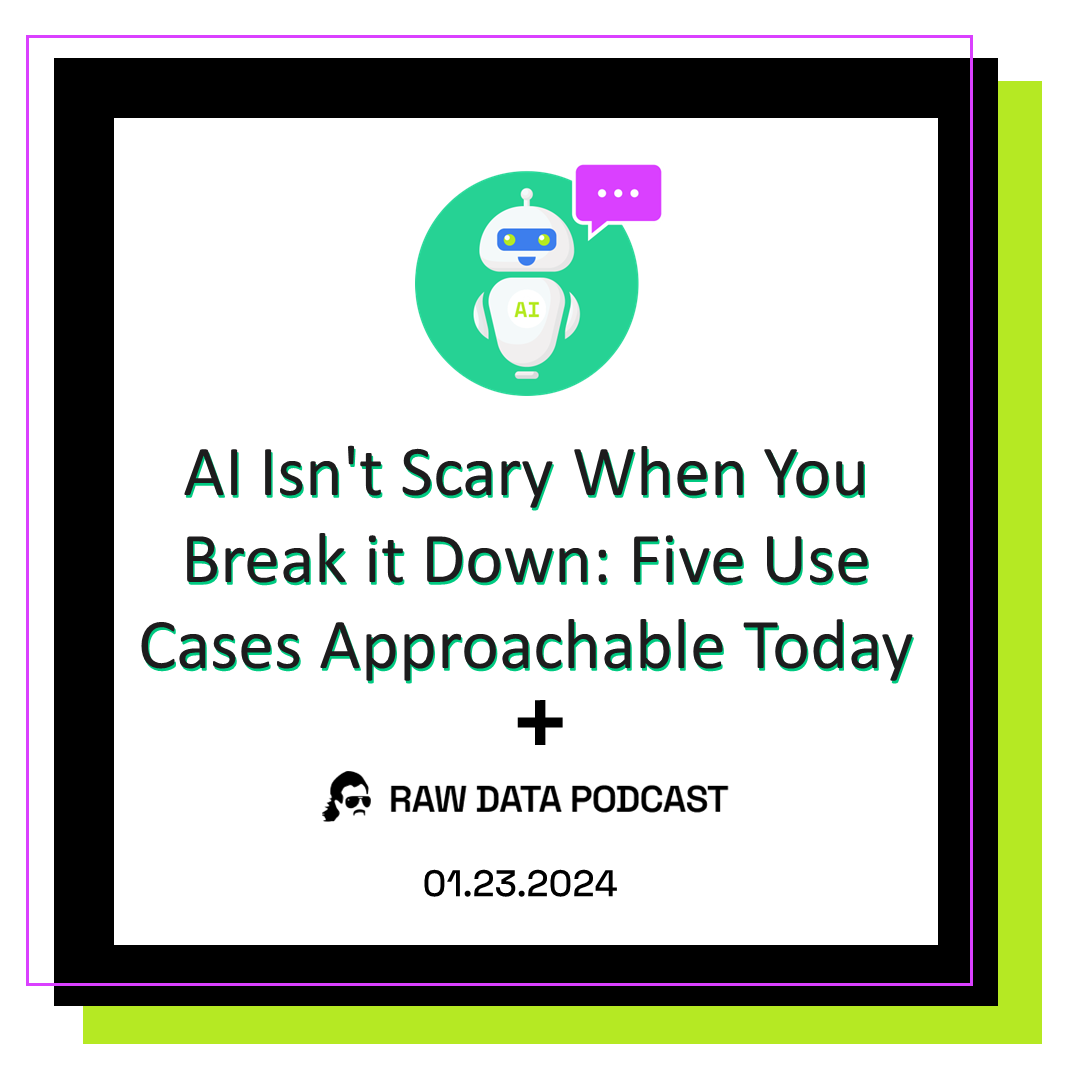
Raw Data By P3 Adaptive
AI Isn’t Scary When You Break it Down: Five Use Cases Approachable Today
Listen Now:Today, we’re taking you on a deep dive into the ever-evolving world of AI, and we want you to feel like you’re right here with us, exploring the tools and features that can truly supercharge your business. Imagine this: harnessing the power of AI to predict customer loyalty and prevent them from drifting away – it’s all about aligning AI projects with your business goals, without getting caught up in the buzz or getting left in the dust.
While some of the latest AI tech is still in its testing phase, Rob and Justin are excited to encourage you to jump right in and start experimenting. Take a moment to explore AI apps on your phone, strike up a chat with Microsoft Copilot, and watch the magic unfold as you introduce your data into the mix. Think of it as a quick dip into the captivating world of AI, where possibilities are endless.
Both Rob and Justin agree: AI holds immense potential, and businesses that hesitate to explore it might just miss out on groundbreaking opportunities.
Oh, and one more thing before you go – if you’ve enjoyed our podcast, be sure to leave a review on your favorite podcast platform to help new listeners find our show!
Rob Collie (00:00): Hello friends. This week, Justin and I sat down to try to de-fang one of the most blood-curdling questions you can be asked. And what is this question? Well, imagine someone very important at your workplace comes up to you and looks at you very sternly and they ask, "Hey, what are we doing about AI?" They expect you to know the answer. Or maybe no one's asking you this question, but you're asking it of yourself.
(00:28): In both cases, this is a very difficult question to answer while simultaneously feeling like a question that you should have a confident answer for, difficult to answer, but you feel like you should have an answer. Doesn't get much worse than that. Those are the uncomfortable moments, aren't they? And it's not just a difficult question, it's actually truly an impossible question to answer in the way that it's formulated both because it's not actually a single question. It's actually many questions.
(00:57): And because it's many questions, you actually have to break it down into its component questions. Each one needs to be evaluated separately. Secondly, it's difficult/impossible to answer because it actually presents AI as the goal. What are we going to do about AI? It has the same formulation as a question like what are we going to do about our declining market share in category X? Even though they're formulated with a similar structure, they're very different questions.
(01:27): AI is a tool. It's actually many tools, many different kinds of tools. Because it's a tool, it is not a goal. You should not have an AI strategy. You should have a business strategy and understand the different places where AI might support those goals. In terms of the way that we approach this podcast, I think the most valuable thing that we aim to do on an ongoing basis is to demystify complicated topics, to bring them down to earth, de-fang them, make them less scary, and turn them into things that are actionable and understandable by business leaders and technical practitioners alike.
(02:08): By that metric, I think today's show very well might compete for the title of Most Valuable show we've ever done. But that's not up to me, is it? That's up to you. So let us know when we get into it.
Announcer: (02:24): Ladies and gentlemen, may I have your attention, please?
(02:27): This is the Raw Data by P3 Adaptive Podcast with your host, Rob Collie, and your co-host Justin Mannhardt. Find out what the experts at P3 Adaptive can do for your business. Just go to P3Adaptive.com. Raw Data by P3 Adaptive is data with the human element.
Rob Collie (02:54): All right, Justin, let's get down to business, shall we? The thing that I want to talk about today, kind of been percolating in the back of my head for a while, is that I'm positive that all over the country, all over the world right now, there are people asking themselves or being asked by important people who work with them, the following question, "What are we doing about AI?"
(03:18): It's one of those questions where when someone asks you something like that, you're really in the headlights. You have to have an answer to this. You can't be the one that doesn't have an answer to this question. It's a terrifying question and it's also a very difficult one to have an answer to.
Justin Mannhardt (03:34): It is.
Rob Collie (03:35): I think one of the biggest reasons why it is difficult to answer this question is because it's actually many questions masquerading as one.
Justin Mannhardt (03:44): Yes, and also answers for the sake of answers. It is difficult. You're in an organization. Your CEO for example is asking you that question, what are we doing about AI? Answers for the sake of answers, and this is where the fluffy stuff maybe comes in is like, "Oh, we're going to modernize and revolutionize and increase the productivity of our people and infuse AI into our products and services." That all sounds well and good, but it sort of misses the why part that goes back to the dawn of time.
Rob Collie (04:20): And it's one of the things that you've been emphasizing lately that I've completely agreed with is it's similar to being asked what's our data strategy? Well, no, no, what's our business strategy and how can we use data to advance the business strategy? Okay, sounds like you're saying the same thing in those two formulations, right?
Justin Mannhardt (04:40): Yeah.
Rob Collie (04:40): But you're not.
Justin Mannhardt (04:41): That's right.
Rob Collie (04:41): As a topic sentence, each one kicks off a different set of thinking, a different set of topics that you're running down in your head and one of them is much more productive than the other. You say that the pressure to answer the question kind of generates fluff. If you reformulate the question as what are the places where we could be using AI to advance our business goals? Again, sounds like the same question, but it's not.
(05:08): One of these has a productive life after it, the other one doesn't. When you take that reformulation of the question and then you start to decompose it into all of the different types of places where you can apply AI, it becomes a list and you can start to evaluate the list in a little bit more detailed fashion, which makes it tangible. It makes it an answerable question when you are able to drill down to this next level of detail. But if you just leave it as AI, the big umbrella term, it means so many different things.
(05:47): What I thought we'd do today is do that decomposition, and not the rotting kind of decomposition. I mean the breaking it down.
Justin Mannhardt (05:54): Break it down.
Rob Collie (05:56): The decomp tree.
Justin Mannhardt (05:57): When you frame it that way, "How can AI help us with our business goals?" It creates the safe space, if you will, where the answer could be, "Oh, we don't need to do anything fancy flashy with AI to achieve this outcome." And that's okay. Some of this is becoming table stakes in the marketing lane. Yeah, we have AI in our thing. I was like, what does that really mean? Did you just wire up the GPT for API? What's really improving?
Rob Collie (06:28): Justin, we have Matthew McConaughey on TV-
Justin Mannhardt (06:31): Right.
Rob Collie (06:32): ... telling us about Salesforce in incredibly non-specific terms.
Justin Mannhardt (06:38): I know.
Rob Collie (06:39): How Salesforce is going to help us with AI.
Justin Mannhardt (06:42): I wonder what that check looks like. Hey, McConaughey, if you ask yourself is data the new oil?
Rob Collie (06:52): There's new sheriff in town. Let's start here. Let's say I'm a company that already has made a relatively significant investment in Power BI. I've already got some good Power BI models and they're now called semantic models. They used be data sets. I've got a bunch of reports, dashboards, whatever, and I'm getting value out of that. What would be a way that we could use AI, broadly speaking, to get more value out of those models, out of that investment?
Justin Mannhardt (07:24): A lot of businesses are asking themselves that "What should I be doing about AI" question, and when they say AI, that's caught up in the hype cycle of generative AI, these things that can produce text or images or media. That's all really exciting. But in our space, the intersection of the work we do, I am concerned that machine learning is getting a backseat to all of that because the value in some of these things to predict things like customer churn or recommended product bundling, that sort of stuff, that's machine learning discipline.
(08:03): I've not seen yet, again, this is all moving really fast and improving a lot, how these large language or even just refer to as the multimodal model world, how well that's going to interject into the analytics exercise directly. I haven't seen it yet. I'm sort of optimistic that it's out there and it's coming, but I see that generative space as being more aligned with things like creativity, efficiency, personal productivity, than it is deep down in the analytics and the data driving better decision-making.
Rob Collie (08:40): Riffing off of what you're saying there, Justin, if I've got a Power BI model that includes things like measuring attrition customer churn, first of all, knowing the score on that is important.
Justin Mannhardt (08:54): That's right.
Rob Collie (08:55): And being able to sub-categorize customer term by demographic or product line or whatever, know where we're doing well, know where we're not doing so well, so we can sort of focus our attention. But when it comes to that thing that we're always talking about, improvement, it seems to me that the subset of AI that we call machine learning probably has some direct application to better informing the actions I take to improve our customer attrition. Can we talk about that a little bit and how close it is to being deployable? How far do I have to walk through broken glass today to start getting this kind of value? How close to deployable is it?
Justin Mannhardt (09:36): Let's stay in your hypothetical scenario here. You've built a data model and a set of reporting in Power BI that's helping you understand customer churn, and maybe it's helping you improve it-
Rob Collie (09:51): Yes.
Justin Mannhardt (09:52): ... via your understanding. I think just to sort of oversimplify, what you're really after is you want to maybe better predict this customer is likely to churn out and here's how we might prevent that, for example.
Rob Collie (10:09): Who and why?
Justin Mannhardt (10:10): Who and why, and can we do something about it? That's hard to do in Power BI semantic models and reporting because you're trying to predict something before you've accumulated all of the data that helps you see that very clearly. Where you could evolve this into machine learning is okay, now your semantic model can be read by a machine learning process in Fabric. This is what a data scientist would do. They would understand here are the variables and measures that are associated with customer churn.
(10:47): You can train a machine learning model to say, "Okay, here's some actual history. Here's what actually happened and here's the variables and then I'm going to give you some other actual history," but I'm not going to tell you the outcome or just keep it simple. Did the customer churn, yes or no? Then the machine learning technology works out algorithms to figure out, "Okay, these compositions of things suggests it's more likely that a customer will or won't churn out," for example.
(11:15): And so what your machine learning application can do is it can read your, let's say early data about customers and it's looking at some of those signals, demographic data, buyer behavior in your platform, transactional information, customer service events, whatever you might have going on there and it can say, "Hey, these patterns of activities suggest these probabilities of outcomes." The machine learning model is actually going to write the output of that data to your lake house and Fabric for example, which then you can read back into your Power BI reporting.
Rob Collie (11:48): Like as a new column.
Justin Mannhardt (11:49): As a new column or a different fact table or whatever it is. And so now you have a report that says, "Hey, here's the early warning signs on customers that are likely to churn out and here's maybe a recommended course of action," because you could train that too. You could say "Here's the ones we were able to retain." Maybe there's some data about what happened to do that you could improve that for there.
(12:13): I think those sorts of predictive applications or predicting waste in a manufacturing process is another interesting idea. Predicting deviations in your business forecast. These are all things that are difficult to do and traditional BI, you're just sort of looking backwards and it's hard to get that level of sophistication in the forward-looking prediction sometimes. Sometimes you can do it, but these are ideas that you could think about.
Rob Collie (12:41): Because Microsoft is with their Fabric strategy, which essentially is the Power BI model, kind of the center of everything, the Power BI model is the lake house, is the one lake storage format, is the Power BI model, is the AI machine learning source as well. So because they're explicitly lighting up all of their AI tools, the whole suite essentially, in a way that they can see into the Power BI models that we've been building, not for AI, we've been building them for other purposes.
(13:12): As the side effect, now we're much closer to that than we ever were before. It's just sitting right there now, unlike some other things we're going to talk about on the show today. This isn't wait-and-see this. This isn't "Maybe they'll figure it out. Maybe it's going to get better over time, so keep an eye on it." This is ready to go.
Justin Mannhardt (13:28): Yeah, and there is still a need, at least from my perspective, there's still value in data scientists and data engineers that understand that part of the puzzle. It's not like Microsoft has made machine learning completely point and click like they did with ATL yet. But there are some interesting things, and this is what's been going on with Copilot. They call it Chat Magics. It's still sort of a shiny demo territory.
(13:55): I've not played around with it a ton, but you can say like, "Hey, here's some data. I want to predict customer churn. What type of machine learning model would make sense for this application?" And it explained, "Oh, you should be using a regression or a whatever, and here's the base code." The base code on these modeling applications, I'm not talking pages and pages and pages of this stuff. I think it's a learnable thing for a lot of people. I'm interested to see how this matures into more of that self-service world.
Rob Collie (14:27): In the end, there's always that last mile of human input that is crucial. Just to kind of put a bow on this one, from a business leader's standpoint, this one is so much more in range. It went from out-of-range to in-range for almost anyone that would care. Most people were priced out of it before. It's just sitting there on the table today. It requires a little bit of thought. Not everyone on our team is data science literate enough to run this sort of stuff, but we do have people that are.
Justin Mannhardt (15:00): Right.
Rob Collie (15:01): Working in conjunction with Power BI models that you already have, that might need a little bit of tuning but not very much in order to make the machine learning model work. We mentioned some scenarios here like customer churn, but you mentioned some others, but there's just so many places where there are very, very, very subtle hard-to-detect patterns in the data that help you.
