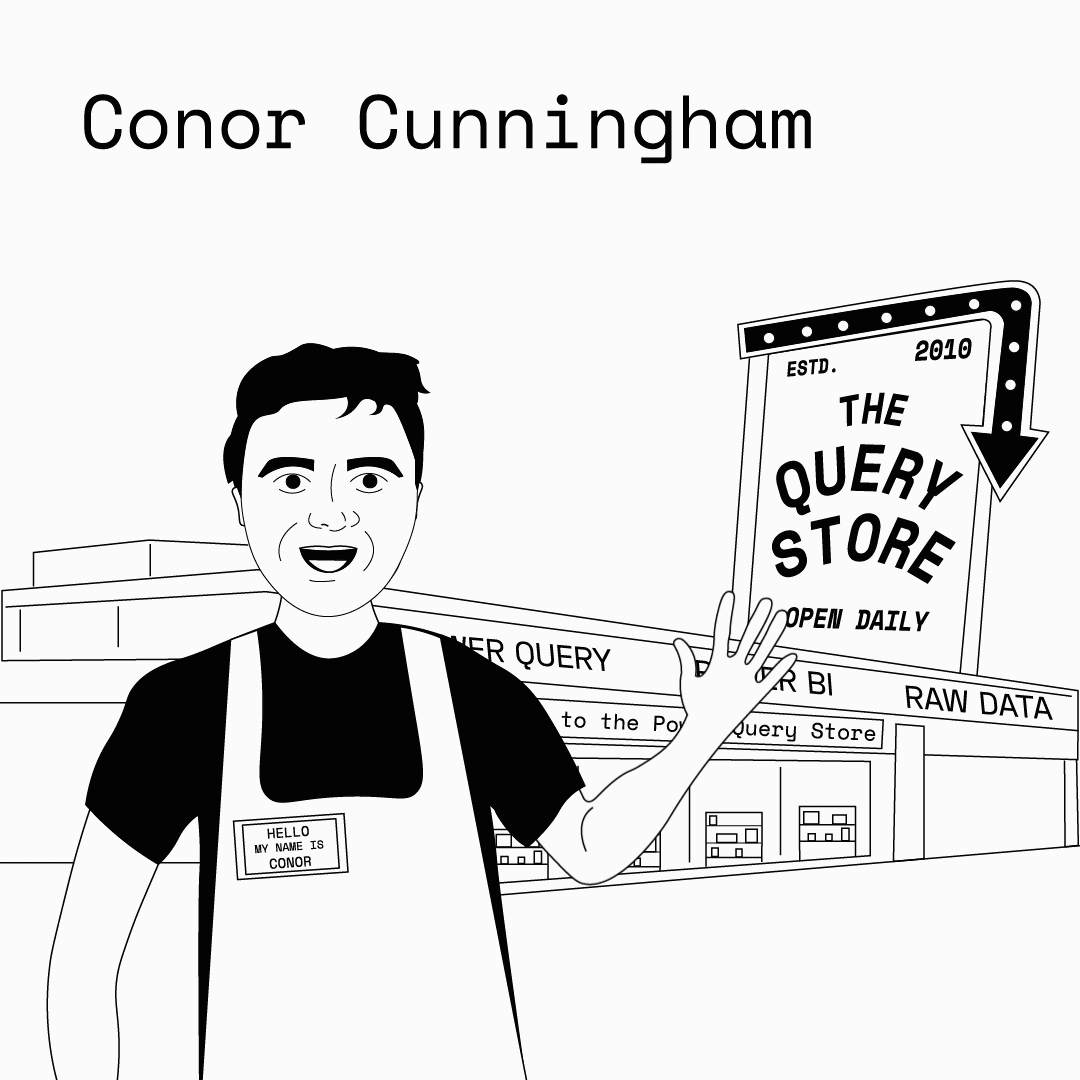
The Query Store’s Open for Biz, w/ Microsoft’s Conor Cunningham
Partner Software Architect, SQL Server Engine-Microsoft