(15:25): It's not perfect, but it's a probability thing that helps you predict and therefore potentially act differently. It's not just churn. Even from the customer example, sometimes it takes a while to understand that this is developing into a really good customer. They might be telegraphing, there probably are telegraphing in some really subtle ways, that I might be a whale in the first earliest interactions. If you're going to assign extra attention to them, there's a crucial window where they might become whale or not.
(15:56): The ability for machine learning algorithms to spot these patterns is magic. I remember there was a machine learning algorithm that was being fed pictures of retinas, people's eyes. It was able to predict... Not predict. It was able to accurately classify retinas into male and female and the people building the model, and people looking at the pictures, the biologists associated with it, they had no idea what it was picking up on. They didn't understand what it was, but they knew it was right all the time.
Justin Mannhardt (16:21): This is part of what Austin's described. We live in a golden age of analytics. The pace at which machine learning technology has advanced over the last decade is pretty amazing.
Rob Collie (16:32): Most people have been priced out of it, not because the machine learning technology wasn't available or practical, but because getting the data ready for it was too difficult. It turns out that now if you've got a Power BI model, you're ready for it. So, giddy up.
Justin Mannhardt (16:50): Let's go.
Rob Collie (16:51): All right. What a great place to start. Another big category is using Copilot to generate code. Now of course, Copilot now means more than writing code in the office context.
Justin Mannhardt (17:04): Copilots.
Rob Collie (17:05): Copilot helps me write a form letter. It helps me write code, and code means a lot of things.
Justin Mannhardt (17:13): It does.
Rob Collie (17:14): Like DAX formulas, are code.
Justin Mannhardt (17:17): That's right.
Rob Collie (17:18): Power Query scripts, are code.
Justin Mannhardt (17:20): That's right.
Rob Collie (17:21): Python notebooks are code.
Justin Mannhardt (17:23): Yeah.
Rob Collie (17:24): And then there's code is code.
Justin Mannhardt (17:26): Code is code.
Rob Collie (17:26): Like C Sharp. Copilot's been a thing in GitHub for a long time.
Justin Mannhardt (17:31): If I'm not mistaken, I think that might've been the first Copilot as they released Copilot on GitHub.
Rob Collie (17:38): I think what they discovered is that's such an amazing name. This is one of those examples where Microsoft really got a name right.
Justin Mannhardt (17:45): Yeah. Ding.
Rob Collie (17:47): It is so good. It is such a good name that they're like, "It doesn't even matter if the technologies aren't related. It's a great brand name." If you're in the Power BI adjacent space right now, what's the state-of-the-art of Copilot? Where are the places where you could sort of really lean in on it versus places that are like, "I'll wait and see. It's not quite baked yet."
Justin Mannhardt (18:10): I would say just like an overall disclaimer. This is obviously all very new. Copilot was announced not that long ago and all these Copilots I think are technically still in some state of a public preview. The world is still trying to figure these things out. The areas where Copilot exists and where it could be helpful is in Power BI Desktop, it's just sort of sneaky. It's not super obvious that there, is what used to be called Quick Measures, got replaced with Copilot and Power BI Desktop.
(18:42): Think of that like your DAX assistant. I don't know that it's remarkably better than Quick Measures, for example. I can't say that it is a silver bullet, but for the things that you don't know how to do, a lot of these large language model applications, which Copilot is, that's what it is, it's all about your efficiency and your productivity. It might not get you to 100% of where you need to end up, but it might get you 80% of the way there and it might get you there faster than if you were bouncing around reading articles you've written or reading Marco's articles.
(19:15): So when you describe something like, "Hey, I want to measure that gives me same store sales year-over-year," and you're just not familiar with that pattern, it could help you with that. But, this is really important, there's actually a page in the documentation. The title is Update Your Data Model to Work Well with Copilot for Power BI. Guess what this thing says?
Rob Collie (19:37): Oh, I'm waiting.
Justin Mannhardt (19:38): Have good table names, have good column names, have good relationships, have a good star schema structure. All these modeling fundamentals are really important in getting quality assistance from these types of Copilots. That's one area. We've obviously seen some scenarios where the DAX generated by Copilot could be further optimized for faster speed and things like that.
Rob Collie (20:05): What a great thing, right?
Justin Mannhardt (20:06): Yeah.
Rob Collie (20:06): Great thing even for us. Every time something happens that makes the value of something that you do, it increases the applications of it, the value of what you do goes up. Our company is, at least partly it's not the only thing we do, but it's certainly the core of what we do, is Power BI. To do Power BI well, you got to do good data models. If you have good data models, you're more likely to be able to use the Copilot feature and write some of your own formulas, another plus 5% on the value of a service that we provide.
(20:39): Rather than replacing us like P3, it's increasing our value. Bring it on, love it. Even there with the code generation stuff, there's sort of two categories of it and I think this is a theme that we'll probably come back to. One is help me do something that I don't know how to do, and one is help me do something that is incredibly tedious.
Justin Mannhardt (21:00): Exactly.
Rob Collie (21:01): I know how to do it, but it's tedious.
Justin Mannhardt (21:03): Yeah.
Rob Collie (21:04): Things that I do in Power Query over and over and over again. It's just like, oh so gross.
Justin Mannhardt (21:08): It's interesting how little I've seen that scenario talked about. For example, there's a third party tool for Power BI that we all really love using called Tabular Editor. One of the things you can do is you can sort of script out common things. For example, I want a time intelligence set of measures for all of my base measures, and so instead of me sitting there teeing all these out, I can execute a script to iterate through the base measures and just create all that for you.
(21:39): I haven't seen that yet where you just like, "Hey Copilot, give me-"
Rob Collie (21:42): A whole family of measures.
Justin Mannhardt (21:44): "... me a whole family of common stuff that I know I always need."
Rob Collie (21:46): I think we should be just as excited about the stuff that removes tedium. Right?
Justin Mannhardt (21:51): 100%.
Rob Collie (21:53): That's a much more solvable problem for AI. I recently was working on a formula and I had no idea how to do it. I really don't think that Copilot is going to be anytime soon able to help me write that formula. Chris Haas helped me write that formula.
Justin Mannhardt (22:09): You and I don't shy away from the fact that our day-to-day involves a lot less DAX compared to years ago.
Rob Collie (22:18): Yes.
Justin Mannhardt (22:19): I've been working on a model for something and I needed to write a measure and man, two years ago Justin, would just know how to do this.
Rob Collie (22:28): Yeah, totally.
Justin Mannhardt (22:29): I used the Copilot. I was like, I want to know the same calculation but for these conditions. I just can't remember the pattern right now, and it helped me. That's kind of where things are with DAX. On the M side, I haven't seen anything in Power BI Desktop yet for Power Query, but they do have, it's called Copilot for Data Factory, which is the ETL service in Fabric, which includes Power Query in that via data flows.
(22:59): There's a capability there where you can say, "I only want to keep the rows where the quantity was over the median value of the data." That's not something that's easy to go click through the UI in Power Query to make that happen, but Copilot will help you generate the M function that would do that for you, for example. I think those things are pretty cool too, or even simple stuff where you say, "I want a data flow that connects to this OData service and pulls back this entity," and it'll get you started.
(23:32): Just to summarize the rest of it, there's Copilots in notebooks that can help you write your Python or build data science models. There's a lot of things out there and I think the whole idea is can it make you more efficient and help you move faster?
Rob Collie (23:45): All right, what should we talk about next? This is one I'm particularly excited about. Data models, Power BI data models you build. One of the primary values of a Power BI data model, it powers reports. Okay, but in a very sharp distinction between Power BI and its competitor tools, a Power BI data model, semantic model, is kind of there to create all the reports, even the ones you're not thinking about today.
Justin Mannhardt (24:11): That's right.
Rob Collie (24:12): This is one of the reasons why, not the only reason, but one of the reasons why it's such a nimble tool in practice compared to its competitors that look the same. It's like invest in the intelligence behind the scenes once and then get all the reports as opposed to go do some intelligence development, get a report, want another report, oh, got to go back to the drawing board, more back end, blah, blah, blah.
Justin Mannhardt (24:38): Right.
Rob Collie (24:38): Once you have that, the way I describe it, this Power BI model is like the lower case O Oracle that you can go to ask any question, and it can answer it. Except that you can't interact with it like that. It's a field list of check boxes and stuff, and a blank page if you're not looking at an existing report. It's also oftentimes, even if you're the one that built the model, it is sometimes incredibly tedious to build the report that you're looking for, right?
Justin Mannhardt (25:07): Yeah.
Rob Collie (25:08): Where are we on eliminating tedium, sit down against a well-constructed Power BI model and say, "Okay, give me a report that shows me blah, blah, blah year-over-year, but filter out this and give me a slicer that controls," whatever. Just I don't want to be clicky, clicky, draggy, draggy for 30 minutes to execute on something that is already super, super, super clear in my mind what I want to see.
Justin Mannhardt (25:39): This is amazingly powerful to me. I can't emphasize the point enough. Everything I'm going to talk about is so predicated on good data models. All this stuff really falls apart when the data models aren't good. I might blast that too many times in this episode. A couple features that I think just really empower business leaders and users of all kinds in Fabric is to work with Copilot to create reports and to interact with natural language to say, "I need to lay out a report page that helps me better understand my cashflow cycle over the past 18 months or to better understand customer profitability."
(26:22): It can suggest the outline of these report pages. It can build the reports for you. The level of empowering people to generate those outputs, because there is a level of friction when you're like, "Oh, how do I get the data label to look right? Which chart do I want?" That's really exciting, is to just connect to a model and say, "This is the problem I wanted to understand. These are the things I'm interested in. Can you recommend a layout or a set of reporting and produce that for me just right now?"
Rob Collie (26:57): Yeah, in last week's podcast about the hockey stuff, I'll give you an example. Do you know how awesome it would be... Again, even if I wasn't expecting it to do any deep thinking, for me to just say, "Hey, give me a new report page titled Stars of the Week that's got three bar charts on it, one showing goals, one showing assists, one showing points, sorted descending. Make sure to put the Indie Inline hockey logo in there. Put the dashboards courtesy of P3 Adaptive label in there like I have on my other report tabs."
(27:31): I could describe that in 30 seconds or less. Even that relatively simple report, I mean I'm going to be sitting there for 15 minutes lining that thing up, laying it out, and it's not the smart work.
Justin Mannhardt (27:42): That's right. Even to be maybe not as specific in your prompts, I think that's my favorite thing with this generative technology is the rubber duck effect where you can say, "I'm interested in this type of information with my hockey league," for example, and to get some suggestion back too, you're still in control of the reporting canvas, so you could get something and you like 80% of it and you can still go modify it and tweak it on your own.
Rob Collie (28:08): It's a non-judgmental rubber duck.
Justin Mannhardt (28:09): Yeah.
Rob Collie (28:10): It's a safe place. Seriously, there's something funny about this, but it's also very real. It's a safe place for you to sit down and ask questions, even dumb questions, learn that they're dumb, ask smarter questions, and then the next time you're interacting with human beings, you're that much smarter about the business. You're that much smarter about the art of the possible in Power BI.
(28:34): Again, if you have a good Power BI model, you're a business leader, you're not technical, this is something that you can be doing today. Is it still called Copilot? I thought they had another name for this, for the report maker.
Justin Mannhardt (28:45): No, this is different. Copilot and Fabric can help you create reports and then there's another feature called Auto-Generated Quick Reports that has AI technology in it, but that's not a chat-based experience. Copilot is a chat-based experience. The Quick Reports is, at least when I played around with this, is you go in and you check fields from the columns and the measure list and then it sort of tries to infer what you might be wanting to do. That's a different thing.
Rob Collie (29:17): I'm going to ask a question that's a legitimate question of mine, but I think other people listening to this will probably have the same question. Where is this chat experience? You can show me where it is. It's not in Power BI Desktop?
Justin Mannhardt (29:27): No, this is in the Power BI service or what would now broadly be known as Fabric.
Rob Collie (29:32): This is just available today in Fabric?
Justin Mannhardt (29:34): It's available only on certain versions of the Fabric SKUs.
Rob Collie (29:39): Certain license levels, price points.
Justin Mannhardt (29:41): If you're curious what you need, we can help with that. You can start and stop these things for just like R&D. If you wanted to do an R&D hour, you could totally do that.
Rob Collie (29:49): What's the next category we should talk about, Justin?
Justin Mannhardt (29:51): Well, you mentioned earlier we do many different things. A lot of what we do is Power BI, but we also play in other areas of the Microsoft stack, other areas of the Power platform, things like Power Apps. Again, this is a great term Copilot, but it's also sort of getting-
Rob Collie (30:10): It's like AI.
Justin Mannhardt (30:12): Yeah.
Rob Collie (30:13): Once you use it too many times, it becomes confusing. What are you doing about Copilot? Well, shit.
Justin Mannhardt (30:20): There's another thing that I think is really interesting for mid-market companies to think about is things like chatbots. There was a feature in Power platform that was formerly, it might still be called Power Virtual Agents, and that was you could sort of build this thing. This same type of concept is all wired up with the generative AI things, and so you can now build what are called Copilots.
Rob Collie (30:44): Oh, no.
Justin Mannhardt (30:45): Let's say you wanted to create a customer service agent, for example, that can help someone navigate their issue and get to the right spot to get it solved. And so you get sort of the richness of the conversational experience. You can sort of train it on the tone and things and you can provide it the resources it would need to refer to in answering these things. Then you can integrate these things into things like your Power Apps experience or into your websites and things like that.
(31:14): That's one of the areas that's just sort of moving way too fast for me to even keep up with because now people are producing all these sorts of agent-type applications to be helping with different things. That was also elusive. You would never build this type of thing in-house before the technology arrived. That would've been a tall order for mid-market companies.
(31:33): I just think the ability to build those types of boutique experiences for your customers or your teams or even think about internally, like knowledge base solutions for example, would you rather dig through a Wiki to find the policy about something? Or would you rather say, "Hey Lukebot, where do I find our policy around this?" And it can not only answer your question, it can direct you to where you need to be going.
(32:05): I think that's some of the Microsoft 365 Copilot opportunity, Rob, where it's aware of your entire estate, it's aware of your OneDrive, it's aware of your documents, it's aware of your Dynamics. More human adjacent fact-finding and researching.
Rob Collie (32:23): Okay, so this is pretty close. In fact, the whole Power Virtual Agents thing has gotten a lot better with the wiring up of GPT.
Justin Mannhardt (32:31): No, they changed the name. They call it Microsoft Copilot Studio. You can build your own Copilots to-
Rob Collie (32:36): Okay, okay, hold on. Hold on just a moment here. I want the sound effect to be included in the show. I was trying very hard to have me just absolutely smash my forehead into the microphone. It's Copilot Studio for building Copilots. Dammit.
Justin Mannhardt (32:54): Yeah.
Rob Collie (32:55): Earlier in the show I said, it's a great name.
Justin Mannhardt (32:58): It's a great name that means 27 different things.
Rob Collie (33:02): And they are going to beat it to death.
Justin Mannhardt (33:05): It's like, what's the better name? Some sort of assistant thing. We built a Copilot. I'm not sure if it has legs for example, but this is some of the ideas we're having to do things like scan resumes or to read your blog posts, see how that goes.
Rob Collie (33:21): These chatbots that they're calling Copilots now can be internal-facing, they can be external-facing?
Justin Mannhardt (33:26): That's right.
Rob Collie (33:27): They can face your customers, your partners, and in a lot of cases like that speed up service times for your customers and partners while reducing the amount of busy work for employees that could be valuable doing other things. So, you create time-
Justin Mannhardt (33:45): That's right.
Rob Collie (33:46): ... not save it. You create time internally while at the same time your customer actually likes it too. I've actually recently experienced the first time that I interacted with a chatbot and felt like it was good. I was in the Chipotle app. I ordered from the wrong restaurant. I wonder why it didn't stop me from ordering from a Chipotle in another state. But once I did, I was able to go in and talk to the chatbot, and it was like, "Yeah, no problem. We got you." It was awesome. I was like, there you go. First time I interacted with a machine, and it was better and faster than interacting with a human.
Justin Mannhardt (34:17): Yeah, it's also cool with these Copilots, one of the things when you go through setting them up is you can give it a website, so you can give it your website and now this Microsoft Copilot thing you're building can digest what you're saying on your website and make that part of its knowledge. I think it's really cool. It's funny you brought up the food delivery thing, Rob, because the other day I was trying to order something for lunch and I was seeing all these restaurants I hadn't heard of. I was like, "This is cool. There's new stuff around." And it was still set to [inaudible 00:34:50].
Rob Collie (34:53): Order food for Rob. Yes, this is fantastic AI. All right, here's one that seems like it's in range, but maybe it's still a little ways out. Go get me data to enhance the quality of my analysis. What's the state-of-the-art there? Government statistics are a notoriously difficult source to track down and puree into a usable format. I don't know if for example, there's anything going there.
Justin Mannhardt (35:22): I don't know about that yet. The thing I would worry about on that scenario, for example, let's say you wanted... The census data is popular, demographical information. I don't know that generative AI is good at reciting back some of those facts and figures I think mainly because of the hallucination problem. I think it could successfully direct you to the right government website that you need to refer to. I guess I've not personally seen an example where I can do that yet.
Rob Collie (35:52): All right.
Justin Mannhardt (35:53): Something that might be interesting to talk later in this episode is the Copilot for Data Factory. That can help you get wired up to data sources.
Rob Collie (36:01): By generating M.
Justin Mannhardt (36:02): By generating M, or prompting you through. You could say, "Oh, I want to pull in the data from the Census website," and its, "Oh, what's the website?" And then get that integrated into your model, for example.
Rob Collie (36:12): What about hypothetically a folder tree full of PDF files? It's got some regularity to it. I just came out of a process where over the holidays I personally spent probably a week fighting that beast, and winning, which is amazing.
Justin Mannhardt (36:29): Good job. Good for you.
Rob Collie (36:31): But doing it with Power Query, doing it with M. When you look at these files, it really does strike me as a perfect AI/ML scenario. If I had a spreadsheet format set up for you and have people going through these PDFs manually re-entering the data into the Excel format, that's a robotic process.
Justin Mannhardt (36:54): It is.
Rob Collie (36:55): It just seems like a perfect application of why do I need to go through this incredibly tedious process of writing all this M? I'm kind of hoping that you say no, it's not possible because it doesn't make me feel like I wasted my time over the holidays. But I think that kind of problem has got to be super, super common.
Justin Mannhardt (37:14): Super common, and I think there's two opportunities. The certainty on successful outcome is sort of not very high yet. Again, all this stuff is so fast and new. So you had to work through this M and hand-write some functions, and do some things that Rob Collie would prefer not to do.
Rob Collie (37:36): Correct.
Justin Mannhardt (37:38): Again, I think the gap is because this Copilot thing isn't in Desktop yet for Power Query. I do think you could have gone to the thing in Fabric Copilot and saying, "I need to combine these PDF files in this location into a common data structure that has these columns." Maybe that would've gotten you there faster. Maybe it wouldn't have. You asked me to see if I could do something related to your hockey league, which was a lot of your score sheets before the data you have were handwritten.