12.15.20One of the World’s Most Amazing Humans, w/ Matt Allington
Power BI Consultant, Trainer, & Microsoft MVP
12.01.20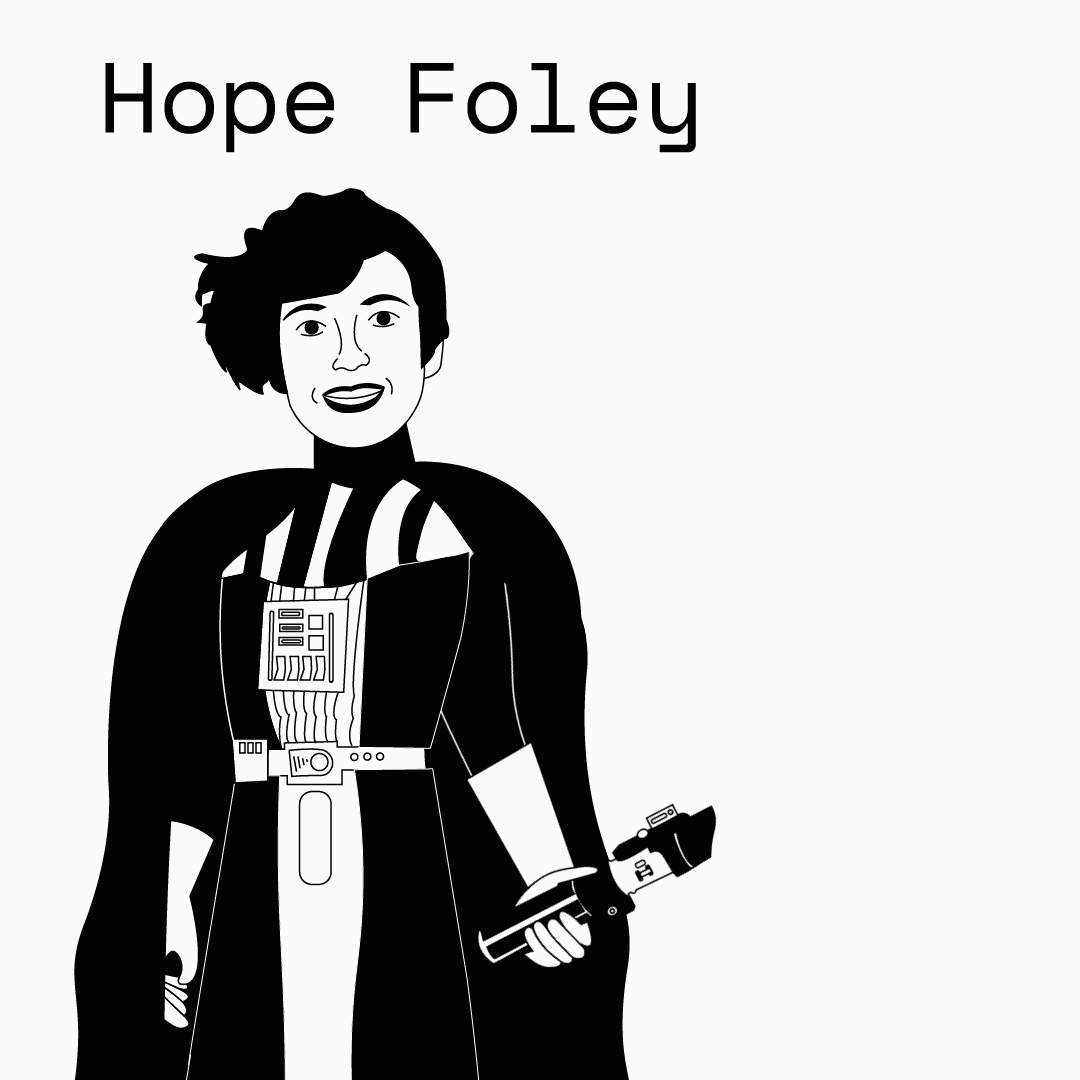