Rob Collie (38:10): Yes.
Justin Mannhardt (38:12): I said, I wonder how well things like GPT4 could do at reading that and producing a structured data output. I haven't invested a ton of time in this yet, but what I found so far is it does do a decent job of transcribing the printed text. It's not great at the handwritten stuff and I don't know if that's because of the safety nets and GPT4.
(38:41): Okay, is this one of those things where you'd need an actual machine learning engineer to train it, how to interpret this thing? This is boutique, but it does make me wonder on the exports from the scoring system, could it have done it basically optical recognition on those and given you structured data output, just here's a table and you don't need to worry about the Power Query.
Rob Collie (39:04): Yeah, I'm starting to get sad now. It sounds like that would've been fast.
Justin Mannhardt (39:07): Maybe.
Rob Collie (39:08): But then on the refresh each week when I get a new one-
Justin Mannhardt (39:11): That's where you'd have to go, "Oh hey, chatbot. Here's a new PDF."
Rob Collie (39:15): We need a Power Query M interface into this stuff.
Justin Mannhardt (39:19): That's right.
Rob Collie (39:20): We need to be able to call it and rerun it because if it's just a one-time thing, there's a little bit of an air gap it sounds like still there. There's a general generative AI craze of creating content, and that's somewhat outside of our field of practice, but it's adjacent to it in some ways.
Justin Mannhardt (39:42): It's outside of it. It's also very real for us just as a company I think in the same way it is for any company. The advice I would have for others is you really do need to get curious about these things and figure out where it can help your business strategy. Just to make it relatable for our world, for example, Microsoft just announced that Copilot for 365 is GA. And so-
Rob Collie (40:13): Generally available.
Justin Mannhardt (40:14): Yep, generally available. You can go buy it regardless of who you are or how big you are, which is great. It's the experiences in PowerPoint and Excel, and email, and Loop and all these other things. For us, we need to figure out how to use this effectively and how to coach our team on how this works well. Part of that is just giving it to a few people and say, "Can we be committed to playing around with this and seeing what does and doesn't work well?"
Rob Collie (40:44): It reminds me of 1996, my new employee orientation at Microsoft. There was new employee orientation on one day and then over the course of the next few weeks, there was one day a week where they would have a scheduled presentation for us and we'd go listen to a Microsoft veteran talk to us. I forget who she was, but she was the best of all of these guest speakers that we had.
(41:07): Her advice was... She had two pieces of advice. Number one, protect your weekends. Microsoft will try to take your weekends. Don't let it. Work hard during the week, but protect your weekends. The second piece of advice she gave was use software. It doesn't matter what it is, be using software all the time, other people's software, other company's software. Be an avid consumer of software. You're going to be learning things a lot.
(41:33): For example, this advice would've been amazing to be following at the moment that the smartphone revolution started. The first people to start using smartphones and realize this was a computing device and there's a new kind of software, and how is this software different from the web page? How is the Facebook app different than Facebook.com in your browser? There's all these things to be learning about. I think you're doing this. You're in town recently and we went axe throwing.
(41:59): Just for grins, you're taking your phone out and you're taking pictures of this scorecard that we're just with a marker, a whiteboard marker on a handheld whiteboard keeping score. You're taking screenshots and sending it to GPT and also taking screenshots of the rules of the game that are sitting there on the table and asking it, "Hey, who won?" It was pretty good. It was figuring this out. This is one of those "use software" moments. Give the official Justin recommendation, what app should people have installed on their phone so that they can be playing around with this kind of thing in the same way that they might be absentmindedly scrolling social media?
Justin Mannhardt (42:41): I have two on my phone right now and I'm sure there are others that I don't even know about, but I have the official Chat GPT mobile app. I use the browser app on a regular basis as well. Then I have the Microsoft Copilot app, which I think it more or less replaces what was happening with the Bing app where that generative AI was sort of in that Bing experience because in Copilot you have an option where you can say if you want to use GPT4 or not.
(43:07): But the Copilot one, I think it does a little bit better job as a research assistant where it will summarize an idea in response to your question, but it also sites sources and direct you to web pages and things like that. Those are the two that I have at the moment. And now, we just got a few of the other Copilot licenses. One joke that's come out of that is you can get a Copilot summary of a Microsoft Teams meeting and it bullets out actions that were discussed in the meeting and one of the actions just says, "Justin will take care of the issues over the next week." I'm like, oh, that's sort of a helpful summary.
Rob Collie (43:48): Either that, or one hell of a meeting. Why did we waste an hour?
Justin Mannhardt (43:51): Yeah.
Rob Collie (43:52): Justin's on it. Okay, so those two apps, the official Chat GPT app and the Microsoft Copilot app.
Justin Mannhardt (44:00): Microsoft Copilot.
Rob Collie (44:01): Either or both of those require subscriptions, licensing, et cetera?
Justin Mannhardt (44:06): The Copilot does not. You can sign in with your Microsoft account. GPT, I believe you need their pro license, which I think is $20 a month for a user. It's the same service you would use on the web, just on your mobile. I've been using GPT so much, Rob, just at my desktop. I have it open in the browser and I use it for all sorts of things during the day, but when you bring it to the mobile device, that's the first time I was like, "Oh shoot. We could take pictures of what we're doing out here at axe throwing and see..." Or you imagine you're traveling and you see an interesting bird, you snap, "What is this?"
Rob Collie (44:46): We've been using the Google Lens, the Google app on our phones over the weekend to take pictures of Lego mini figs because we're going through all of our kids' old Legos that are just all dumped in a bin. Some Lego mini figs are like worth $2, some are worth $150. You don't even know, because the pieces parts, they're not even all together. Take a picture of a torso of a mini fig just sitting on your table and it tells you what the mini fig is. Then you can see what the other pieces are, and you're like, "Oh my God, those pieces go together. Whoa, look at that, a $100 mini fig."
(45:20): We also have an app for Lego that it isn't quite what I want it to be, but you just dump a bunch of Lego bricks on your table and take a picture of this random pile of Lego bricks and it'll tell you what sets you have enough bricks to build.
Justin Mannhardt (45:35): Wow.
Rob Collie (45:36): What I want to do is tell it what sets we have because we've got this big stack of instruction manuals and say, "Okay, what parts are we missing?" As a big mountain of Lego bricks, it's worth X to sell this stuff. But if they were sold as used as kits, it's like 20X. There's like thousands of dollars of difference in market value of these things.
Justin Mannhardt (46:05): The secondhand Lego market is this amazing. Tangent alert, but...
Rob Collie (46:10): It is. It is, and it is ripe for AI disruption. [inaudible 00:46:15] explained to you. If you're out there listening, this is what we should do. Let's just should pivot the whole company into building the world's greatest resale market Lego brick AI tool.
Justin Mannhardt (46:25): It seems pretty smart to me.
Rob Collie (46:27): Totally. Yeah. Meeting adjourned.
Justin Mannhardt (46:28): Start tomorrow.
Rob Collie (46:29): Justin will take care of all of the issues.
Justin Mannhardt (46:31): I will take care of the issues. The only thing I would reinforce in this whole hype cycle of AI is tying this back to the FOMO/phobo topics we've brought up is I'm personally just trying to stay curious and patient with the generative AI technologies because it's moving real fast and I'm just sort of like, "Well, what's going to get traction in the marketplace?" Microsoft's obviously a first mover with things like Copilot, but we've got a long history of what does and doesn't work in business intelligence. We don't have that about generative AI yet. I think curiosity and patience is important.
P3 Adaptive (47:11): Thanks for listening to the Raw Data by P3 Adaptive Podcast. Let the experts at P3 Adaptive help your business. Just go to P3Adaptive.com. Have a data day.
Sign up to receive email updates
Enter your name and email address below and I'll send you periodic updates about the podcast.
Other Episodes
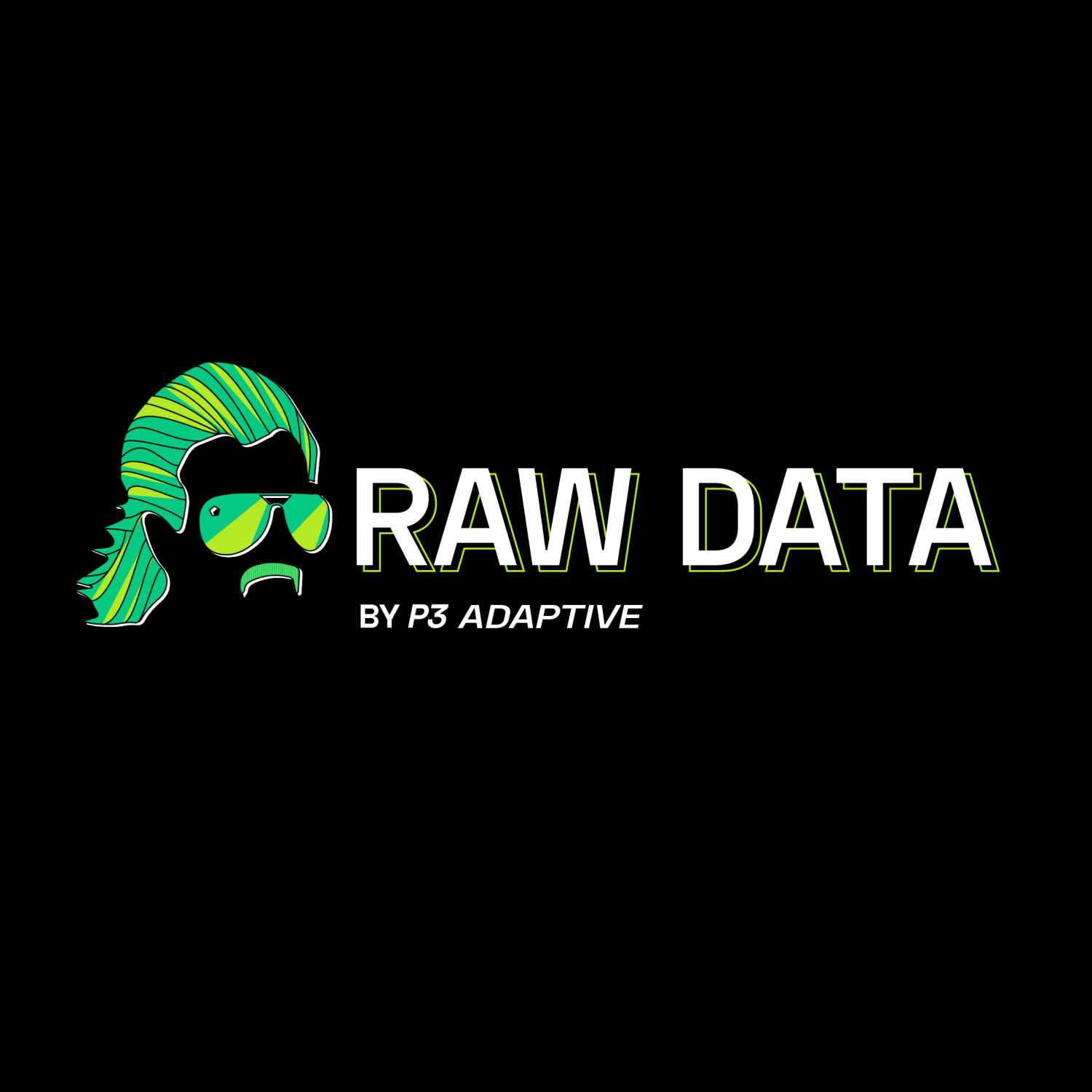
Adjacent and Between: Demystifying Digital Transformation with Power Apps and Power Automate
03.26.24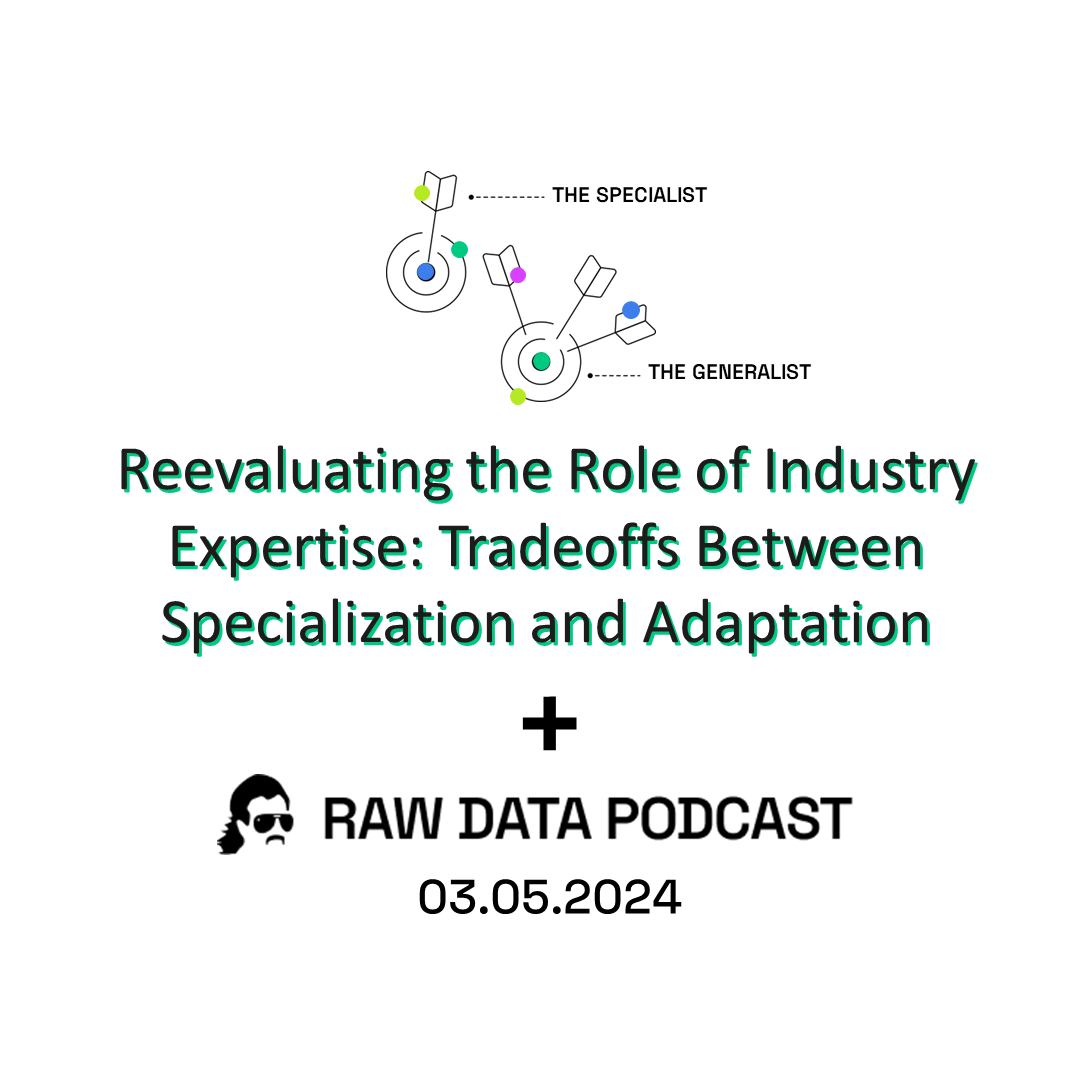
Reevaluating the Role of Industry Expertise: Tradeoffs Between Specialization and Adaptation
03.05.24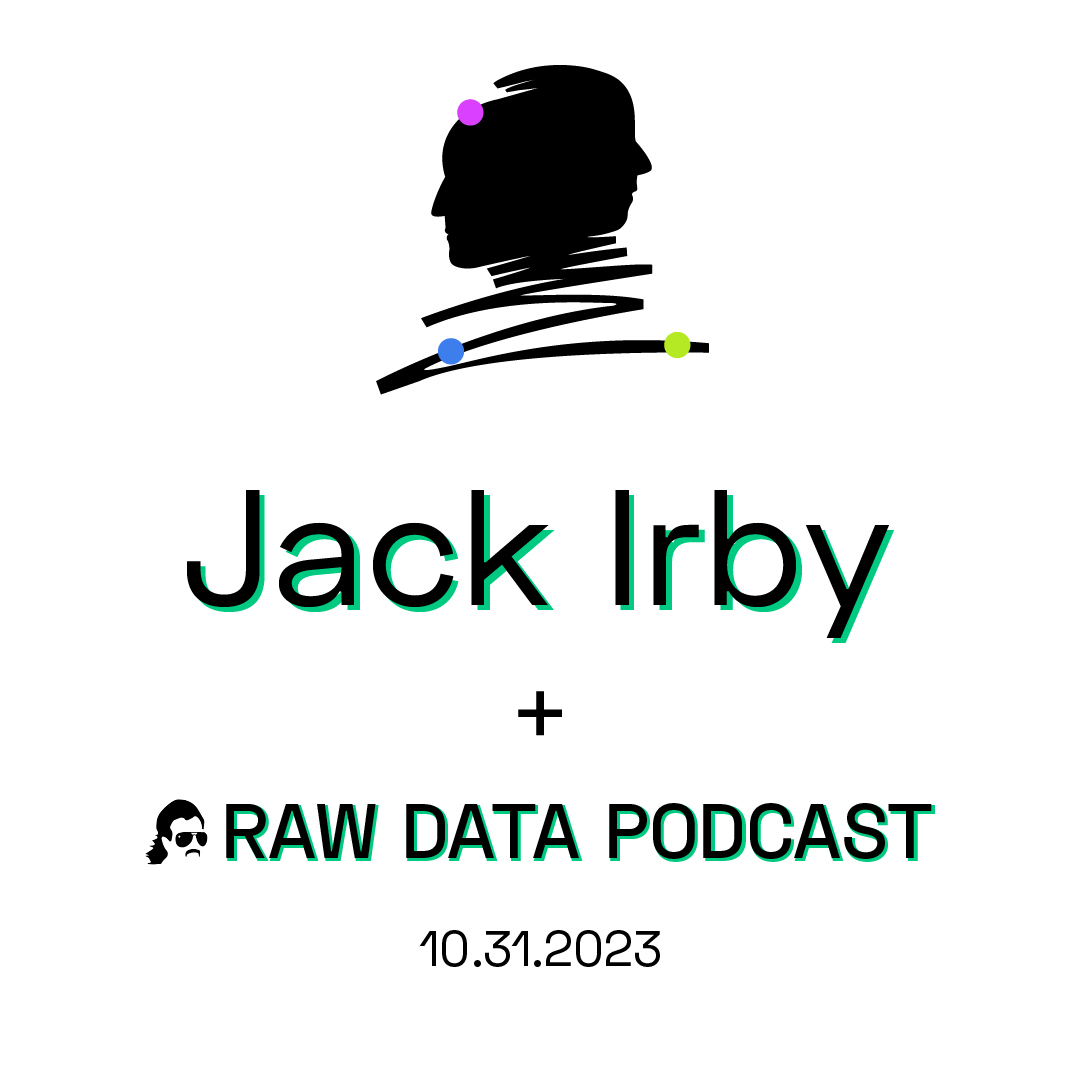
The Fruit of the Electric Grapevine: Industry-Leading Visibility on Limited Resources, w/ Jack Irby
10.31.23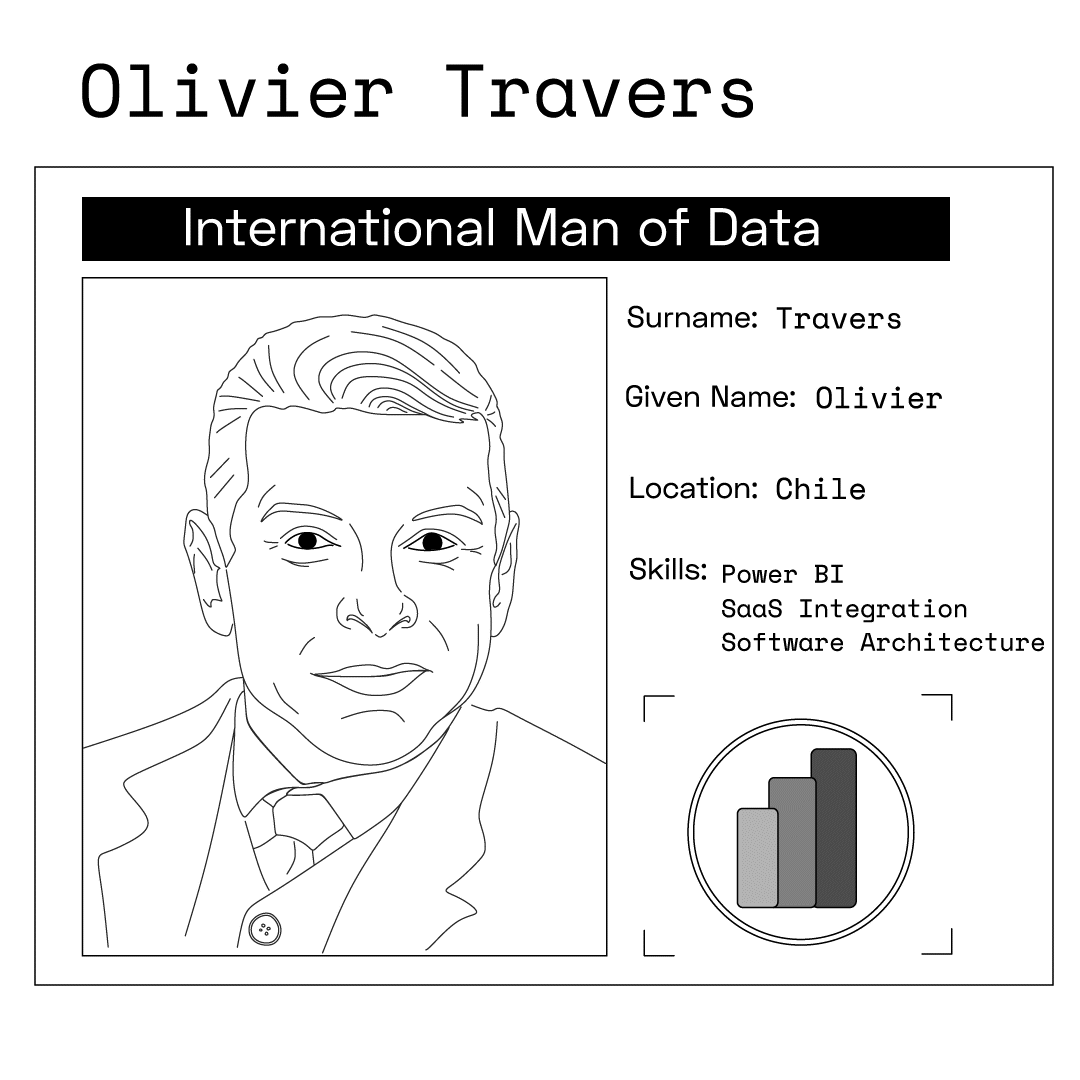
Stay Away from Guadalcanal, w/ Olivier Travers
MS Data Platform International Man Of Mystery
01.04.22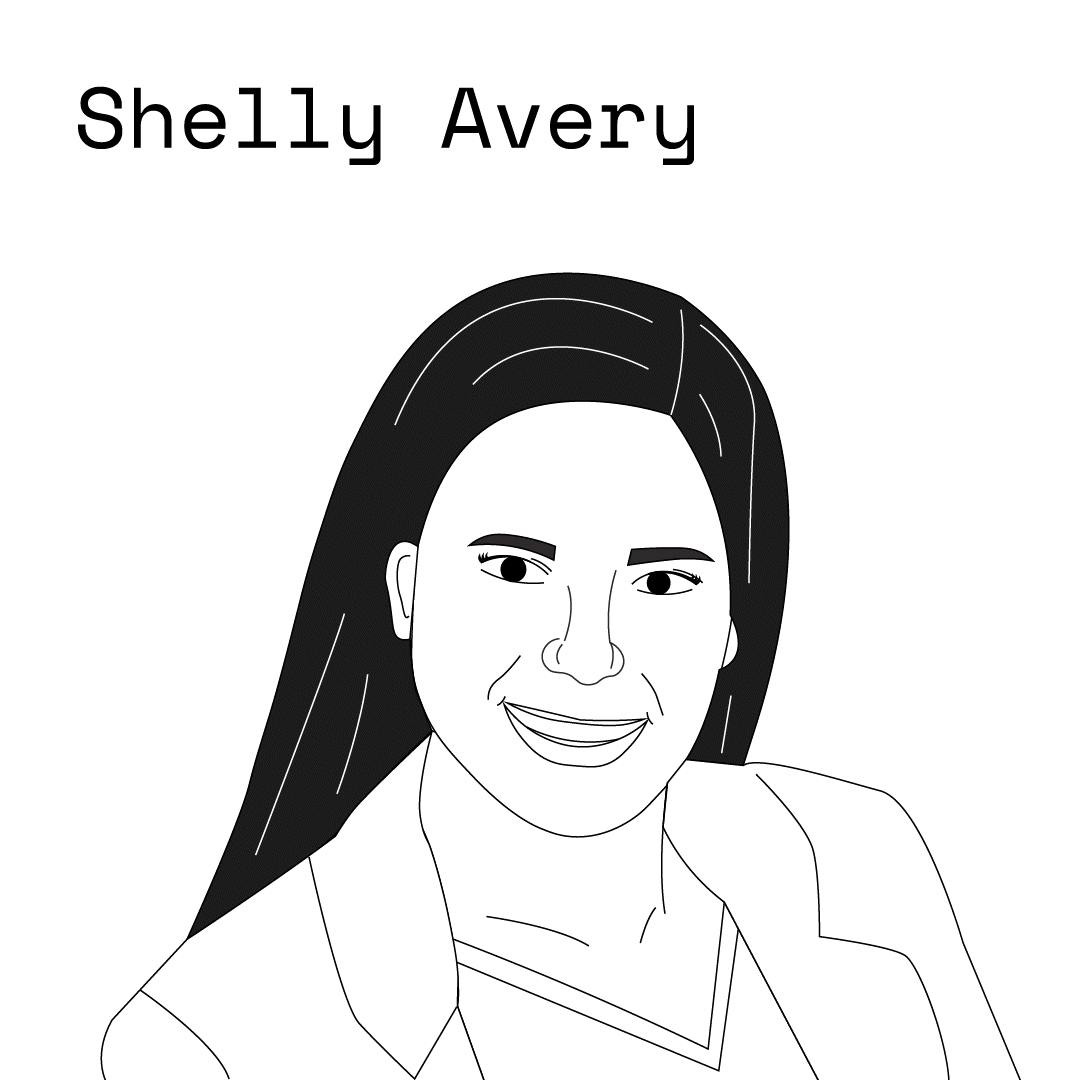
99% is the New Zero, w/ Microsoft Director Shelly Avery
Director-US Healthcare Specialist, Microsoft
09.14.21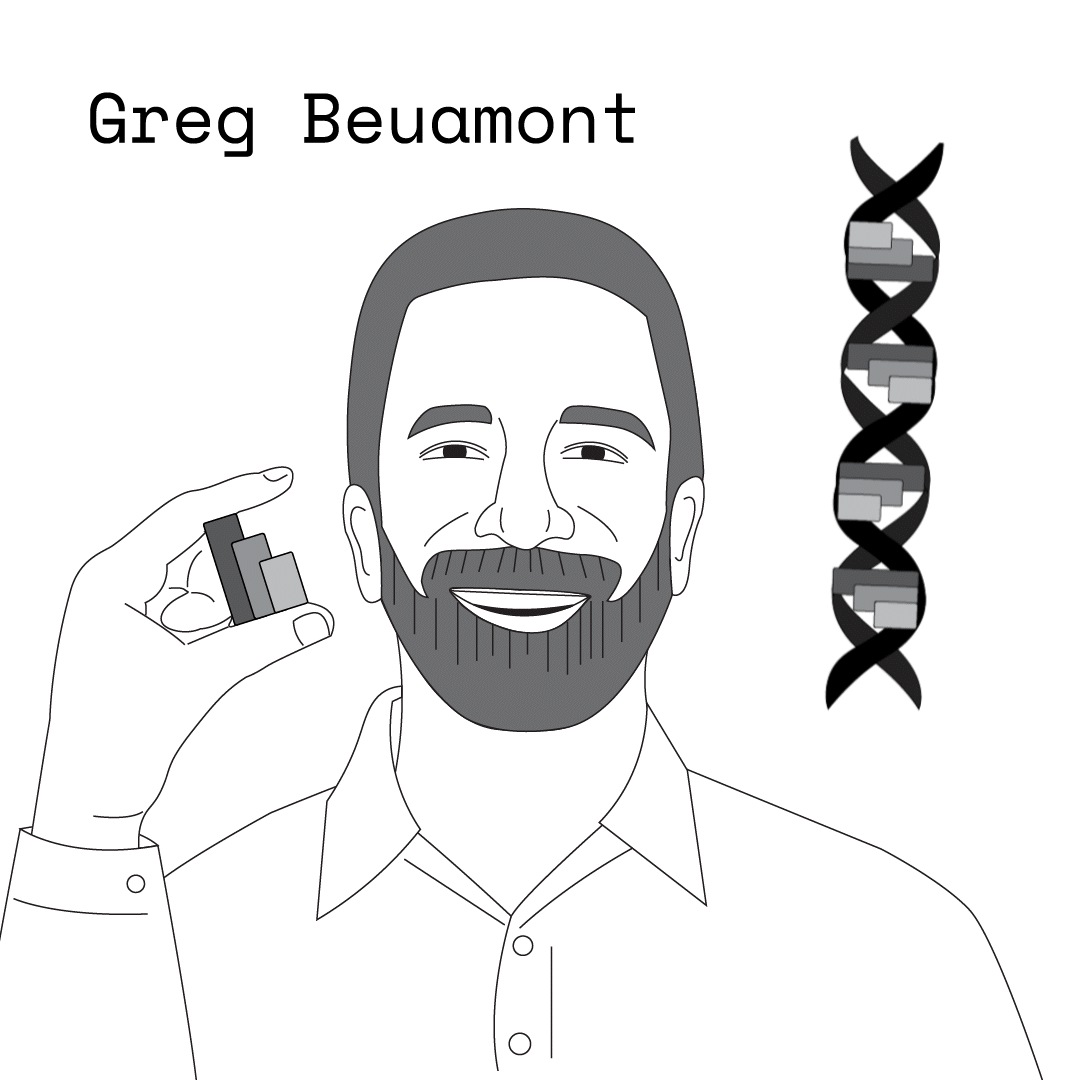
For Those About to DAX, w/ Microsoft’s Greg Beaumont
Senior Business Intelligence Specialist, Microsoft
08.17.21
Spreadsheet Celebrities & Power BI Playdates, w/ Chandoo
Hear from the Mozart of Excel Dashboards: Chandoo
08.10.21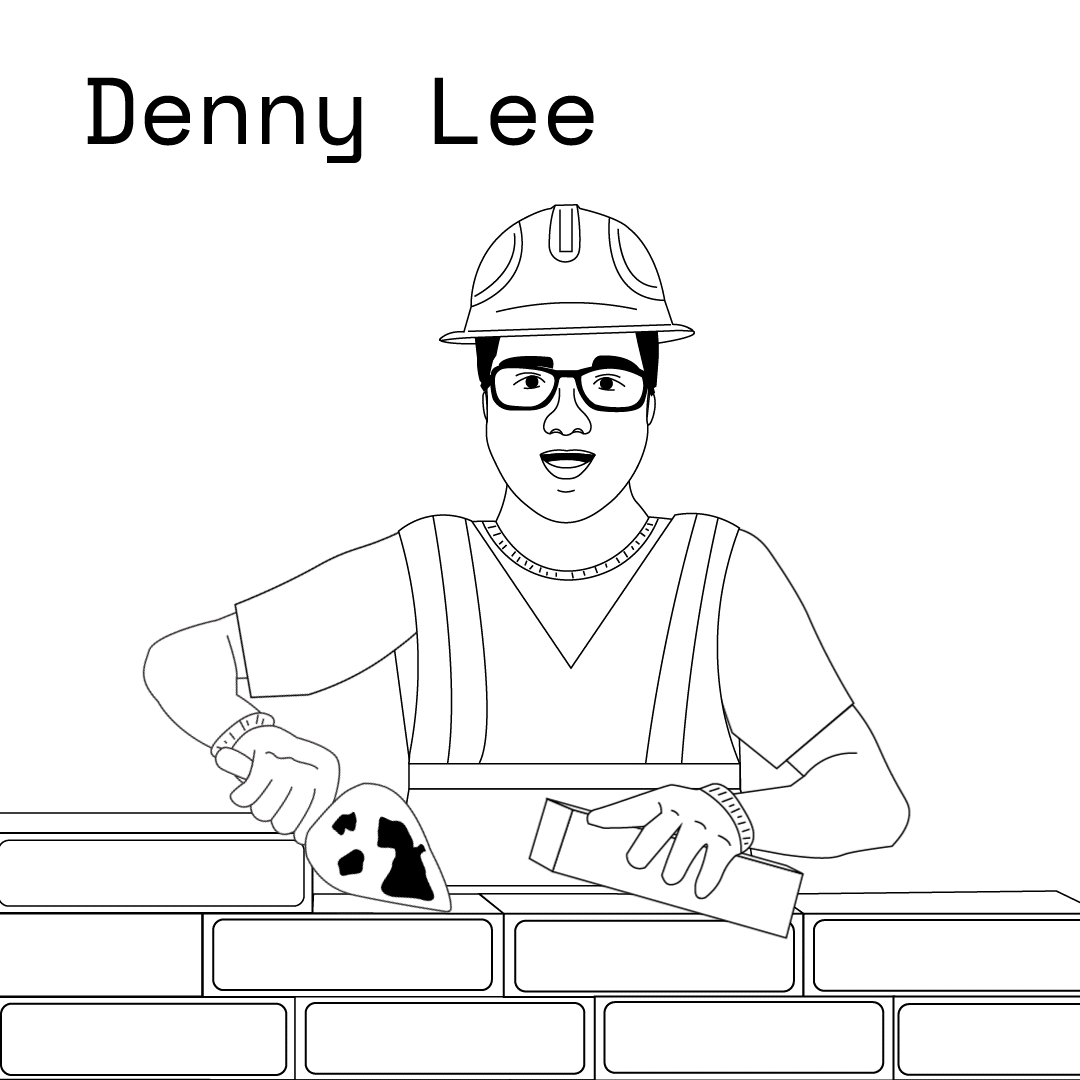
The Data Lakehouse: Brick by Brick, w/ Databricks’ Denny Lee
Sr. Staff Developer Advocate, Databricks
08.03.21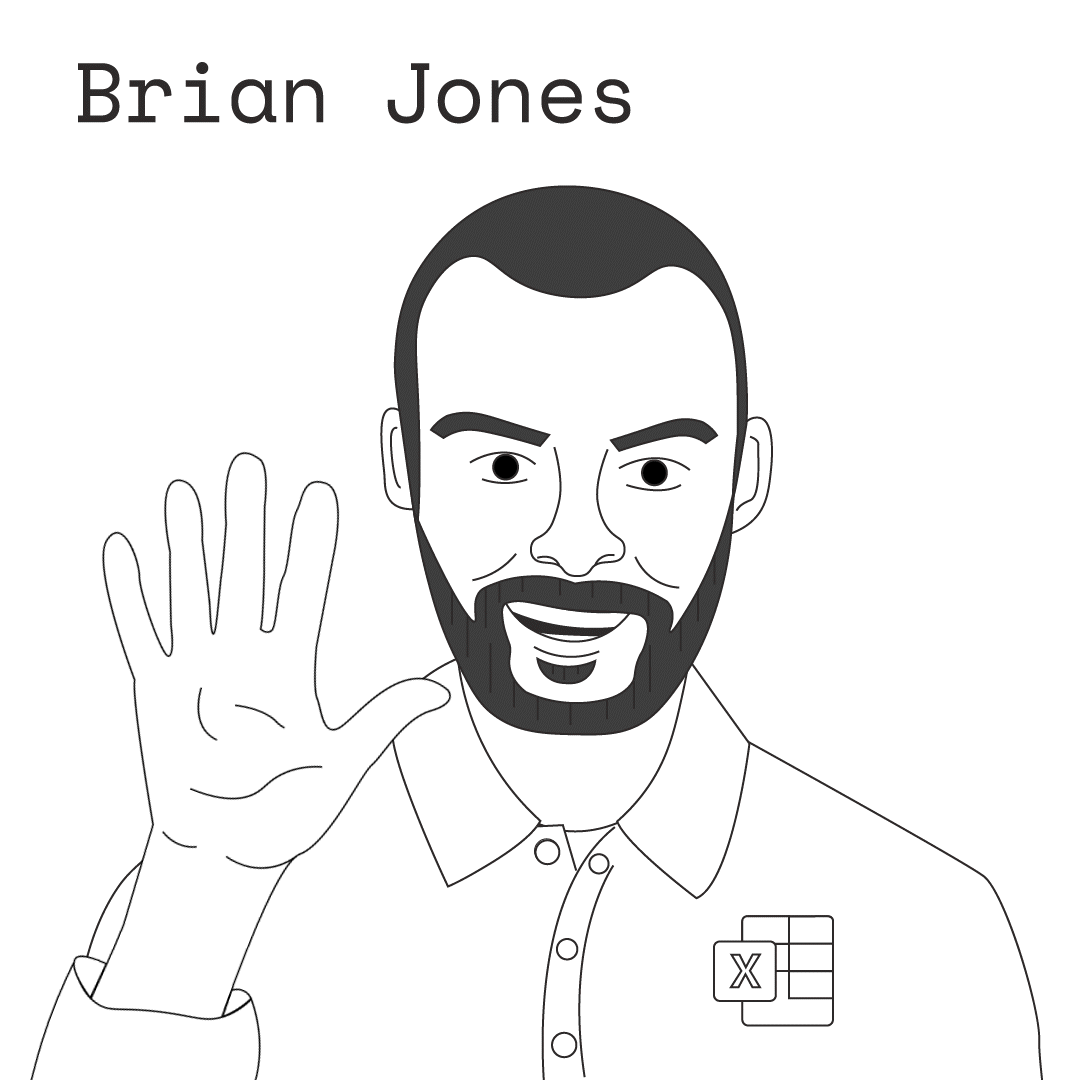
Excel Past/Present/Future, w/ Excel Head of Product Brian Jones
Head of Product, Excel-Microsoft
07.27.21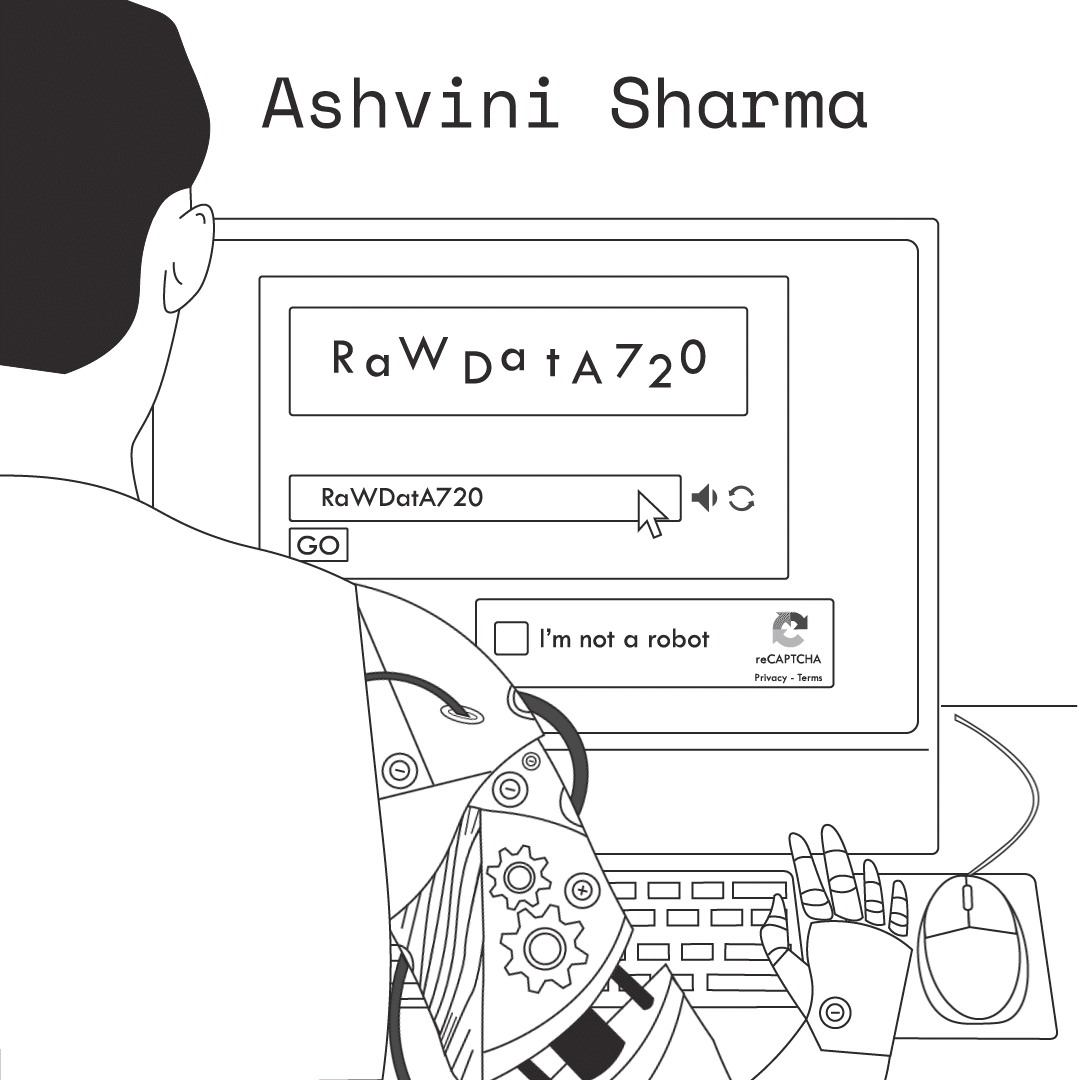
Robotic Process Automation for All, w/ Microsoft’s Ashvini Sharma
Group Program Manager-Power Automate RPA, Microsoft
07.20.21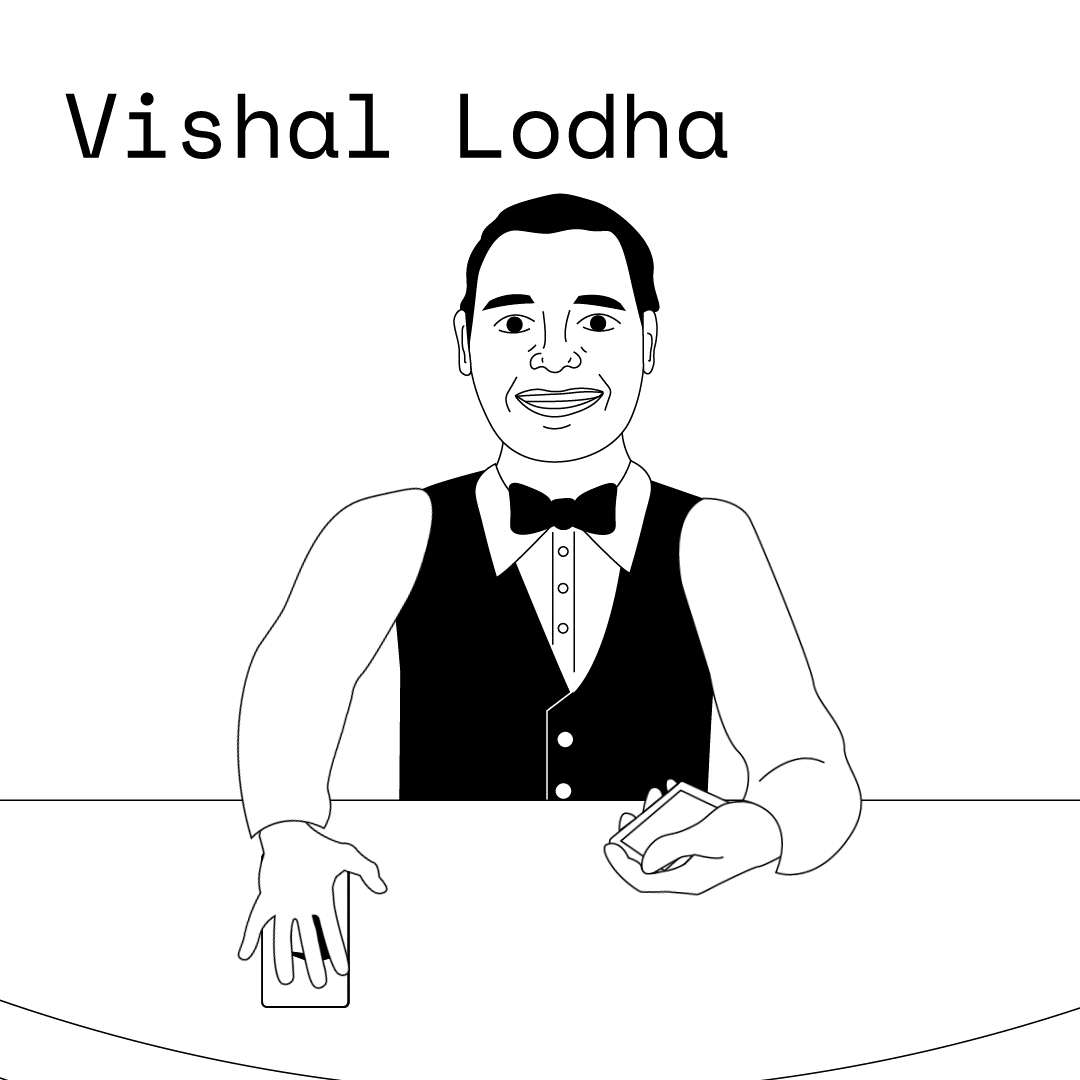
COVID Basement Racquetball, w/ Microsoft’s Vishal Lodha
Strategic Account Director, Microsoft
06.03.21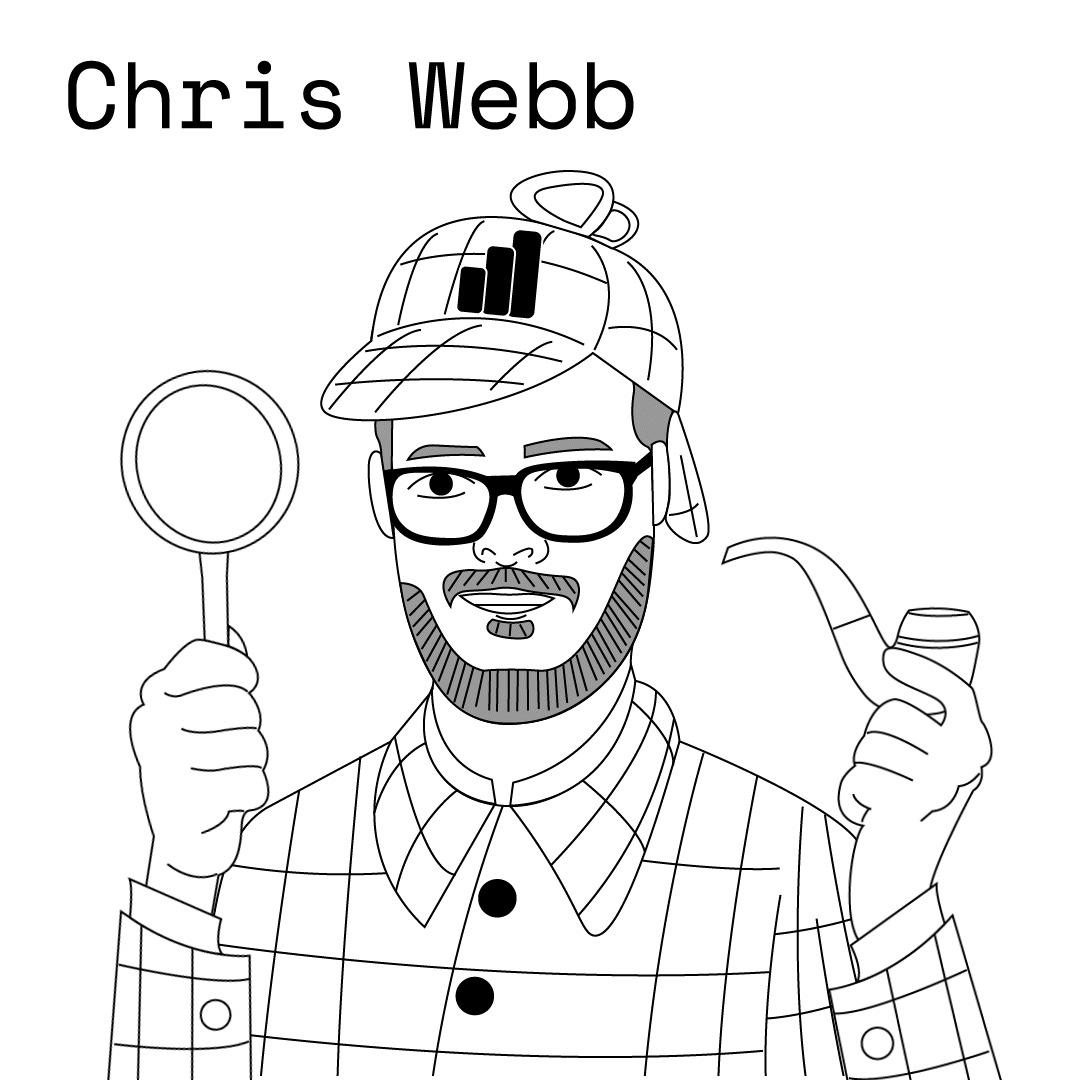
Surfing the Changing Seas of Data, w/ Microsoft’s Chris Webb
Principal Program Manager-Power BI Customer Advisory Team, Microsoft
06.01.21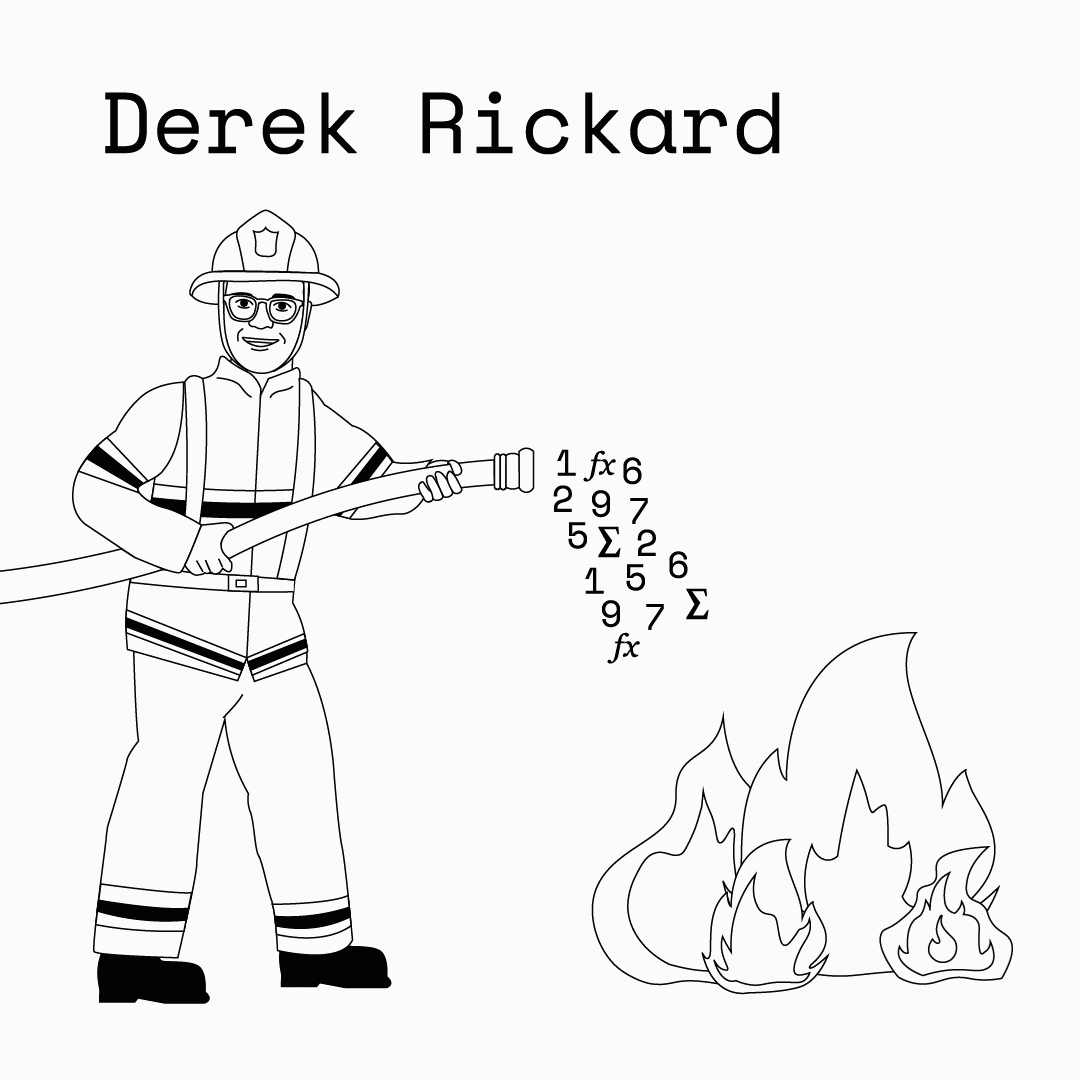
A Different Kind of Data Emergency, w/ Derek Rickard
Chief Data Officer, Emergency Reporting
05.04.21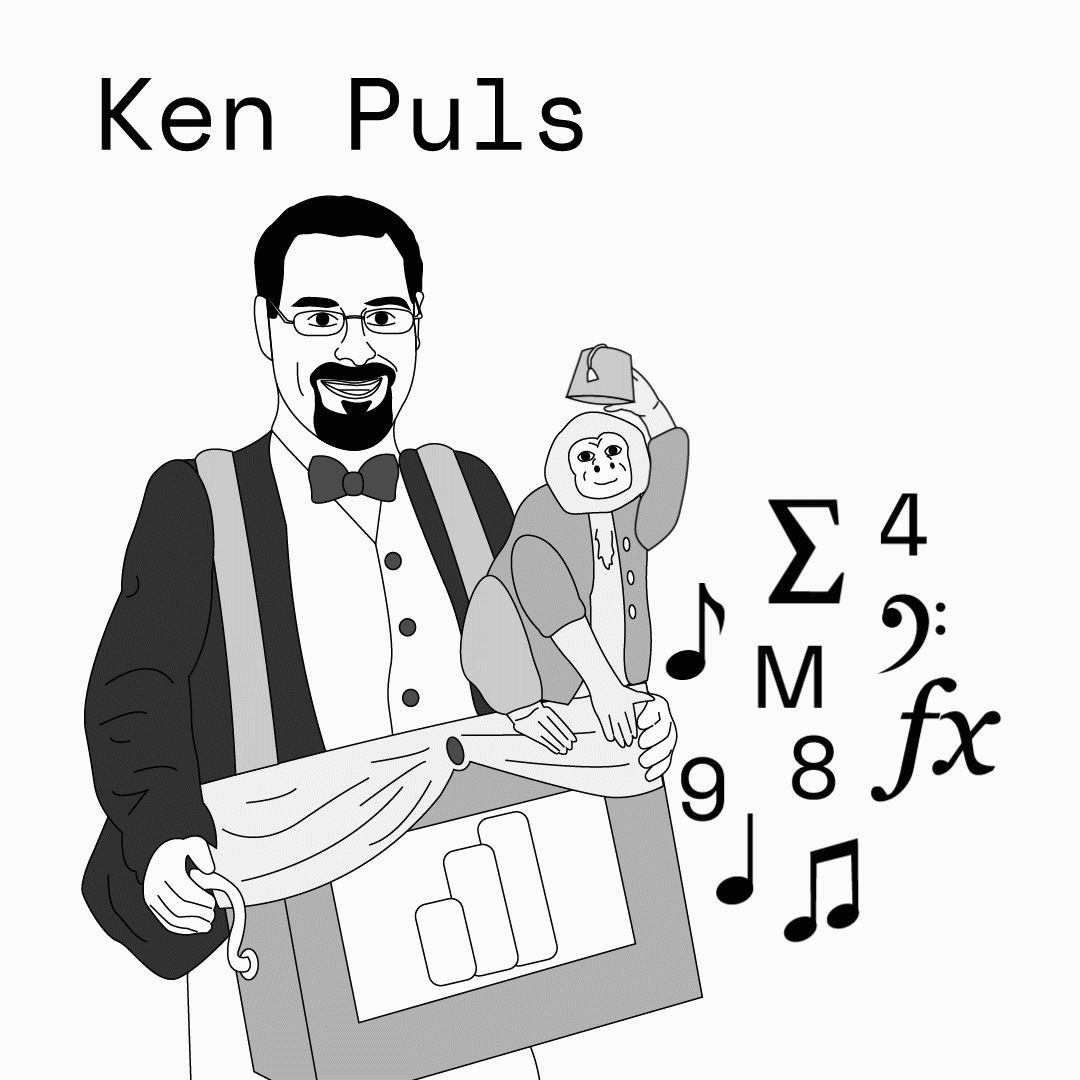
Earth’s Only Hope vs. Alien Excel Invaders, w/ Ken Puls
President and Chief Training Officer, Excelguru Consulting Inc.
04.27.21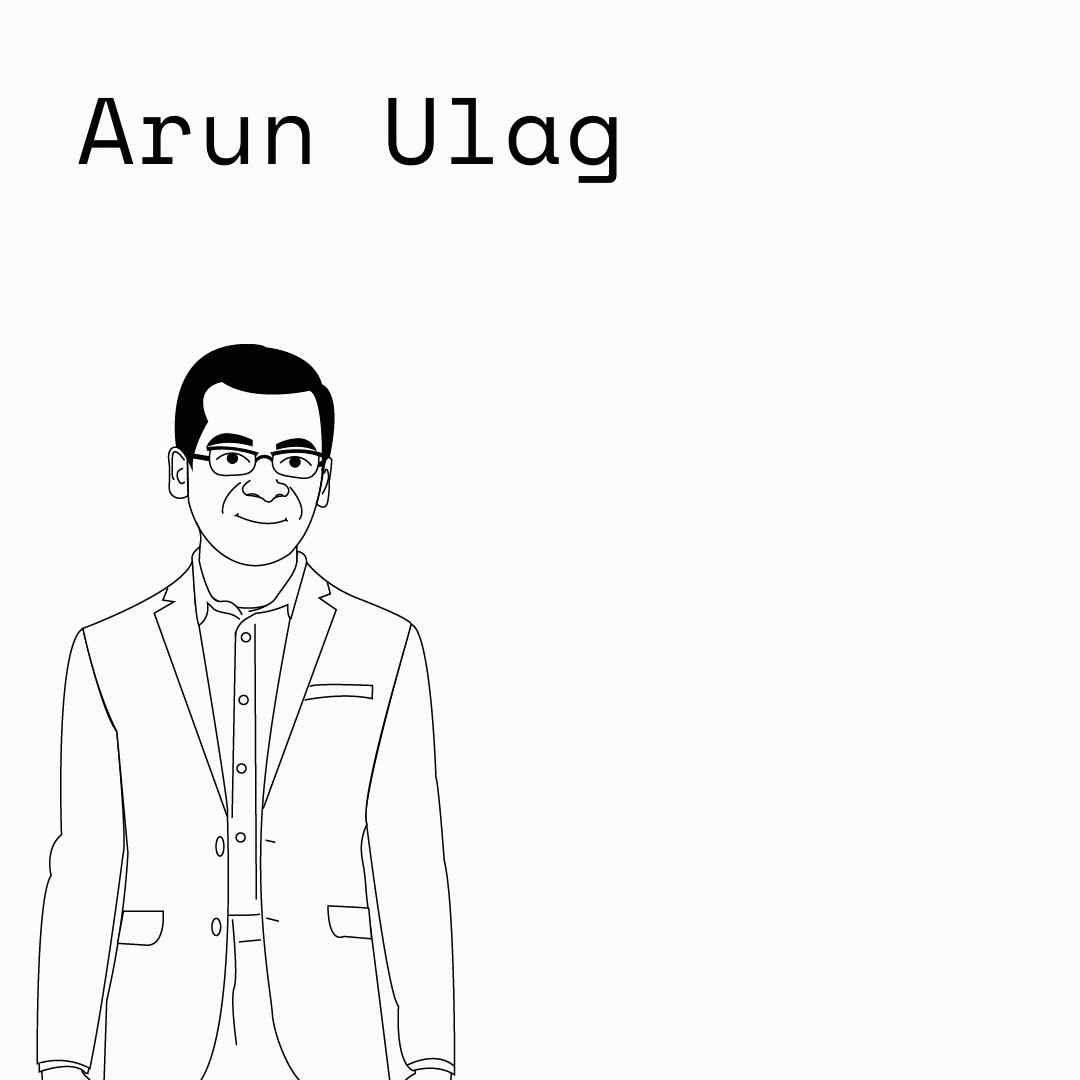
Intelligence Platform is a Cool Name, w/ Microsoft CVP Arun Ulag
Corporate Vice President of the Business Intelligence Platform at Microsoft
04.01.21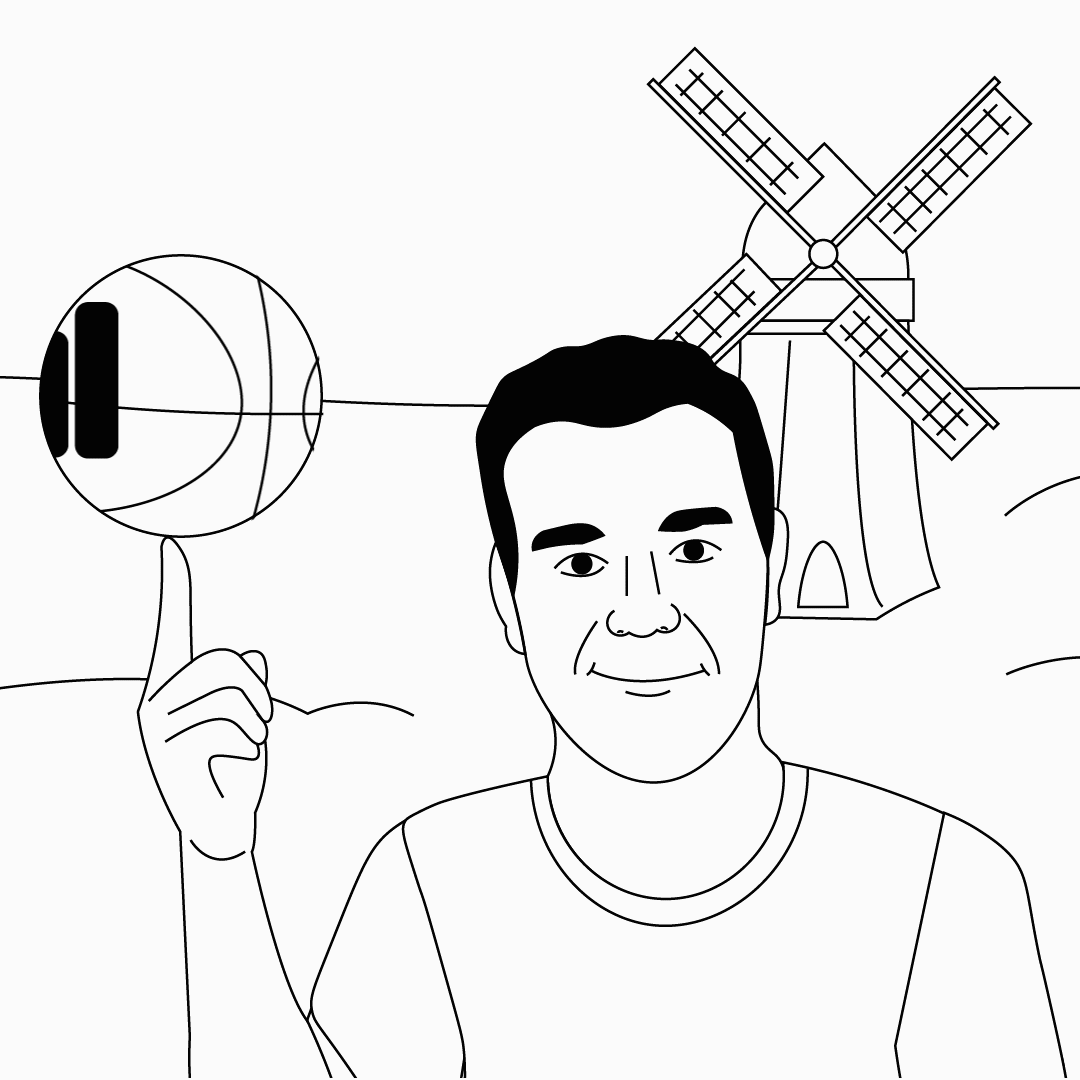
The Dutchman of Data Dunked on Me, w/ Kasper de Jonge
Analytics Advisor and Principal Program Manager-Power BI, Microsoft
03.23.21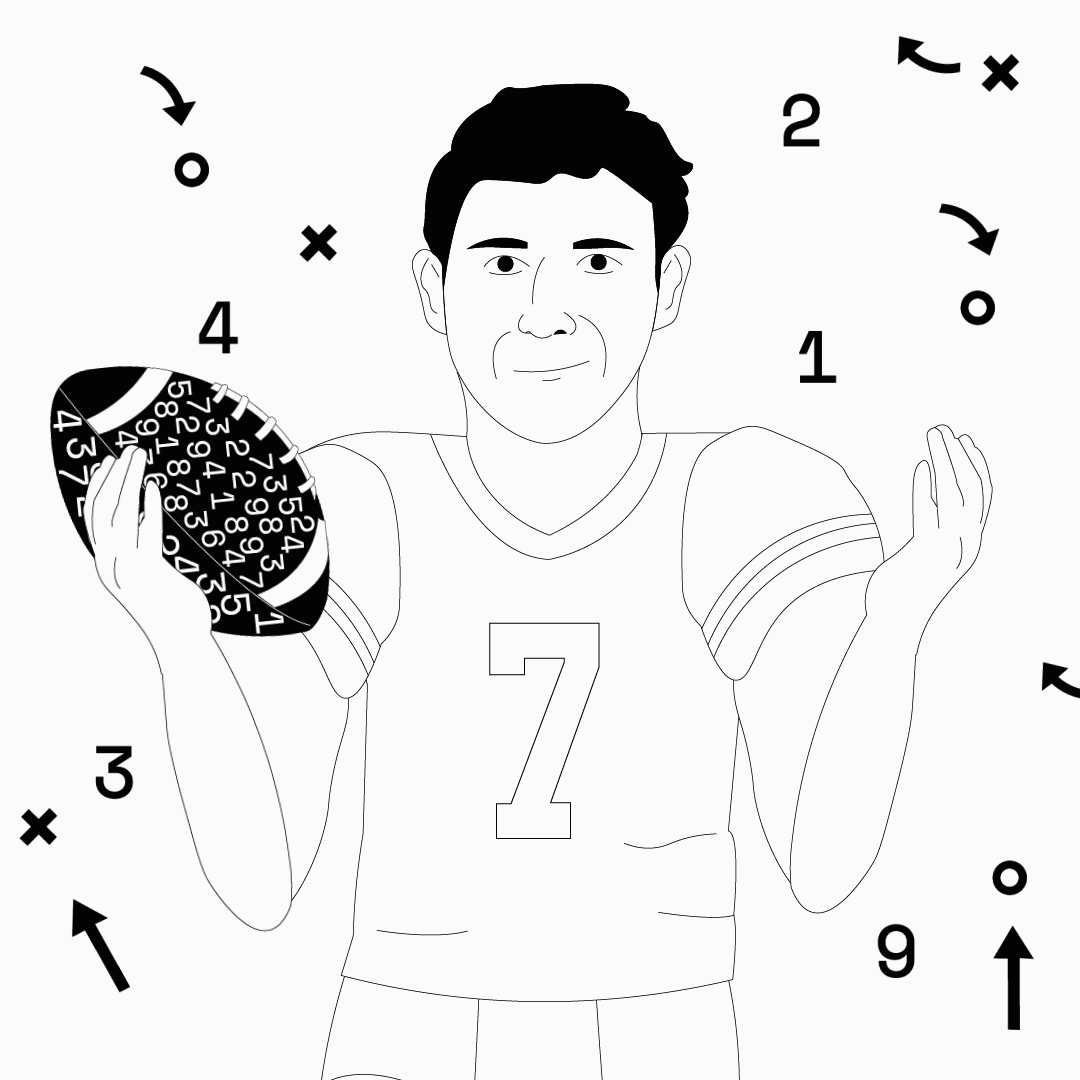
Everyone’s in the Middle, w/ Former NFL QB Hugh Millen
Former NFL Quarterback and Data Nerd
03.09.21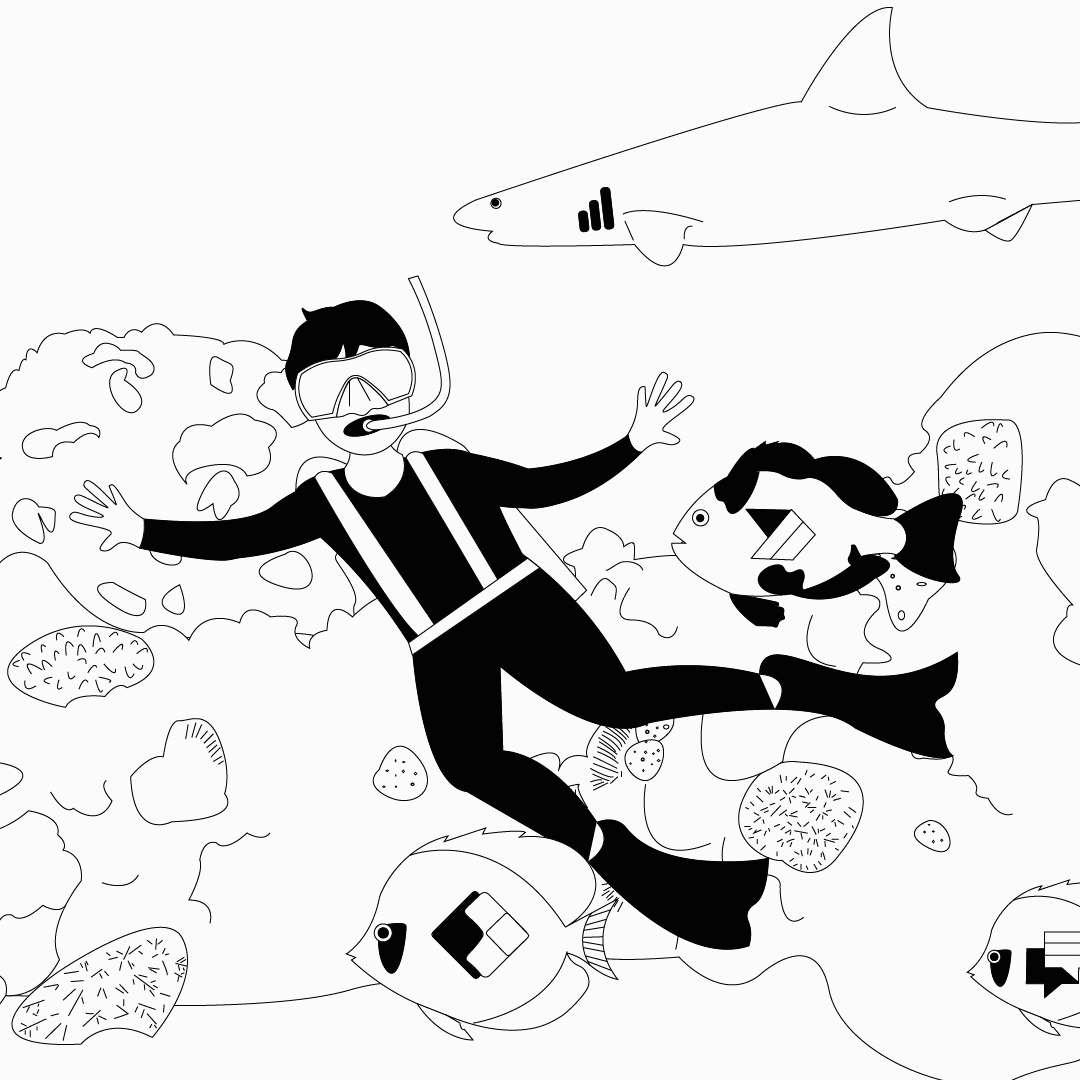
Marine Biology Leads to Power Platforming, w/ Chuck Sterling
Senior Program Manager, Microsoft
02.23.21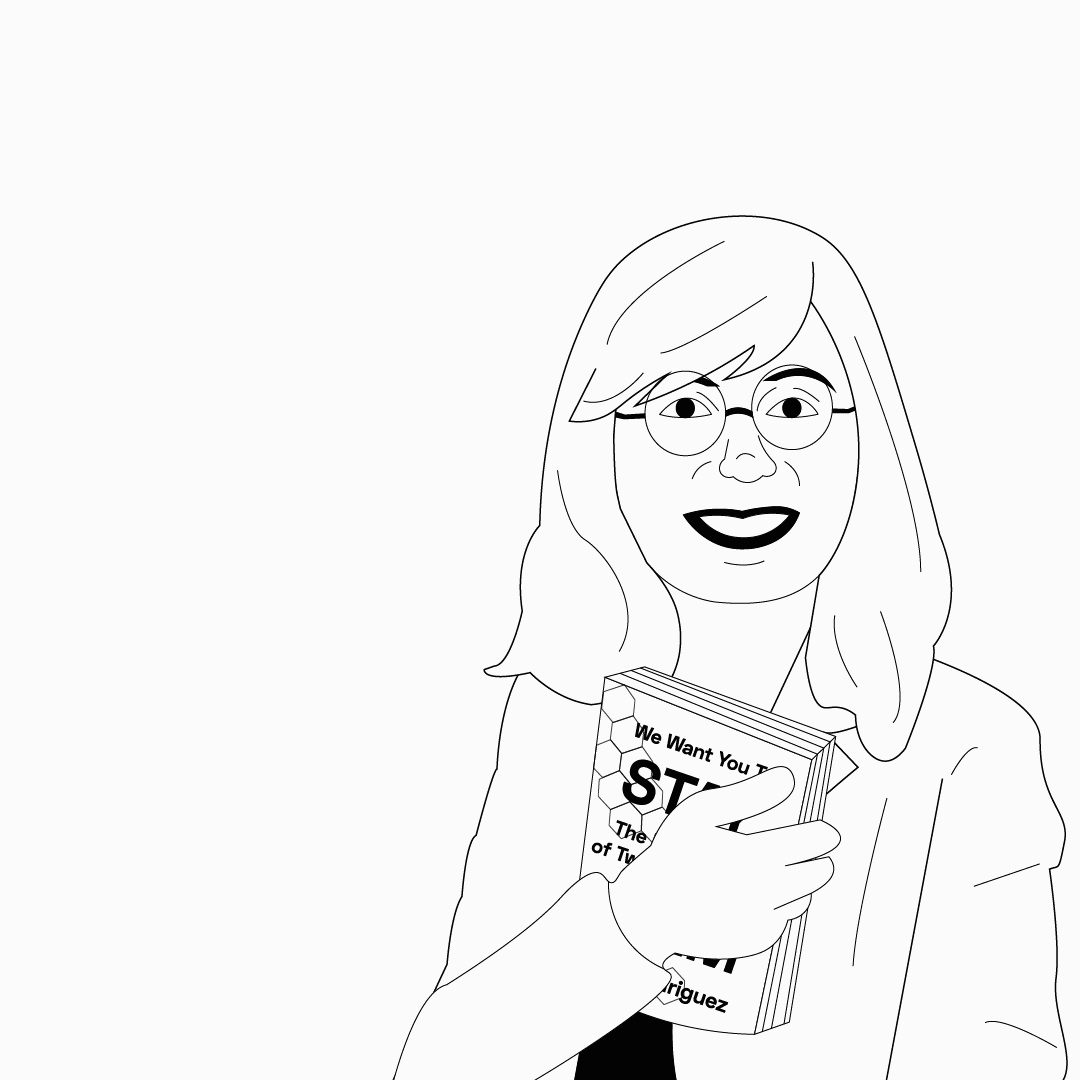
Data Chefs Can Come From Anywhere, w/ Gartner’s Lori Rodriguez
Vice President Executive Programs at Gartner
01.26.21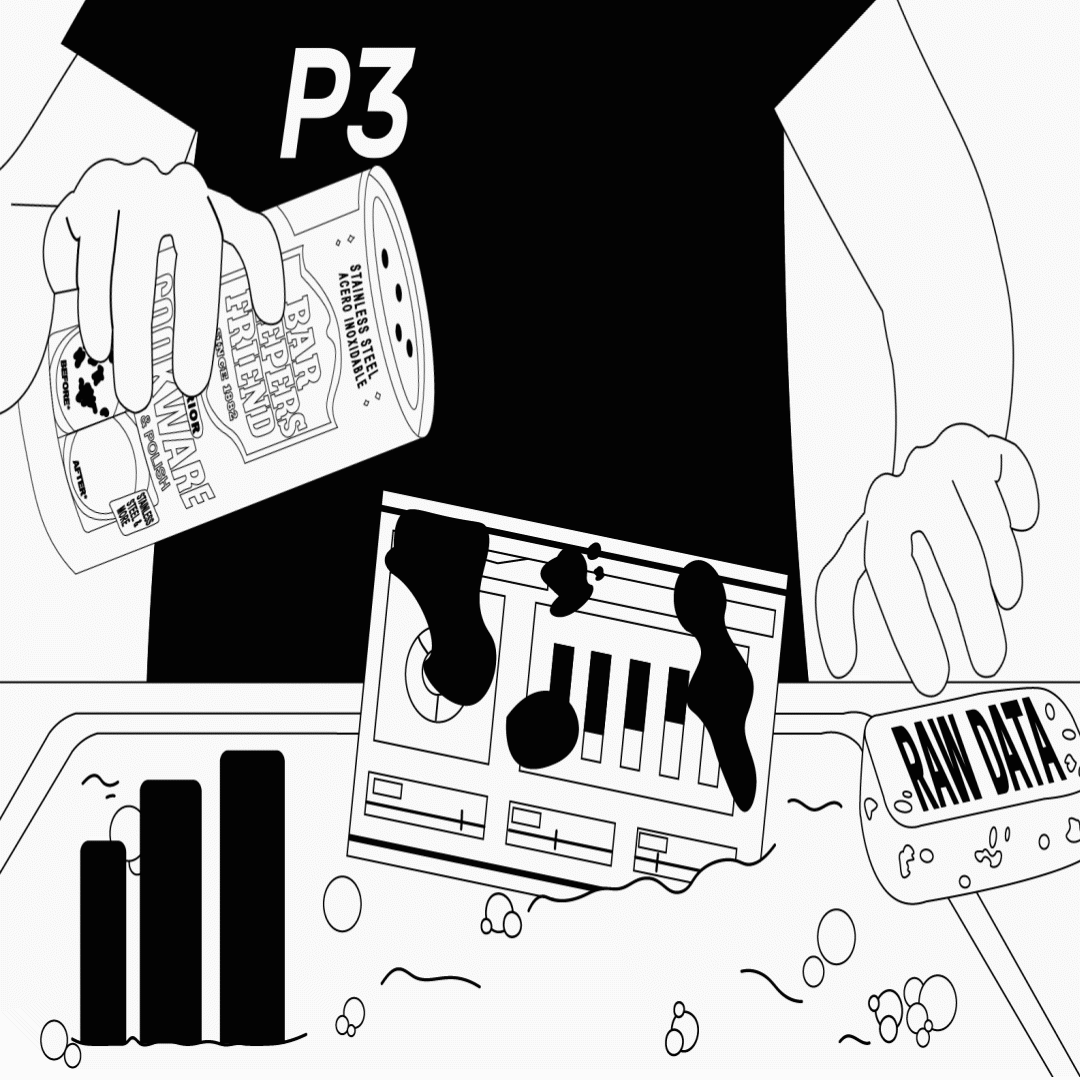
Scrubbing Data & Beating COVID, w/ Matt Selig of Bar Keepers Friend
Exec. Vice President, Chief Financial Officer & Chief Human Resources Officer at SerVaas Labs
01.05.21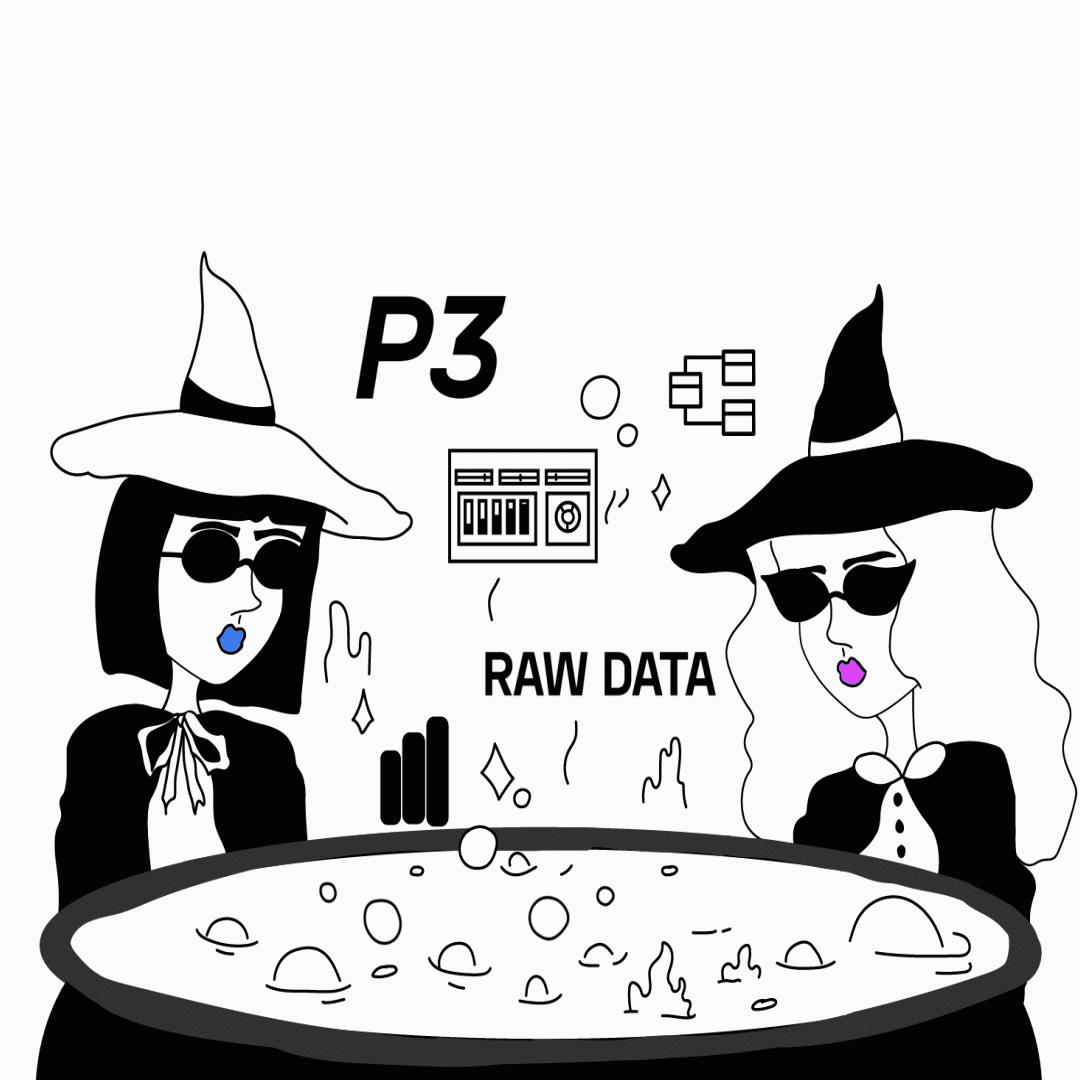
The Data Witches, w/ Shannon Lindsay & Stephanie Bruno
Shannon Lindsay-Microsoft Certified Data and Analytics Professional &
Stephanie Bruno-Associate Director of Informatics at the Elizabeth Glaser Pediatric AIDS Foundation (EGPAF)
12.22.20
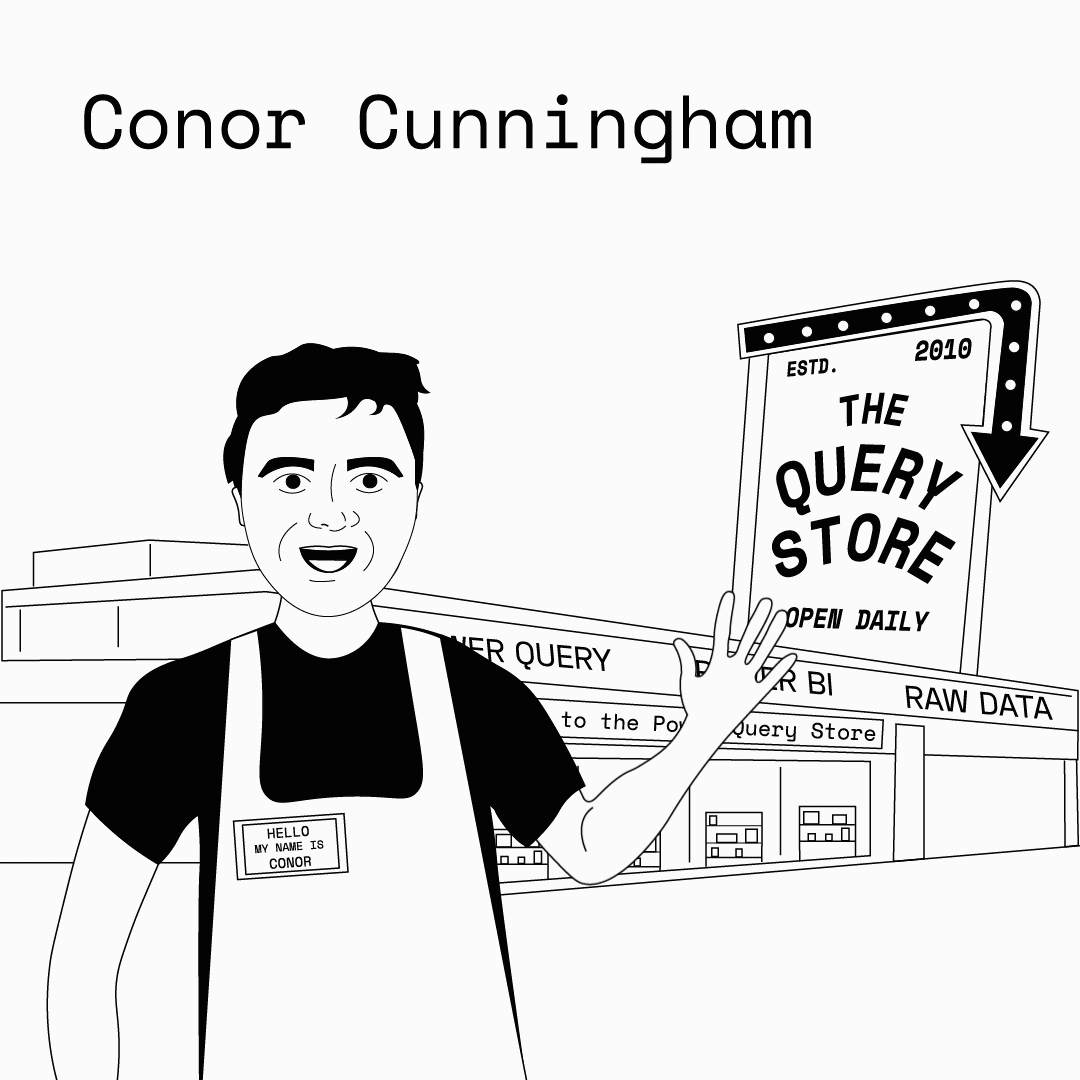
The Query Store’s Open for Biz, w/ Microsoft’s Conor Cunningham
Partner Software Architect, SQL Server Engine-Microsoft
12.15.20One of the World’s Most Amazing Humans, w/ Matt Allington
Power BI Consultant, Trainer, & Microsoft MVP
12.01.20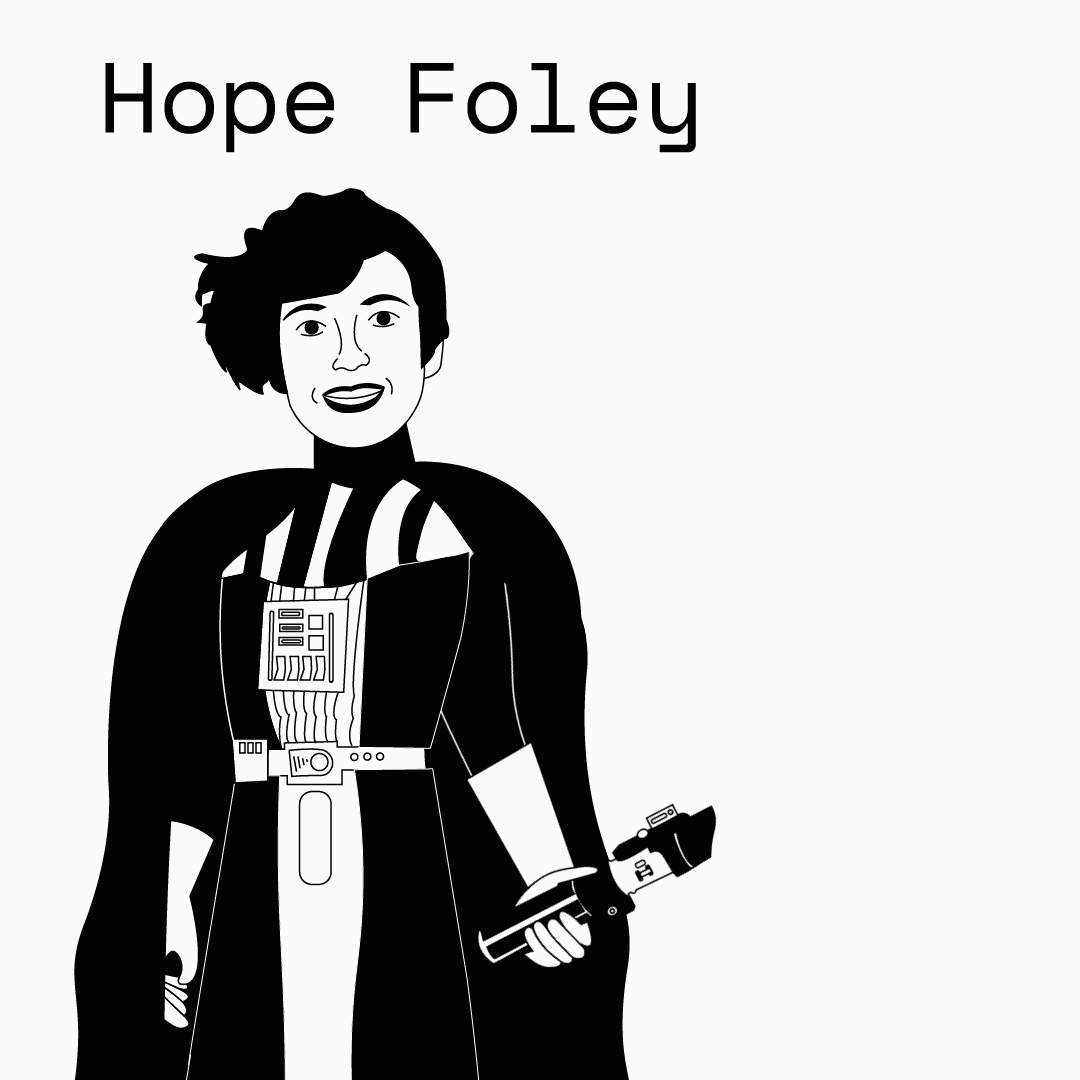